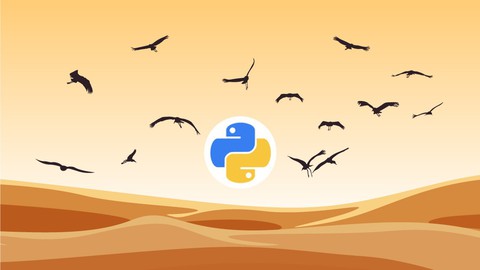
Bio-inspired Artificial Intelligence Algorithms
Bio-inspired Artificial Intelligence Algorithms, available at $79.99, has an average rating of 4.25, with 88 lectures, based on 146 reviews, and has 1713 subscribers.
You will learn about Understand the theory and practice of the main bio-inspired artificial intelligence algorithms Solve real-world optimization problems using bio-inspired algorithms Minimize the price of airline tickets using Genetic Algorithms Create custom menus using Differential Evolution Classify handwritten digits using Artificial Neural Networks Adapt antibodies and antigens with the Clonal Selection algorithm, applied in digit recognition Optimize course schedules using Particle Swarm Optimization Solve shortest paths problems using Ant Colony Optimization This course is ideal for individuals who are People interested in how nature can provide inspiration for Computer Science problems or People interested in artificial intelligence algorithms, especially those inspired in Biology or Developers who want to solve real optimization and classification problems or Data Scientists who want to increase their portfolio It is particularly useful for People interested in how nature can provide inspiration for Computer Science problems or People interested in artificial intelligence algorithms, especially those inspired in Biology or Developers who want to solve real optimization and classification problems or Data Scientists who want to increase their portfolio.
Enroll now: Bio-inspired Artificial Intelligence Algorithms
Summary
Title: Bio-inspired Artificial Intelligence Algorithms
Price: $79.99
Average Rating: 4.25
Number of Lectures: 88
Number of Published Lectures: 88
Number of Curriculum Items: 88
Number of Published Curriculum Objects: 88
Original Price: $19.99
Quality Status: approved
Status: Live
What You Will Learn
- Understand the theory and practice of the main bio-inspired artificial intelligence algorithms
- Solve real-world optimization problems using bio-inspired algorithms
- Minimize the price of airline tickets using Genetic Algorithms
- Create custom menus using Differential Evolution
- Classify handwritten digits using Artificial Neural Networks
- Adapt antibodies and antigens with the Clonal Selection algorithm, applied in digit recognition
- Optimize course schedules using Particle Swarm Optimization
- Solve shortest paths problems using Ant Colony Optimization
Who Should Attend
- People interested in how nature can provide inspiration for Computer Science problems
- People interested in artificial intelligence algorithms, especially those inspired in Biology
- Developers who want to solve real optimization and classification problems
- Data Scientists who want to increase their portfolio
Target Audiences
- People interested in how nature can provide inspiration for Computer Science problems
- People interested in artificial intelligence algorithms, especially those inspired in Biology
- Developers who want to solve real optimization and classification problems
- Data Scientists who want to increase their portfolio
Nature offers a wide range of inspirations for biological processes to be incorporated into technology and computing. Some of these processes and patterns have been inspiring the development of algorithms that can be used to solve real-world problems. They are called bio-inspired algorithms, whose inspiration in nature allows for applications in various optimization and classification problems.
In this course, you will learn the theoretical and mainly the practical implementation of the main and mostly used bio-inspired algorithms! By the end of the course you will have all the tools you need to build artificial intelligence solutions that can be applied to your own problems! The course is divided into six sections that cover different algorithms applied in real-world case studies. See below the projects that will be implemented step by step:
-
Genetic Algorithms (GA): It is one of the most used and well-known bio-inspired algorithm to solve optimization problems. It is based on biological evolution in which populations of individuals evolve over generations through mutation, selection, and crossing over. We will solve the flight schedule problem and the goal is to minimize the price of air line tickets and the time spend waiting at the airport.
