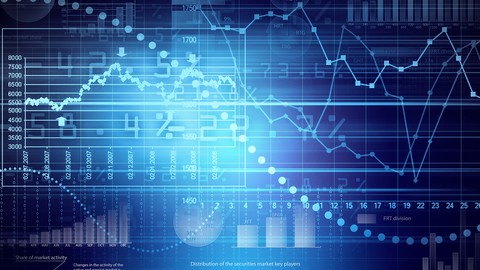
Unsupervised Machine Learning Hidden Markov Models in Python
Unsupervised Machine Learning Hidden Markov Models in Python, available at $24.99, has an average rating of 4.64, with 74 lectures, based on 4260 reviews, and has 29521 subscribers.
You will learn about Understand and enumerate the various applications of Markov Models and Hidden Markov Models Understand how Markov Models work Write a Markov Model in code Apply Markov Models to any sequence of data Understand the mathematics behind Markov chains Apply Markov models to language Apply Markov models to website analytics Understand how Google's PageRank works Understand Hidden Markov Models Write a Hidden Markov Model in Code Write a Hidden Markov Model using Theano Understand how gradient descent, which is normally used in deep learning, can be used for HMMs This course is ideal for individuals who are Students and professionals who do data analysis, especially on sequence data or Professionals who want to optimize their website experience or Students who want to strengthen their machine learning knowledge and practical skillset or Students and professionals interested in DNA analysis and gene expression or Students and professionals interested in modeling language and generating text from a model It is particularly useful for Students and professionals who do data analysis, especially on sequence data or Professionals who want to optimize their website experience or Students who want to strengthen their machine learning knowledge and practical skillset or Students and professionals interested in DNA analysis and gene expression or Students and professionals interested in modeling language and generating text from a model.
Enroll now: Unsupervised Machine Learning Hidden Markov Models in Python
Summary
Title: Unsupervised Machine Learning Hidden Markov Models in Python
Price: $24.99
Average Rating: 4.64
Number of Lectures: 74
Number of Published Lectures: 65
Number of Curriculum Items: 74
Number of Published Curriculum Objects: 65
Original Price: $24.99
Quality Status: approved
Status: Live
What You Will Learn
- Understand and enumerate the various applications of Markov Models and Hidden Markov Models
- Understand how Markov Models work
- Write a Markov Model in code
- Apply Markov Models to any sequence of data
- Understand the mathematics behind Markov chains
- Apply Markov models to language
- Apply Markov models to website analytics
- Understand how Google's PageRank works
- Understand Hidden Markov Models
- Write a Hidden Markov Model in Code
- Write a Hidden Markov Model using Theano
- Understand how gradient descent, which is normally used in deep learning, can be used for HMMs
Who Should Attend
- Students and professionals who do data analysis, especially on sequence data
- Professionals who want to optimize their website experience
- Students who want to strengthen their machine learning knowledge and practical skillset
- Students and professionals interested in DNA analysis and gene expression
- Students and professionals interested in modeling language and generating text from a model
Target Audiences
- Students and professionals who do data analysis, especially on sequence data
- Professionals who want to optimize their website experience
- Students who want to strengthen their machine learning knowledge and practical skillset
- Students and professionals interested in DNA analysis and gene expression
- Students and professionals interested in modeling language and generating text from a model
The Hidden Markov Modelor HMM is all about learning sequences.
A lot of the data that would be very useful for us to model is in sequences. Stock prices are sequences of prices. Language is a sequence of words. Credit scoring involves sequences of borrowing and repaying money, and we can use those sequences to predict whether or not you’re going to default. In short, sequences are everywhere, and being able to analyze them is an important skill in your data science toolbox.
The easiest way to appreciate the kind of information you get from a sequence is to consider what you are reading right now. If I had written the previous sentence backwards, it wouldn’t make much sense to you, even though it contained all the same words. So order is important.
While the current fad in deep learningis to use recurrent neural networks to model sequences, I want to first introduce you guys to a machine learning algorithm that has been around for several decades now – the Hidden Markov Model.
This course follows directly from my first course in Unsupervised Machine Learning for Cluster Analysis, where you learned how to measure the probability distribution of a random variable. In this course, you’ll learn to measure the probability distribution of a sequence of random variables.
You guys know how much I love deep learning, so there is a little twist in this course. We’ve already covered gradient descent and you know how central it is for solving deep learning problems. I claimed that gradient descent could be used to optimize any objective function. In this course I will show you how you can use gradient descent to solve for the optimal parameters of an HMM, as an alternative to the popular expectation-maximization algorithm.
