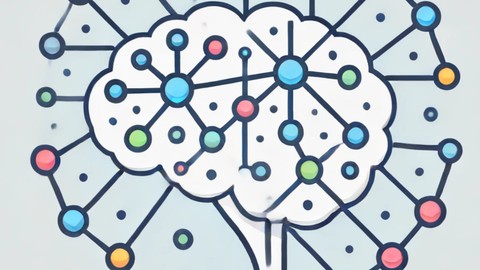
Introduction to Machine Learning
Introduction to Machine Learning, available at $54.99, has an average rating of 4, with 91 lectures, 1 quizzes, based on 2 reviews, and has 8 subscribers.
You will learn about Understand Machine Learning Fundamentals Preprocess Data: Perform data cleaning, handle missing values, and conduct feature engineering on datasets. Normalize and Standardize Data: Apply normalization and standardization techniques to prepare data for machine learning models. Explore Data Visually: Use data visualization techniques to understand data distributions and identify patterns. Perform Statistical Analysis: Conduct basic statistical analysis to summarize data characteristics. Analyze and preprocess the datasets to prepare it for machine learning modeling. Build a Simple Models: Construct a logistic regression model to predict outcomes. Evaluate Model Performance: Use accuracy, precision, recall, and F1-score to evaluate the performance of machine learning models. Split Data into Training and Testing Sets: Implement data splitting techniques to create training and testing datasets. Predict House Prices: Build and evaluate a regression model using the California housing dataset. Deploy a Machine Learning Model: Develop and deploy a machine learning model as a web application using Flask. This course is ideal for individuals who are Aspiring Data Scientists and Machine Learning Enthusiasts or Beginners with No Prior Experience or Programmers and software developers looking to expand their skill set by incorporating machine learning into their repertoire. or Data Analysts and Statisticians or Students and academics in fields related to computer science, mathematics, engineering, or data science. or Professionals from various industries (e.g., finance, healthcare, marketing) who want to understand how machine learning can be applied to their domain. or Individuals who enjoy learning new technologies and skills for personal enrichment. It is particularly useful for Aspiring Data Scientists and Machine Learning Enthusiasts or Beginners with No Prior Experience or Programmers and software developers looking to expand their skill set by incorporating machine learning into their repertoire. or Data Analysts and Statisticians or Students and academics in fields related to computer science, mathematics, engineering, or data science. or Professionals from various industries (e.g., finance, healthcare, marketing) who want to understand how machine learning can be applied to their domain. or Individuals who enjoy learning new technologies and skills for personal enrichment.
Enroll now: Introduction to Machine Learning
Summary
Title: Introduction to Machine Learning
Price: $54.99
Average Rating: 4
Number of Lectures: 91
Number of Quizzes: 1
Number of Published Lectures: 91
Number of Published Quizzes: 1
Number of Curriculum Items: 92
Number of Published Curriculum Objects: 92
Original Price: $49.99
Quality Status: approved
Status: Live
What You Will Learn
- Understand Machine Learning Fundamentals
- Preprocess Data: Perform data cleaning, handle missing values, and conduct feature engineering on datasets.
- Normalize and Standardize Data: Apply normalization and standardization techniques to prepare data for machine learning models.
- Explore Data Visually: Use data visualization techniques to understand data distributions and identify patterns.
- Perform Statistical Analysis: Conduct basic statistical analysis to summarize data characteristics.
- Analyze and preprocess the datasets to prepare it for machine learning modeling.
- Build a Simple Models: Construct a logistic regression model to predict outcomes.
- Evaluate Model Performance: Use accuracy, precision, recall, and F1-score to evaluate the performance of machine learning models.
- Split Data into Training and Testing Sets: Implement data splitting techniques to create training and testing datasets.
- Predict House Prices: Build and evaluate a regression model using the California housing dataset.
- Deploy a Machine Learning Model: Develop and deploy a machine learning model as a web application using Flask.
Who Should Attend
- Aspiring Data Scientists and Machine Learning Enthusiasts
- Beginners with No Prior Experience
- Programmers and software developers looking to expand their skill set by incorporating machine learning into their repertoire.
- Data Analysts and Statisticians
- Students and academics in fields related to computer science, mathematics, engineering, or data science.
- Professionals from various industries (e.g., finance, healthcare, marketing) who want to understand how machine learning can be applied to their domain.
- Individuals who enjoy learning new technologies and skills for personal enrichment.