-
Differential Evolution (DE): It is also inspired in biological evolution and the case study we will solve step by step is the creation of menus, correctly balancing the amount of carbohydrates, proteins and fats.
-
Neural Networks (ANN): It is based on how biological neurons work and is considered one of the most modern techniques to solve complex problems, such as: chatbots, automatic translators, self driving cars, voice recognition, among many others. The case study will be the creation of a neural network for image classification.
-
Clonal Selection Algorithm (CSA): It is based on the functioning of the optimization of the antibody response against an antigen, resembling the process of biological evolution. These concepts will be used in practice for digit identification and digit generation.
-
Particle Swarm Optimization (PSO): It relies on the social behavior of animals, in which the swarm tries to find the best solution to a specific problem. The problem to be solved will be the timetable: there is a course, people who want to take it and different timetables. In the end, the algorithm will indicate the best times for each class to take the course.
-
Ant Colony Optimization (ACO): It is based on concepts of how ants search for food in nature. The case study will be one of the most classic in the area, which is the choice of the shortest path.
Each type of problem requires different techniques for its solution. When you understand the intuition and implementation of bio-inspired algorithms, it is easier to identify which techniques are the best to be applied in each scenario. During the course, all the code will be implemented step by step using the Python programming language! We are going to use Google Colab, so you do not have to worry about installing libraries on your machine, as everything will be developed online using Google’s GPUs!
Course Curriculum
Chapter 1: Introduction
Lecture 1: Couse content
Lecture 2: Course materials
Chapter 2: Genetic Algorithms
Lecture 1: Case study – flight schedule
Lecture 2: Creating the variables
Lecture 3: Flights dataset
Lecture 4: Printing the schedule
Lecture 5: Hours to minutes
Lecture 6: Fitness function 1
Lecture 7: Fitness function 2
Lecture 8: Genetic algorithm – intuition
Lecture 9: Part 1 – mutation
Lecture 10: Part 2 – crossover
Lecture 11: Part 3 – complete genetic algorithm
Lecture 12: Part 4 – complete genetic algorithm
Lecture 13: Part 5 – complete genetic algorithm
Chapter 3: Differential Evolution
Lecture 1: Introduction to the algorithm
Lecture 2: General structure of the algorithm
Lecture 3: The variation operator and the generation of new vectors
Lecture 4: Main differences between DE and GA
Lecture 5: Application: nutrient allocation problem
Lecture 6: Part 1 – Candidate solution
Lecture 7: Part 2 – Population of vectors
Lecture 8: Part 3 – Objective/fitness function
Lecture 9: Part 4 – selecting three other vectors
Lecture 10: Part 5 – variation operator
Lecture 11: Part 6 – selecting the best vector from each population
Lecture 12: Part 7 – running the algorithm
Lecture 13: Part 8 – solution graph
Chapter 4: Artificial Neural Networks
Lecture 1: Biological fundamentals
Lecture 2: Single layer perceptron
Lecture 3: Multi-layer networks – sum and activation functions
Lecture 4: Multi-layer networks – error calculation
Lecture 5: Gradient descent
Lecture 6: Delta parameter
Lecture 7: Adjusting the weights with backpropagation
Lecture 8: Bias, error, stochastic gradient descent, and more concepts
Lecture 9: Part 1 – digits dataset
Lecture 10: Part 2 – pre-processing the images
Lecture 11: Part 3 – training
Lecture 12: Part 4 – evaluating
Lecture 13: Part 5 – classifying one single image
Chapter 5: Clonal Selection Algorithm
Lecture 1: Clonal Selection Algorithm
Lecture 2: General structure of the algorithm
Lecture 3: Calculating the cloning factor
