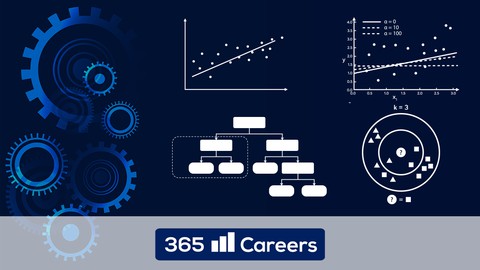
The Supervised Machine Learning Bootcamp
The Supervised Machine Learning Bootcamp, available at $74.99, has an average rating of 4.59, with 83 lectures, 12 quizzes, based on 1124 reviews, and has 4788 subscribers.
You will learn about Regression and Classification Algorithms Using sk-learn and Python to implement supervised machine learning techniques K-nearest neighbors for both classification and regression Naïve Bayes Ridge and Lasso Regression Decision Trees Random Forests Support Vector Machines Practical case studies for training, testing and evaluating and improving model performance Cross-validation for parameter optimization Learn to use metrics such as Precision, Recall, F1-score, as well as a confusion matrix to evaluate true model performance You will dive into the theoretical foundation behind each algorithm with the aid of intuitive explanation of formulas and mathematical notions This course is ideal for individuals who are Aspiring data scientists and machine learning engineers or Data Scientists and Data Analysts looking to up their skillset or Anyone who wants to gain an understanding of the machine learning field and its vast opportunities It is particularly useful for Aspiring data scientists and machine learning engineers or Data Scientists and Data Analysts looking to up their skillset or Anyone who wants to gain an understanding of the machine learning field and its vast opportunities.
Enroll now: The Supervised Machine Learning Bootcamp
Summary
Title: The Supervised Machine Learning Bootcamp
Price: $74.99
Average Rating: 4.59
Number of Lectures: 83
Number of Quizzes: 12
Number of Published Lectures: 83
Number of Published Quizzes: 12
Number of Curriculum Items: 95
Number of Published Curriculum Objects: 95
Original Price: $199.99
Quality Status: approved
Status: Live
What You Will Learn
- Regression and Classification Algorithms
- Using sk-learn and Python to implement supervised machine learning techniques
- K-nearest neighbors for both classification and regression
- Naïve Bayes
- Ridge and Lasso Regression
- Decision Trees
- Random Forests
- Support Vector Machines
- Practical case studies for training, testing and evaluating and improving model performance
- Cross-validation for parameter optimization
- Learn to use metrics such as Precision, Recall, F1-score, as well as a confusion matrix to evaluate true model performance
- You will dive into the theoretical foundation behind each algorithm with the aid of intuitive explanation of formulas and mathematical notions
Who Should Attend
- Aspiring data scientists and machine learning engineers
- Data Scientists and Data Analysts looking to up their skillset
- Anyone who wants to gain an understanding of the machine learning field and its vast opportunities
Target Audiences
- Aspiring data scientists and machine learning engineers
- Data Scientists and Data Analysts looking to up their skillset
- Anyone who wants to gain an understanding of the machine learning field and its vast opportunities
Why should you consider taking the Supervised Machine Learning course?
The supervised machine learning algorithms you will learn here are some of the most powerful data science tools you need to solve regression and classification tasks. These are invaluable skills anyone who wants to work as a machine learning engineer and data scientist should have in their toolkit.
Naïve Bayes, KNNs, Support Vector Machines, Decision Trees, Random Forests, Ridge and Lasso Regression.
In this course, you will learn the theory behind all 6 algorithms, and then apply your skills to practical case studies tailored to each one of them, using Python’s sci-kit learn library.
First, we cover naïve Bayes – a powerful technique based on Bayesian statistics. Its strong point is that it’s great at performing tasks in real-time. Some of the most common use cases are filtering spam e-mails, flagging inappropriate comments on social media, or performing sentiment analysis. In the course, we have a practical example of how exactly that works, so stay tuned!
Next up is K-nearest-neighbors – one of the most widely used machine learning algorithms. Why is that? Because of its simplicity when using distance-based metrics to make accurate predictions.
