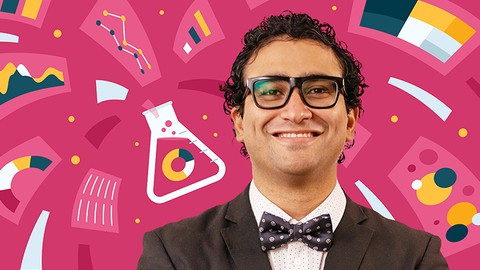
One Week of Data Science in Python – New 2024!
One Week of Data Science in Python – New 2024!, available at $94.99, has an average rating of 4.39, with 145 lectures, based on 283 reviews, and has 3778 subscribers.
You will learn about Perform statistical analysis on real world datasets Understand feature engineering strategies and tools Perform one hot encoding and normalization Understand the difference between normalization and standardization Deal with missing data using pandas Change pandas DataFrame datatypes Define a function and apply it to a Pandas DataFrame column Perform Pandas operations and filtering Calculate and display correlation matrix heatmap Perform data visualization using Seaborn and Matplotlib libraries Plot single line plot, pie charts and multiple subplots using matplotlib Plot pairplot, countplot, and correlation heatmaps using Seaborn Plot distribution plot (distplot), Histograms and scatterplots Understand machine learning regression fundamentals Learn how to optimize model parameters using least sum of squares Split the data into training and testing using SK Learn Library Perform data visualization and basic exploratory data analysis Build, train and test our first regression model in Scikit-Learn Assess trained machine learning regression model performance Understand the theory and intuition behind boosting Train an XG-boost algorithm in Scikit-Learn to solve regression type problems Train several machine learning models classifier models such as Logistic Regression, Support Vector Machine, K-Nearest Neighbors, and Random Forest Classifier Assess trained model performance using various KPIs such as accuracy, precision, recall, F1-score, AUC and ROC. Compare the performance of the classification model using various KPIs. Apply autogluon to solve regression and classification type problems Use AutoGluon library to perform prototyping of AI/ML models using few lines of code Plot various models’ performance on model leaderboard Optimize regression and classification models hyperparameters using SK-Learn Learn the difference between various hyperparameters optimization strategies such as grid search, randomized search, and Bayesian optimization. Perform hyperparameters optimization using Scikit-Learn library. Understand bias variance trade-off and L1 and L2 regularization This course is ideal for individuals who are The course is targeted towards anyone wanting to gain a fundamental understanding of Data Science and solve practical real world business problems or Beginners Data Scientists wanting to advance their careers and build their portfolio or Seasoned consultants wanting to transform businesses by leveraging Data Science or Tech enthusiasts who are passionate and new to Data science & AI and want to gain practical experience It is particularly useful for The course is targeted towards anyone wanting to gain a fundamental understanding of Data Science and solve practical real world business problems or Beginners Data Scientists wanting to advance their careers and build their portfolio or Seasoned consultants wanting to transform businesses by leveraging Data Science or Tech enthusiasts who are passionate and new to Data science & AI and want to gain practical experience.
Enroll now: One Week of Data Science in Python – New 2024!
Summary
Title: One Week of Data Science in Python – New 2024!
Price: $94.99
Average Rating: 4.39
Number of Lectures: 145
Number of Published Lectures: 136
Number of Curriculum Items: 145
Number of Published Curriculum Objects: 136
Original Price: $39.99
Quality Status: approved
Status: Live
What You Will Learn
- Perform statistical analysis on real world datasets
- Understand feature engineering strategies and tools
- Perform one hot encoding and normalization
- Understand the difference between normalization and standardization
- Deal with missing data using pandas
- Change pandas DataFrame datatypes
- Define a function and apply it to a Pandas DataFrame column
- Perform Pandas operations and filtering
- Calculate and display correlation matrix heatmap
- Perform data visualization using Seaborn and Matplotlib libraries
- Plot single line plot, pie charts and multiple subplots using matplotlib
- Plot pairplot, countplot, and correlation heatmaps using Seaborn
- Plot distribution plot (distplot), Histograms and scatterplots
- Understand machine learning regression fundamentals
- Learn how to optimize model parameters using least sum of squares
- Split the data into training and testing using SK Learn Library
- Perform data visualization and basic exploratory data analysis
- Build, train and test our first regression model in Scikit-Learn
- Assess trained machine learning regression model performance
- Understand the theory and intuition behind boosting
- Train an XG-boost algorithm in Scikit-Learn to solve regression type problems
- Train several machine learning models classifier models such as Logistic Regression, Support Vector Machine, K-Nearest Neighbors, and Random Forest Classifier
- Assess trained model performance using various KPIs such as accuracy, precision, recall, F1-score, AUC and ROC.
