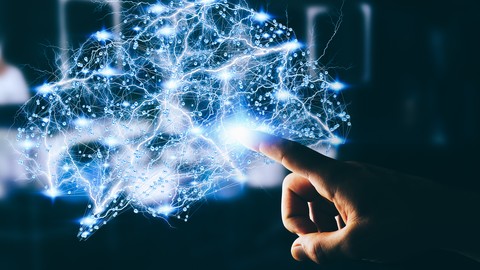
Neural Networks with TensorFlow – A Complete Guide!: 3-in-1
Neural Networks with TensorFlow – A Complete Guide!: 3-in-1, available at $44.99, has an average rating of 3.81, with 76 lectures, 2 quizzes, based on 8 reviews, and has 124 subscribers.
You will learn about Get hands-on and understand Advanced Neural Networks with TensorFlow. Develop an autonomous agent in an Atari environment with OpenAI Gym Apply NLP and sentiment analysis to your data. Improve the network by understanding the activation function. Explore the input and output of different games in OpenAI Gym. Generate new images using variational autoencoders. Perform encoding MNIST characters in Autoencoders. Implement neural networks and improve predictions This course is ideal for individuals who are Data scientists who are familiar with Python and perform machine learning activities on a day-to-day basis, who are looking forward to build powerful Deep Learning models. It is particularly useful for Data scientists who are familiar with Python and perform machine learning activities on a day-to-day basis, who are looking forward to build powerful Deep Learning models.
Enroll now: Neural Networks with TensorFlow – A Complete Guide!: 3-in-1
Summary
Title: Neural Networks with TensorFlow – A Complete Guide!: 3-in-1
Price: $44.99
Average Rating: 3.81
Number of Lectures: 76
Number of Quizzes: 2
Number of Published Lectures: 76
Number of Published Quizzes: 2
Number of Curriculum Items: 78
Number of Published Curriculum Objects: 78
Original Price: $199.99
Quality Status: approved
Status: Live
What You Will Learn
- Get hands-on and understand Advanced Neural Networks with TensorFlow.
- Develop an autonomous agent in an Atari environment with OpenAI Gym
- Apply NLP and sentiment analysis to your data.
- Improve the network by understanding the activation function.
- Explore the input and output of different games in OpenAI Gym.
- Generate new images using variational autoencoders.
- Perform encoding MNIST characters in Autoencoders.
- Implement neural networks and improve predictions
Who Should Attend
- Data scientists who are familiar with Python and perform machine learning activities on a day-to-day basis, who are looking forward to build powerful Deep Learning models.
Target Audiences
- Data scientists who are familiar with Python and perform machine learning activities on a day-to-day basis, who are looking forward to build powerful Deep Learning models.
Tensorflow is Google’s popular offering for machine learning and deep learning. It has become a popular choice of tool for performing fast, efficient, and accurate Deep Learning. TensorFlow is one of the newest and most comprehensive libraries for implementing Deep Learning and building CNNs. Neural Networks are at the forefront of almost all recent major technology breakthroughs. The intersection of big data, parallel programming, and AI generated a new wave of Neural Network research.
Are you looking forward to getting hands-on and use Deep Learning to build CNNs and train efficient Neural Networks? If yes, then this is the course perfect for you!
This comprehensive 3-in-1 course takes a solution-based approach where every topic is explicated with the help of a real-world example. Use Tensorflow to implement different kinds of Neural Networks – from simple feedforward Neural Networks to multi layered perceptrons, CNNs, RNNs and more! Moreover, Implement multi layered perceptrons, CNN, and more using Tensorflow!
By the end of the course, you’ll not just be able to build powerful Deep Learning models, but also accelerate the training of your models and scale them as required.
Contents and Overview
This training program includes 3 complete courses, carefully chosen to give you the most comprehensive training possible.
The first course, Learning Neural Networks with Tensorflow, covers Neural Networks by solving real real-world datasets using Tensorflow. In this course, you’ll start by building a simple flower recognition program, making you feel comfortable with Tensorflow, and it will teach you several important concepts in Neural Networks. Next, you’ll start working with high-dimensional uses to predict one output: 1275 molecular features you can use to predict the atomization energy of an atom. The next program we’ll create is a handwritten number recognition system trained on the famous MNIST dataset. In the final program, estimate what a celebrity looks like, checking for new pictures to see whether a celebrity is attractive, wears a hat, has lipstick on, and many more properties that are difficult to estimate with “traditional” computer vision techniques.