Target Audiences
- Aspiring Data Scientists and Machine Learning Enthusiasts
- Beginners with No Prior Experience
- Programmers and software developers looking to expand their skill set by incorporating machine learning into their repertoire.
- Data Analysts and Statisticians
- Students and academics in fields related to computer science, mathematics, engineering, or data science.
- Professionals from various industries (e.g., finance, healthcare, marketing) who want to understand how machine learning can be applied to their domain.
- Individuals who enjoy learning new technologies and skills for personal enrichment.
Welcome to “Machine Learning for Beginners: ” your gateway to understanding and mastering the foundational concepts of machine learning. This course is meticulously designed for those who are eager to dive into the world of machine learning, regardless of their prior experience. Whether you are a complete beginner, a student, a professional looking to expand your skill set, or a hobbyist with a keen interest in technology, this course will equip you with the essential knowledge and practical skills to start your journey in machine learning.
Course Overview
Machine learning is a transformative technology that is reshaping industries and driving innovation across various domains. This course aims to demystify machine learning by breaking down complex concepts into manageable, easy-to-understand modules. We will cover everything from the basics of machine learning to deploying your models in real-world applications.
The course begins with an introduction to the fundamental concepts of machine learning. You will learn about the different types of machine learning, including supervised, unsupervised, and reinforcement learning, and explore their real-world applications. This foundational knowledge will set the stage for more in-depth exploration and hands-on practice.
Hands-On Learning with Real-World Datasets
A significant focus of this course is hands-on learning using real-world datasets. We will start with the Titanic dataset, a classic dataset used in machine learning tutorials, to introduce you to essential concepts and techniques. You will learn how to preprocess data, handle missing values, and perform feature engineering to prepare your data for modeling. Through exploratory data analysis (EDA), you will visualize and analyze data patterns, gaining crucial insights for building effective models.
Building and Evaluating Machine Learning Models
You will build your first machine learning model using logistic regression to predict Titanic passenger survival. This step-by-step approach will guide you through the entire process, from understanding the problem to evaluating the model’s performance. You will also build a second machine learning model using logistic regression to predict house prices in California. You will learn to evaluate model performance using various metrics such as accuracy, precision, recall, and F1-score.
Deploying Machine Learning Models
Understanding how to build models is crucial, but knowing how to deploy them is equally important. This course will guide you through deploying your machine learning models as web applications using Flask, a lightweight web framework for Python.
As a capstone project, you will use the California housing dataset to build and deploy a house price prediction model. This project will provide you with hands-on experience in handling a different type of dataset and using regression techniques to make predictions.
This course is ideal for:
-
Aspiring Data Scientists and Machine Learning Enthusiasts: Those looking to build a strong foundation in machine learning.
-
Beginners with No Prior Experience: Complete beginners who want an accessible introduction to machine learning.
-
Programmers and Software Developers: Professionals seeking to incorporate machine learning into their skill set.
-
Data Analysts and Statisticians: Individuals looking to transition into machine learning roles.
-
Students and Academics: Those studying related fields and seeking practical, hands-on experience.
-
Professionals in Various Industries: Individuals in finance, healthcare, marketing, and other sectors wanting to understand and apply machine learning in their domain.
-
Hobbyists and Lifelong Learners: Anyone interested in exploring new technologies and enhancing their knowledge.
Conclusion
By the end of this course, you will have a solid understanding of machine learning fundamentals, practical experience with real-world datasets, and the skills to build, evaluate, and deploy machine learning models. Join us on this exciting journey and transform your curiosity into capability, ready to tackle real-world challenges with machine learning. Welcome aboard!
Course Curriculum
Chapter 1: Overview of Machine Learning
Lecture 1: Introduction
Lecture 2: What is Machine Learning?
Lecture 3: Supervised Learning
Lecture 4: Unsupervised learning
Lecture 5: Reinforcement learning
Lecture 6: What is an Algorithm
Lecture 7: Introduction to Python for Data Science
Lecture 8: What is Pandas
Chapter 2: Setting Up Your Machine Learning Environment
Lecture 1: Python Installation on Windows
Lecture 2: What are virtual environments
Lecture 3: Creating and activating a virtual environment on Windows
Lecture 4: Python Installation on macOS
Lecture 5: Creating and activating a virtual environment on macOS
Lecture 6: What is Jupyter Notebook
Lecture 7: Installing Pandas and Jupyter Notebook in the Virtual Environment
Lecture 8: Starting Jupyter Notebook
Lecture 9: Exploring Jupyter Notebook Server Dashboard Interface
Lecture 10: Creating a new Notebook
Lecture 11: Exploring Jupyter Notebook Source and Folder Files
Lecture 12: Exploring the Notebook Interface
Lecture 13: Installing and importing libraries
Chapter 3: Data Preprocessing
Lecture 1: What is a Dataset
Lecture 2: Loading the dataset
Lecture 3: Exploring the Dataset
Lecture 4: Handle missing values and drop unnecessary columns.