- Compare the performance of the classification model using various KPIs.
- Apply autogluon to solve regression and classification type problems
- Use AutoGluon library to perform prototyping of AI/ML models using few lines of code
- Plot various models’ performance on model leaderboard
- Optimize regression and classification models hyperparameters using SK-Learn
- Learn the difference between various hyperparameters optimization strategies such as grid search, randomized search, and Bayesian optimization.
- Perform hyperparameters optimization using Scikit-Learn library.
- Understand bias variance trade-off and L1 and L2 regularization
Who Should Attend
- The course is targeted towards anyone wanting to gain a fundamental understanding of Data Science and solve practical real world business problems
- Beginners Data Scientists wanting to advance their careers and build their portfolio
- Seasoned consultants wanting to transform businesses by leveraging Data Science
- Tech enthusiasts who are passionate and new to Data science & AI and want to gain practical experience
Target Audiences
- The course is targeted towards anyone wanting to gain a fundamental understanding of Data Science and solve practical real world business problems
- Beginners Data Scientists wanting to advance their careers and build their portfolio
- Seasoned consultants wanting to transform businesses by leveraging Data Science
- Tech enthusiasts who are passionate and new to Data science & AI and want to gain practical experience
Do you want to learn Data Science and build robust applications Quickly and Efficiently?
Are you an absolute beginner who wants to break into Data Science and look for a course that includes all the basics you need?
Are you a busy aspiring entrepreneur who wants to maximize business revenues and reduce costs with Data Science but don’t have the time to get there quickly and efficiently?
This course is for you if the answer is yes to any of these questions!
Data Science is one of the hottest tech fields to be in now!
The field is exploding with opportunities and career prospects.
Data Science is widely adopted in many sectors, such as banking, healthcare, transportation, and technology.
In business, Data Science is applied to optimize business processes, maximize revenue, and reduce cost.
This course aims to provide you with knowledge of critical aspects of data science in one week and in a practical, easy, quick, and efficient way.
This course is unique and exceptional in many ways. It includes several practice opportunities, quizzes, and final capstone projects.
Every day, we will spend 1-2 hours together and master a data science topic.
First, we will start with the Data Science essential starter pack and master key Data Science Concepts, including the Data Science project lifecycle, what recruiters look for, and what jobs are available.
Next, we will understand exploratory data analysis and visualization techniques using Pandas, matplotlib, and Seaborn libraries.
In the following section, we will learn about regression fundamentals. We will learn how to build, train, test, and deploy regression models using the Scikit Learn library.
In the following section, we will learn about hyperparameter optimization strategies such as grid search, randomized search, and Bayesian optimization.
Next, we will learn how to train several classification algorithms such as Logistic Regression, Support Vector Machine, K-Nearest Neighbors, Random Forest Classifier, and Naïve Bayes in SageMaker and SK-Learn libraries.
Next, we will cover Data Science on Autopilot! We will learn how to use the AutoGluon library for prototyping multiple AI/ML models and deploying the best one.
So who is this course for?
The course targets anyone wanting to gain a fundamental understanding of Data Science and solve practical, real-world business problems.
In this course:
-
You will have an actual practical project-based learning experience. We will build over ten projects together
-
You will have access to all the codes and slides
-
You will get a certificate of completion that you can post on your LinkedIn profile to showcase your skills in Data Science to employers.