We’ll follow up with decision tree algorithms, which will serve as the basis for our next topic – namely random forests. They are powerful ensemble learners, capable of harnessing the power of multiple decision trees to make accurate predictions.
After that, we’ll meet Support Vector Machines – classification and regression models, capable of utilizing different kernels to solve a wide variety of problems. In the practical part of this section, we’ll build a model for classifying mushrooms as either poisonous or edible. Exciting!
Finally, you’ll learn about Ridge and Lasso Regression – they are regularization algorithms that improve the linear regression mechanism by limiting the power of individual features and preventing overfitting. We’ll go over the differences and similarities, as well as the pros and cons of both regression techniques.
Each section of this course is organized in a uniform way for an optimal learning experience:
– We start with the fundamental theory for each algorithm. To enhance your understanding of the topic, we’ll walk you through a theoretical case, as well as introduce mathematical formulas behind the algorithm.
– Then, we move on to building a model in order to solve a practical problem with it. This is done using Python’s famous sklearn library.
– We analyze the performance of our models with the aid of metrics such as accuracy, precision, recall, and the F1 score.
– We also study various techniques such as grid search and cross-validation to improve the model’s performance.
To top it all off, we have a range of complementary exercises and quizzes, so that you can enhance your skill set. Not only that, but we also offer comprehensive course materials to guide you through the course, which you can consult at any time.
The lessons have been created in 365’s unique teaching style many of you are familiar with. We aim to deliver complex topics in an easy-to-understand way, focusing on practical application and visual learning.
With the power of animations, quiz questions, exercises, and well-crafted course notes, the Supervised Machine Learning course will fulfill all your learning needs.
If you want to take your data science skills to the next level and add in-demand tools to your resume, this course is the perfect choice for you.
Click ‘Buy this course’ to continue your data science journey today!
Course Curriculum
Chapter 1: Introduction
Lecture 1: Introduction
Chapter 2: Setting up the Environment
Lecture 1: Installing Anaconda
Lecture 2: Jupyter Dashboard – Part 1
Lecture 3: Jupyter Dashboard – Part 2
Lecture 4: Installing the relevant packages
Chapter 3: Naïve Bayes
Lecture 1: Motivation
Lecture 2: Bayes' Thought Experiment
Lecture 3: Bayes' Thought Experiment: Assignment
Lecture 4: Bayes' Theorem
Lecture 5: The Ham-or-Spam Example
Lecture 6: The Ham-or-Spam Example: Assignment
Lecture 7: The YouTube Dataset: Creating the Data Frame
Lecture 8: CountVectorizer
Lecture 9: The YouTube Dataset: Preprocessing
Lecture 10: The YouTube Dataset: Preprocessing: Assignment
Lecture 11: The YouTube Dataset: Classification
Lecture 12: The YouTube Dataset: Classification: Assignment
Lecture 13: The YouTube Dataset: Confusion Matrix
Lecture 14: The YouTube Dataset: Accuracy, Precision, Recall, and the F1 score
Lecture 15: The YouTube Dataset: Changing the Priors
Lecture 16: Naïve Bayes: Assignment
Chapter 4: K-Nearest Neighbors
Lecture 1: Motivation
Lecture 2: Math Prerequisites: Distance Metrics
Lecture 3: Random Dataset: Generating the Dataset
Lecture 4: Random Dataset: Visualizing the Dataset
Lecture 5: Random Dataset: Classification
Lecture 6: Random Dataset: How to Break a Tie
Lecture 7: Random Dataset: Decision Regions
Lecture 8: Random Dataset: Choosing the Best K-value
Lecture 9: Random Dataset: Grid Search
Lecture 10: Random Dataset: Model Performance
Lecture 11: KNeighbors Classifier: Assignment
Lecture 12: Theory with a Practical Example
Lecture 13: KNN vs Linear Regression: A Linear Problem
Lecture 14: KNN vs Linear Regression: A Non-linear Problem
Lecture 15: KNeighbors Regressor: Assignment
Lecture 16: Pros and Cons
Chapter 5: Decision Trees and Random Forests
Lecture 1: What is a Tree in Computer Science?