After the course, you’ll not only be able to build a Neural Network for your own dataset, you’ll also be able to reason which techniques will improve your Neural Network.
The second course, Advanced Neural Networks with Tensorflow, covers getting hands-on to understand Advanced Neural Networks with TensorFlow. You’ll explore Deep Reinforcement Learning algorithms such as Generative Networks and Deep Q Learning. You will learn to implement some more complex types of neural networks such as Deep Q Learning with OpenAI Gym, autoencoders, and Siamese neural networks. During the course of the video, you will be working on real-world datasets to get a hands-on understanding of neural network programming. You will also get to train generative models and will learn Autoencoder applications.
By the end of this course, you will have a fair understanding of how you can leverage the power of TensorFlow to train neural networks of varying complexities, without any hassle.
The third course, TensorFlow for Neural Network Solutions, covers exploring high-level concepts such as neural networks, CNN and RNN using TensorFlow. This course covers important high-level concepts such as neural networks, CNN, RNN, and NLP. Once you are familiar and comfortable with the TensorFlow ecosystem, the last section will show you how to take it to production. Once you are familiar and comfortable with the TensorFlow ecosystem, the last section will show you how to take it to production.
By the end of the course, you’ll not just be able to build powerful Deep Learning models, but also accelerate the training of your models and scale them as required.
About the Authors
● Roland Meertensis currently developing computer vision algorithms for self-driving cars. Previously he has worked as a research engineer at a translation department. Examples of things he has made are a Neural Machine Translation implementation, a post-editor, and a tool that estimates the quality of a translated sentence. Last year, he worked at the Micro Aerial Vehicle Laboratory at the university of Delft, on indoor localization (SLAM) and obstacle avoidance behaviors for a drone that delivers food inside a restaurant. Another thing he worked on was detecting and following people using onboard computer vision algorithms on a stereo camera. For his Master’s thesis, he did an internship at a company called SpirOps, where he worked on the development of a dialogue manager for project Romeo. In his Artificial Intelligence study, he specialized in cognitive artificial intelligence and brain-computer interfacing. His research interests lie in machine learning techniques, human-robot interaction, brain-computer interfaces, and human-computer interaction.
● Nick McClure is currently a senior data scientist at PayScale, Inc. in Seattle, WA. Prior to this, he has worked at Zillow Group and Caesars Entertainment Corporation. He got his degrees in Applied Mathematics from The University of Montana and the College of Saint Benedict and Saint John’s University. He has a passion for learning and advocating for analytics, machine learning, and artificial intelligence.
Course Curriculum
Chapter 1: Learning Neural Networks with Tensorflow
Lecture 1: The Course Overview
Lecture 2: Solving Public Datasets
Lecture 3: Why We Use Docker and Installation Instructions
Lecture 4: Our Code, in a Jupyter Notebook
Lecture 5: Understanding TensorFlow
Lecture 6: The Iris Dataset
Lecture 7: The Human Brain and How to Formalize It
Lecture 8: Backpropagation
Lecture 9: Overfitting — Why We Split Our Train and Test Data
Lecture 10: Ground State Energies of 16,242 Molecules
Lecture 11: First Approach – Easy Layer Building
Lecture 12: Preprocessing Data
Lecture 13: Understanding the Activation Function
Lecture 14: The Importance of Hyperparameters
Lecture 15: Images of Written Digits
Lecture 16: Dense Layer Approach
Lecture 17: Convolution and Pooling Layers
Lecture 18: Convolution and Pooling Layers (Continued)
Lecture 19: From Activations to Probabilities – the Softmax Function
Lecture 20: Optimization and Loss Functions
Lecture 21: Large-Scale CelebFaces Attributes (CelebA) Dataset
Lecture 22: Building an Input Pipeline in TensorFlow
Lecture 23: Building a Convolutional Neural Network
Lecture 24: Batch Normalization
Lecture 25: Understanding What Your Network Learned –Visualizing Activations
Chapter 2: Advanced Neural Networks with Tensorflow
Lecture 1: The Course Overview
Lecture 2: The Approach of This Course
Lecture 3: Installing Docker and Downloading the Source Code for This Course
Lecture 4: Understanding Jupyter Notebooks and TensorFlow
Lecture 5: Visualizing Your Graph
Lecture 6: Adding Summaries
Lecture 7: Plotting the Weights in a Histogram
Lecture 8: Inspecting Input and Output
Lecture 9: Encoding MNIST Characters
Lecture 10: Practical Application –Denoising
Lecture 11: The Dropout Layer
Lecture 12: Variational Autoencoders
Lecture 13: The Omniglot Dataset
Lecture 14: What Is a Siamese Neural Network?