Lecture 4: Calculation of hypermutation
Lecture 5: Application – Digit generation/recognition
Lecture 6: Part 1 – antibody function
Lecture 7: Part 2 – antibody population
Lecture 8: Part 3 – fitness function
Lecture 9: Part 4 – antibody affinity list
Lecture 10: Part 5 – selecting the N best antibodies
Lecture 11: Part 6 – cloning the best antibodies
Lecture 12: Part 7 – Hypermutation of the antibodies
Lecture 13: Part 8 – Running the algorithm
Lecture 14: Part 9 – Solution graph
Chapter 6: Particle Swarm Optimization
Lecture 1: Introduction to the algorithm
Lecture 2: General structure of the algorithm
Lecture 3: Particles and the population (swarm)
Lecture 4: Individual best particle and Global best particle
Lecture 5: Updating the position and velocity of the particles
Lecture 6: Graphical/vectorial representation of position/velocity update
Lecture 7: Case study
Lecture 8: Part 1 – Particle
Lecture 9: Part 2 – Population
Lecture 10: Part 3 – Fitness function
Lecture 11: Part 4 – Personal best position (pbest)
Lecture 12: Part 5 – Global best position (gbest)
Lecture 13: Part 6 – Updating the position and velocity of the particle
Lecture 14: Part 7 – New position/particle
Lecture 15: Part 8 – Running the algorithm
Lecture 16: Part 9 – Solution graph
Chapter 7: Ant Colony Optimization
Lecture 1: Foraging behavior of ants
Lecture 2: Foraging behavior of ants: part 2
Lecture 3: Update of pheromone deposition
Lecture 4: Probability of edge selection
Lecture 5: Ants and the TSP problem
Lecture 6: Case study
Lecture 7: Part 1 – Edges
Lecture 8: Part 2 – Edge selection probability
Lecture 9: Part 3 – Function that chooses edges
Lecture 10: Part 4 – Generating paths/ants
Lecture 11: Part 5 – Path length function
Lecture 12: Part 6 – Pheromone update
Lecture 13: Part 7 – Running the algorithm
Lecture 14: Part 8 – 5 nodes
Lecture 15: Part 9 – Running the algorithm with 5 nodes
Chapter 8: Final remarks
Lecture 1: Final remarks
Lecture 2: BONUS
Instructors
-
Jones Granatyr
Professor -
Guilherme Matos Passarini, phD
Professor -
AI Expert Academy
Instructor
Rating Distribution
- 1 stars: 2 votes
- 2 stars: 0 votes
- 3 stars: 19 votes
- 4 stars: 46 votes
- 5 stars: 79 votes
Frequently Asked Questions
How long do I have access to the course materials?
You can view and review the lecture materials indefinitely, like an on-demand channel.
Can I take my courses with me wherever I go?
Definitely! If you have an internet connection, courses on Udemy are available on any device at any time. If you don’t have an internet connection, some instructors also let their students download course lectures. That’s up to the instructor though, so make sure you get on their good side!
You may also like
- Best Interior Design Courses to Learn in March 2025
- Best Writing Courses Courses to Learn in March 2025
- Best Storytelling Courses to Learn in March 2025
- Best Creativity Workshops Courses to Learn in March 2025
- Best Resilience Training Courses to Learn in March 2025
- Best Emotional Intelligence Courses to Learn in March 2025
- Best Time Management Courses to Learn in March 2025
- Best Remote Work Strategies Courses to Learn in March 2025
- Best Freelancing Courses to Learn in March 2025
- Best E-commerce Strategies Courses to Learn in March 2025
- Best Personal Branding Courses to Learn in March 2025
- Best Stock Market Trading Courses to Learn in March 2025
- Best Real Estate Investing Courses to Learn in March 2025
- Best Financial Technology Courses to Learn in March 2025
- Best Agile Methodologies Courses to Learn in March 2025
- Best Project Management Courses to Learn in March 2025
- Best Leadership Skills Courses to Learn in March 2025
- Best Public Speaking Courses to Learn in March 2025
- Best Affiliate Marketing Courses to Learn in March 2025
- Best Email Marketing Courses to Learn in March 2025