We’re going to do it in Theano and Tensorflow, which are popular libraries for deep learning. This is also going to teach you how to work with sequences in Theano and Tensorflow, which will be very useful when we cover recurrent neural networks and LSTMs.
This course is also going to go through the many practical applications of Markov models and hidden Markov models. We’re going to look at a model of sickness and health, and calculate how to predict how long you’ll stay sick, if you get sick. We’re going to talk about how Markov models can be used to analyze how people interact with your website, and fix problem areas like high bounce rate, which could be affecting your SEO. We’ll build language models that can be used to identify a writer and even generate text – imagine a machine doing your writing for you. HMMs have been very successful in natural language processing or NLP.
We’ll look at what is possibly the most recent and prolific application of Markov models – Google’s PageRank algorithm. And finally we’ll discuss even more practical applications of Markov models, including generating images, smartphone autosuggestions, and using HMMs to answer one of the most fundamental questions in biology – how is DNA, the code of life, translated into physical or behavioral attributes of an organism?
All of the materials of this course can be downloaded and installed for FREE. We will do most of our work in Numpy and Matplotlib, along with a little bit of Theano. I am always available to answer your questions and help you along your data science journey.
This course focuses on “how to build and understand“, not just “how to use”. Anyone can learn to use an API in 15 minutes after reading some documentation. It’s not about “remembering facts”, it’s about “seeing for yourself” via experimentation. It will teach you how to visualize what’s happening in the model internally. If you want more than just a superficial look at machine learning models, this course is for you.
See you in class!
“If you can’t implement it, you don’t understand it”
-
Or as the great physicist Richard Feynman said: “What I cannot create, I do not understand”.
-
My courses are the ONLY courses where you will learn how to implement machine learning algorithms from scratch
-
Other courses will teach you how to plug in your data into a library, but do you really need help with 3 lines of code?
-
After doing the same thing with 10 datasets, you realize you didn’t learn 10 things. You learned 1 thing, and just repeated the same 3 lines of code 10 times…
Suggested Prerequisites:
-
calculus
-
linear algebra
-
probability
-
Be comfortable with the multivariate Gaussian distribution
-
Python coding: if/else, loops, lists, dicts, sets
-
Numpy coding: matrix and vector operations, loading a CSV file
WHAT ORDER SHOULD I TAKE YOUR COURSES IN?:
-
Check out the lecture “Machine Learning and AI Prerequisite Roadmap” (available in the FAQ of any of my courses, including the free Numpy course)
Course Curriculum
Chapter 1: Introduction and Outline
Lecture 1: Introduction and Outline: Why would you want to use an HMM?
Lecture 2: Where to get the Code and Data
Lecture 3: How to Succeed in this Course
Chapter 2: Markov Models
Lecture 1: The Markov Property
Lecture 2: Markov Models
Lecture 3: Probability Smoothing and Log-Space
Lecture 4: The Math of Markov Chains
Chapter 3: Markov Models: Example Problems and Applications
Lecture 1: Example Problem: Sick or Healthy
Lecture 2: Example Problem: Expected number of continuously sick days
Lecture 3: Example application: SEO and Bounce Rate Optimization
Lecture 4: Example Application: Build a 2nd-order language model and generate phrases
Lecture 5: Example Application: Google’s PageRank algorithm
Lecture 6: Suggestion Box
Chapter 4: Hidden Markov Models for Discrete Observations
Lecture 1: From Markov Models to Hidden Markov Models
Lecture 2: HMM – Basic Examples
Lecture 3: Parameters of an HMM
Lecture 4: The 3 Problems of an HMM
Lecture 5: The Forward-Backward Algorithm (part 1)
Lecture 6: The Forward-Backward Algorithm (part 2)
Lecture 7: The Forward-Backward Algorithm (part 3)
Lecture 8: The Viterbi Algorithm (part 1)
Lecture 9: The Viterbi Algorithm (part 2)
Lecture 10: HMM Training (part 1)
Lecture 11: HMM Training (part 2)
Lecture 12: HMM Training (part 3)
Lecture 13: HMM Training (part 4)
Lecture 14: How to Choose the Number of Hidden States
Lecture 15: Baum-Welch Updates for Multiple Observations
Lecture 16: Discrete HMM in Code
Lecture 17: The underflow problem and how to solve it
Lecture 18: Discrete HMM Updates in Code with Scaling
Lecture 19: Scaled Viterbi Algorithm in Log Space
Chapter 5: Discrete HMMs Using Deep Learning Libraries
Lecture 1: Gradient Descent Tutorial
Lecture 2: Theano Scan Tutorial
Lecture 3: Discrete HMM in Theano
Lecture 4: Improving our Gradient Descent-Based HMM
Lecture 5: Tensorflow Scan Tutorial
Lecture 6: Discrete HMM in Tensorflow
Chapter 6: HMMs for Continuous Observations
Lecture 1: Gaussian Mixture Models with Hidden Markov Models
Lecture 2: Generating Data from a Real-Valued HMM
Lecture 3: Continuous-Observation HMM in Code (part 1)
Lecture 4: Continuous-Observation HMM in Code (part 2)
Lecture 5: Continuous HMM in Theano
Lecture 6: Continuous HMM in Tensorflow
Chapter 7: HMMs for Classification
Lecture 1: Unsupervised or Supervised?