-
All this comes with a 30-day money-back guarantee, so you can give a course a try risk-free!
Check out the preview videos and the outline to get an idea of the projects we will cover.
Enroll today, and let’s harness the power of Data Science together!
Course Curriculum
Chapter 1: Course Introduction, Welcome Message & Data Science Starter Pack!
Lecture 1: Course Welcome Message
Lecture 2: Introduction, Best Practices, & Success Tips
Lecture 3: Course Outline
Lecture 4: What is Data Science?
Lecture 5: What is a Typical Data Scientist Profile, Education, Experience & Salary?
Lecture 6: What do Data Scientists REALLY do?
Lecture 7: What do Recruiters Look for in Data Science Applicants?
Lecture 8: What Data Science Jobs are Available Out there?
Chapter 2: Day 1: Data Wrangling, Exploratory Data Analysis (EDA) & Feature Engineering
Lecture 1: Day #1 Welcome Message
Lecture 2: Project Introduction & Data Wrangling 101
Lecture 3: Coding Task #1 – Import Data and Obtain Data Statistical Summary
Lecture 4: Exercise #1 [Optional]
Lecture 5: Coding Task #2 – Deal with Missing Values
Lecture 6: Exercise #2 [Optional]
Lecture 7: Coding Task #3 – Perform One-Hot Encoding
Lecture 8: Exercise #3 [Optional]
Lecture 9: Feature Scaling 101
Lecture 10: Coding Task #4 – Perform Feature Scaling: Normalization & Standardization
Lecture 11: Exercise #4 [Optional]
Lecture 12: Coding Task #5 – Perform Filtering Operation
Lecture 13: Exercise #5 [Optional]
Lecture 14: Coding Task #6 – Perform EDA on Both Classes
Lecture 15: Exercise #6 [Optional]
Lecture 16: Coding Task #7 – Functions With Pandas
Lecture 17: Exercise #7 [Optional]
Lecture 18: Coding Task #8 – Correlations and Histograms
Lecture 19: Exercise #8 [Optional]
Lecture 20: Final Capstone Project Overview
Lecture 21: Final Capstone Project Solution
Lecture 22: Day #1 Conclusion
Chapter 3: Day 2: Effective Data Visualization in Data Science
Lecture 1: Day #2 Welcome Message
Lecture 2: Project Overview and Key Learning Outcomes
Lecture 3: Data Visualization 101
Lecture 4: Coding Task #1 – Plot Pie Charts with Matplotlib
Lecture 5: Exercise #1 [Optional]
Lecture 6: Coding Task #2 – Plot Single & Multiple LinePlots
Lecture 7: Exercise #2 [Optional]
Lecture 8: Coding Task #3 – Plot Scatterplots
Lecture 9: Exercise #3 [Optional]
Lecture 10: Coding Task #4 – Plot Histograms
Lecture 11: Exercise #4 [Optional]
Lecture 12: Coding Task #5 – Searborn Part 1
Lecture 13: Exercise #5 [Optional]
Lecture 14: Coding Task #6 – Searborn Part 2
Lecture 15: Exercise #6 [Optional]
Lecture 16: Final Capstone Project Overview
Lecture 17: Final Capstone Project Solution
Lecture 18: Day #2 Conclusion
Chapter 4: Day 3: Regression Analysis in Data Science
Lecture 1: Day #3 Welcome Message
Lecture 2: Project Overview and Key Learning Outcomes
Lecture 3: What is Regression?
Lecture 4: What is XGBoost? and Why?