Lecture 2: The Concept of Decision Trees
Lecture 3: Decision Trees in Machine Learning
Lecture 4: Decision Trees: Pros and Cons
Lecture 5: Practical Example: The Iris Dataset
Lecture 6: Practical Example: Creating a Decision Tree
Lecture 7: Practical Example: Plotting the Tree
Lecture 8: Decision Tree Metrics Intuition: Gini Inpurity
Lecture 9: Decision Tree Metrics: Information Gain
Lecture 10: Tree Pruning: Dealing with Overfitting
Lecture 11: Random Forest as Ensemble Learning
Lecture 12: Bootstrapping
Lecture 13: From Bootstrapping to Random Forests
Lecture 14: Random Forest in Code – Glass Dataset
Lecture 15: Census Data and Income – Preprocessing
Lecture 16: Training the Decision Tree
Lecture 17: Training the Random Forest
Chapter 6: Support Vector Machines
Lecture 1: Introduction to Support Vector Machines
Lecture 2: Linearly separable classes – hard margin problem
Lecture 3: Non-linearly separable classes – soft margin problem
Lecture 4: Kernels – Intuition
Lecture 5: Intro to the practical case
Lecture 6: Preprocessing the data
Lecture 7: Splitting the data into train and test and rescaling
Lecture 8: Implementing a linear SVM
Lecture 9: Analyzing the results– Confusion Matrix, Precision, and Recall
Lecture 10: Cross-validation
Lecture 11: Choosing the kernels and C values for cross-validation
Lecture 12: Hyperparameter tuning using GridSearchCV
Lecture 13: Support Vector Machines – Assignment
Chapter 7: Ridge and Lasso Regression
Lecture 1: Regression Analysis Overview
Lecture 2: Overfitting and Multicollinearity
Lecture 3: Introduction to Regularization
Lecture 4: Ridge Regression Basics
Lecture 5: Ridge Regression Mechanics
Lecture 6: Regularization in More Complicated Scenarios
Lecture 7: Lasso Regression Basics
Lecture 8: Lasso Regression vs Ridge Regression
Lecture 9: The Hitters Dataset: Preprocessing and Preparation
Lecture 10: Exploratory Data Analysis
Lecture 11: Performing Linear Regression
Lecture 12: Cross-validation for Choosing a Tuning Parameter
Lecture 13: Performing Ridge Regression with Cross-validation
Lecture 14: Performing Lasso Regression with Cross-validation
Instructors
-
365 Careers
Creating opportunities for Data Science and Finance students
Rating Distribution
- 1 stars: 7 votes
- 2 stars: 13 votes
- 3 stars: 127 votes
- 4 stars: 409 votes
- 5 stars: 568 votes
Frequently Asked Questions
How long do I have access to the course materials?
You can view and review the lecture materials indefinitely, like an on-demand channel.
Can I take my courses with me wherever I go?
Definitely! If you have an internet connection, courses on Udemy are available on any device at any time. If you don’t have an internet connection, some instructors also let their students download course lectures. That’s up to the instructor though, so make sure you get on their good side!
You may also like
- Best Video Editing Courses to Learn in February 2025
- Best Music Production Courses to Learn in February 2025
- Best Animation Courses to Learn in February 2025
- Best Digital Illustration Courses to Learn in February 2025
- Best Renewable Energy Courses to Learn in February 2025
- Best Sustainable Living Courses to Learn in February 2025
- Best Ethical AI Courses to Learn in February 2025
- Best Cybersecurity Fundamentals Courses to Learn in February 2025
- Best Smart Home Technology Courses to Learn in February 2025
- Best Holistic Health Courses to Learn in February 2025
- Best Nutrition And Diet Planning Courses to Learn in February 2025
- Best Yoga Instruction Courses to Learn in February 2025
- Best Stress Management Courses to Learn in February 2025
- Best Mindfulness Meditation Courses to Learn in February 2025
- Best Life Coaching Courses to Learn in February 2025
- Best Career Development Courses to Learn in February 2025
- Best Relationship Building Courses to Learn in February 2025
- Best Parenting Skills Courses to Learn in February 2025
- Best Home Improvement Courses to Learn in February 2025
- Best Gardening Courses to Learn in February 2025