Lecture 2: Generative vs. Discriminative Classifiers
Lecture 3: HMM Classification on Poetry Data (Robert Frost vs. Edgar Allan Poe)
Chapter 8: Bonus Example: Parts-of-Speech Tagging
Lecture 1: Parts-of-Speech Tagging Concepts
Lecture 2: POS Tagging with an HMM
Chapter 9: Theano, Tensorflow, and Machine Learning Basics Review
Lecture 1: (Review) Gaussian Mixture Models
Lecture 2: (Review) Theano Tutorial
Lecture 3: (Review) Tensorflow Tutorial
Chapter 10: Setting Up Your Environment (FAQ by Student Request)
Lecture 1: Pre-Installation Check
Lecture 2: Anaconda Environment Setup
Lecture 3: How to install Numpy, Scipy, Matplotlib, Pandas, IPython, Theano, and TensorFlow
Chapter 11: Extra Help With Python Coding for Beginners (FAQ by Student Request)
Lecture 1: How to Code by Yourself (part 1)
Lecture 2: How to Code by Yourself (part 2)
Lecture 3: Proof that using Jupyter Notebook is the same as not using it
Lecture 4: Python 2 vs Python 3
Chapter 12: Effective Learning Strategies for Machine Learning (FAQ by Student Request)
Lecture 1: How to Succeed in this Course (Long Version)
Lecture 2: Is this for Beginners or Experts? Academic or Practical? Fast or slow-paced?
Lecture 3: Machine Learning and AI Prerequisite Roadmap (pt 1)
Lecture 4: Machine Learning and AI Prerequisite Roadmap (pt 2)
Chapter 13: Appendix / FAQ Finale
Lecture 1: What is the Appendix?
Lecture 2: BONUS
Instructors
-
Lazy Programmer Team
Artificial Intelligence and Machine Learning Engineer -
Lazy Programmer Inc.
Artificial intelligence and machine learning engineer
Rating Distribution
- 1 stars: 38 votes
- 2 stars: 42 votes
- 3 stars: 193 votes
- 4 stars: 1605 votes
- 5 stars: 2382 votes
Frequently Asked Questions
How long do I have access to the course materials?
You can view and review the lecture materials indefinitely, like an on-demand channel.
Can I take my courses with me wherever I go?
Definitely! If you have an internet connection, courses on Udemy are available on any device at any time. If you don’t have an internet connection, some instructors also let their students download course lectures. That’s up to the instructor though, so make sure you get on their good side!
You may also like
- Digital Marketing Foundation Course
- Google Shopping Ads Digital Marketing Course
- Multi Cloud Infrastructure for beginners
- Master Lead Generation: Grow Subscribers & Sales with Popups
- Complete Copywriting System : write to sell with ease
- Product Positioning Masterclass: Unlock Market Traction
- How to Promote Your Webinar and Get More Attendees?
- Digital Marketing Courses
- Create music with Artificial Intelligence in this new market
- Create CONVERTING UGC Content So Brands Will Pay You More
- Podcast: The top 8 ways to monetize by Podcasting
- TikTok Marketing Mastery: Learn to Grow & Go Viral
- Free Digital Marketing Basics Course in Hindi
- MailChimp Free Mailing Lists: MailChimp Email Marketing
- Automate Digital Marketing & Social Media with Generative AI
- Google Ads MasterClass – All Advanced Features
- Online Course Creator: Create & Sell Online Courses Today!
- Introduction to SEO – Basic Principles of SEO
- Affiliate Marketing For Beginners: Go From Novice To Pro
- Effective Website Planning Made Simple