Lecture 15: Training and Testing a Siamese Neural Network
Lecture 16: Alternative Loss Functions
Lecture 17: Speed of Your Network
Lecture 18: Getting Started with the OpenAI Gym
Lecture 19: Random Search
Lecture 20: Reinforcement Learning Explained
Lecture 21: Reinforcement Learning Explained (Continued)
Lecture 22: Reinforcement Learning Tricks
Lecture 23: Playing Atari Games
Lecture 24: Defining Our Network
Lecture 25: Starting and Training a Session
Chapter 3: TensorFlow for Neural Network Solution
Lecture 1: The Course Overview
Lecture 2: Implementing Operational Gates
Lecture 3: Working with Gates and Activation Functions
Lecture 4: Implementing a One-Layer Neural Network
Lecture 5: Implementing Different Layers
Lecture 6: Learning to Play Tic-Tac-Toe
Lecture 7: Working with Bag-of-Words
Lecture 8: Implementing TF-IDF
Lecture 9: Working with Skip-Gram Embeddings
Lecture 10: Working with CBOW Embeddings
Lecture 11: Implementing a Simpler CNN
Lecture 12: Implementing an Advanced CNN
Lecture 13: Applying Stylenet/Neural-Style
Lecture 14: Implementing RNN for Spam Prediction
Lecture 15: Implementing an LSTM Model
Lecture 16: Stacking Multiple LSTM Layers
Lecture 17: Training a Siamese Similarity Measure
Lecture 18: Implementing Unit Tests
Lecture 19: Using Multiple Executors
Lecture 20: Parallelizing TensorFlow
Lecture 21: Production Tips for TensorFlow
Lecture 22: Productionalizing TensorFlow – An Example
Lecture 23: Visualizing Graphs in TensorBoard
Lecture 24: Working with a Genetic Algorithm
Lecture 25: Clustering Using K-Means
Lecture 26: Solving a System of ODEs
Instructors
-
Packt Publishing
Tech Knowledge in Motion
Rating Distribution
- 1 stars: 1 votes
- 2 stars: 0 votes
- 3 stars: 2 votes
- 4 stars: 3 votes
- 5 stars: 2 votes
Frequently Asked Questions
How long do I have access to the course materials?
You can view and review the lecture materials indefinitely, like an on-demand channel.
Can I take my courses with me wherever I go?
Definitely! If you have an internet connection, courses on Udemy are available on any device at any time. If you don’t have an internet connection, some instructors also let their students download course lectures. That’s up to the instructor though, so make sure you get on their good side!
You may also like
- Best Video Editing Courses to Learn in March 2025
- Best Music Production Courses to Learn in March 2025
- Best Animation Courses to Learn in March 2025
- Best Digital Illustration Courses to Learn in March 2025
- Best Renewable Energy Courses to Learn in March 2025
- Best Sustainable Living Courses to Learn in March 2025
- Best Ethical AI Courses to Learn in March 2025
- Best Cybersecurity Fundamentals Courses to Learn in March 2025
- Best Smart Home Technology Courses to Learn in March 2025
- Best Holistic Health Courses to Learn in March 2025
- Best Nutrition And Diet Planning Courses to Learn in March 2025
- Best Yoga Instruction Courses to Learn in March 2025
- Best Stress Management Courses to Learn in March 2025
- Best Mindfulness Meditation Courses to Learn in March 2025
- Best Life Coaching Courses to Learn in March 2025
- Best Career Development Courses to Learn in March 2025
- Best Relationship Building Courses to Learn in March 2025
- Best Parenting Skills Courses to Learn in March 2025
- Best Home Improvement Courses to Learn in March 2025
- Best Gardening Courses to Learn in March 2025