Lecture 5: Coding Task #1 – Import Libraries and Datasets
Lecture 6: Exercise #1 [Optional]
Lecture 7: Coding Task #2 – Exploratory Data Analysis (EDA) & Visualization
Lecture 8: Exercise #2 [Optional]
Lecture 9: Coding Task #3 – Prepare The Data Before Model Training
Lecture 10: Exercise #3 [Optional]
Lecture 11: Coding Task #4 – Train an XG-Boost Model
Lecture 12: Exercise #4 [Optional]
Lecture 13: Final Capstone Project Overview
Lecture 14: Final Capstone Project Solution
Lecture 15: Day #3 Conclusion
Chapter 5: Day 4: Classification Analysis in Data Science
Lecture 1: Day #4 Welcome Message
Lecture 2: Introduction & Project Overview
Lecture 3: Classification Models KPIs
Lecture 4: Coding Task #1 – Import Libraries and Datasets
Lecture 5: Exercise #1 [Optional]
Lecture 6: Coding Task #2 – Perform Data Visualization
Lecture 7: Exercise #2 [Optional]
Lecture 8: Coding Task #3 – Plot Feature Importance
Lecture 9: Coding Task #4 – Train & Evaluate a Logistic Regression Classifier Model
Lecture 10: Exercise #3 [Optional]
Lecture 11: Coding Task #5 – Train & Evaluate Support Vector Machines
Lecture 12: Coding Task #6 – Train & Evaluate Random Forest Classifier Model
Lecture 13: Coding Task #7 – Train & Evaluate K-Nearest Neighbors Classifier
Lecture 14: Exercise #4 [Optional]
Lecture 15: Coding Task #8 – Train & Evaluate a Naïve Bayes Classifier Model
Lecture 16: Exercise #5 [Optional]
Lecture 17: Coding Task #9 – Compare Classifier Models
Lecture 18: Coding Task #10 – Concluding Remarks
Lecture 19: Final Capstone Project
Lecture 20: Final Capstone Project Solution Part #1
Lecture 21: Final Capstone Project Solution Part #2
Lecture 22: Final Capstone Project Solution Part #3
Lecture 23: Day #4 Conclusion
Chapter 6: Day 5: Data Science on Autopilot
Lecture 1: Day #5 Welcome Message
Lecture 2: Introduction and Project Overview
Lecture 3: What is AutoGluon?
Lecture 4: Coding Task #1 – Import AutoGluon, Key Libraries & Datasets
Lecture 5: Exercise #1 [Optional]
Lecture 6: Coding Task #2 – Perform Exploratory Data Analysis (EDA)
Lecture 7: Exercise #2 [Optional]
Lecture 8: Coding Task #3 – Perform Data Visualization
Instructors
-
Dr. Ryan Ahmed, Ph.D., MBA
Best-Selling Professor, 400K+ students, 250K+ YT Subs -
SuperDataScience Team
Helping Data Scientists Succeed -
Ligency Team
Helping Data Scientists Succeed
Rating Distribution
- 1 stars: 4 votes
- 2 stars: 2 votes
- 3 stars: 13 votes
- 4 stars: 85 votes
- 5 stars: 179 votes
Frequently Asked Questions
How long do I have access to the course materials?
You can view and review the lecture materials indefinitely, like an on-demand channel.
Can I take my courses with me wherever I go?
Definitely! If you have an internet connection, courses on Udemy are available on any device at any time. If you don’t have an internet connection, some instructors also let their students download course lectures. That’s up to the instructor though, so make sure you get on their good side!
You may also like
- Digital Marketing Foundation Course
- Google Shopping Ads Digital Marketing Course
- Multi Cloud Infrastructure for beginners
- Master Lead Generation: Grow Subscribers & Sales with Popups
- Complete Copywriting System : write to sell with ease
- Product Positioning Masterclass: Unlock Market Traction
- How to Promote Your Webinar and Get More Attendees?
- Digital Marketing Courses
- Create music with Artificial Intelligence in this new market
- Create CONVERTING UGC Content So Brands Will Pay You More
- Podcast: The top 8 ways to monetize by Podcasting
- TikTok Marketing Mastery: Learn to Grow & Go Viral
- Free Digital Marketing Basics Course in Hindi
- MailChimp Free Mailing Lists: MailChimp Email Marketing
- Automate Digital Marketing & Social Media with Generative AI
- Google Ads MasterClass – All Advanced Features
- Online Course Creator: Create & Sell Online Courses Today!
- Introduction to SEO – Basic Principles of SEO
- Affiliate Marketing For Beginners: Go From Novice To Pro
- Effective Website Planning Made Simple