Lecture 5: Encode categorical variables.
Lecture 6: What is Feature Engineering
Lecture 7: Create new features.
Lecture 8: Dropping unnecessary columns
Chapter 4: Data Visualization
Lecture 1: Visualize survival rate by gender
Lecture 2: Visualize survival rate by class
Lecture 3: Visualize numerical features
Lecture 4: Visualize the distribution of Age
Lecture 5: Visualize number of passengers in each passenger class
Lecture 6: Visualize number of passengers that survived
Lecture 7: Visualize the correlation matrix of numerical variables
Lecture 8: Visualize the distribution of Fare.
Chapter 5: Data Preparation and Model Training
Lecture 1: What is a Model
Lecture 2: What does training a model mean ?
Lecture 3: Define features and target variable.
Lecture 4: Split data into training and testing sets.
Lecture 5: Standardize features.
Lecture 6: What is a logistic regression model.
Lecture 7: Train logistic regression model.
Lecture 8: Making Predictions
Chapter 6: Model Evaluation
Lecture 1: What is accuracy in machine learning
Lecture 2: What is confusion matrix.
Lecture 3: What is is classification report.
Lecture 4: What is a Heatmap
Lecture 5: Evaluate the model using accuracy, confusion matrix, and classification report.
Lecture 6: Visualize the confusion matrix.
Chapter 7: Saving and Loading Model
Lecture 1: Saving the Model
Lecture 2: Loading the model
Lecture 3: Improving Understanding of the model's prediction
Chapter 8: Predicting real house prices using machine learning
Lecture 1: Importing Libraries and modules
Lecture 2: Loading dataset and creating a dataframe
Lecture 3: Checking for missing values
Lecture 4: Dropping column and splitting data
Lecture 5: Standardize the features for housing dataframe
Lecture 6: Initialize and train the regression model
Lecture 7: Make predictions on the test set.
Lecture 8: Evaluating the model for the housing dataset.
Lecture 9: Predicting a small sample of data
Lecture 10: Creating scatter plot
Lecture 11: Creating a bar plot
Lecture 12: Saving the housing model
Lecture 13: Loading the housing model
Chapter 9: Create a House Price Prediction Tool
Lecture 1: What is Flask
Lecture 2: Installing Flask
Lecture 3: Installing Visual Studio Code
Lecture 4: Creating a minimal flask app
Lecture 5: How to run a flask app
Lecture 6: Http and Http Methods
Lecture 7: Loading the saved model and scaler into Python file
Lecture 8: Define the home route
Lecture 9: Define the prediction route
Lecture 10: Creating the template
Lecture 11: Adding a form to the template
Lecture 12: Displaying predictions and clearing form inputs
Lecture 13: Testing the prediction tool
Lecture 14: Exploring deployment and hosting options
Lecture 15: Create a new account on pythonanywhere
Lecture 16: Creating a new web app in Pythonanywhere
Lecture 17: Uploading project files to Pythonanywhere
Lecture 18: Creating and activating a virtual environment on Pythonanywhere
Lecture 19: What is a WSGI File
Lecture 20: Configuring WSGI File
Lecture 21: Running your app in a cloud hosting environment
Lecture 22: Project files
Chapter 10: Decision Trees and Random Forest
Lecture 1: Building a decision tree
Instructors
-
Bluelime Learning Solutions
Making Learning Simple
Rating Distribution
- 1 stars: 0 votes
- 2 stars: 0 votes
- 3 stars: 0 votes
- 4 stars: 1 votes
- 5 stars: 1 votes
Frequently Asked Questions
How long do I have access to the course materials?
You can view and review the lecture materials indefinitely, like an on-demand channel.
Can I take my courses with me wherever I go?
Definitely! If you have an internet connection, courses on Udemy are available on any device at any time. If you don’t have an internet connection, some instructors also let their students download course lectures. That’s up to the instructor though, so make sure you get on their good side!
You may also like
- Digital Marketing Foundation Course
- Google Shopping Ads Digital Marketing Course
- Multi Cloud Infrastructure for beginners
- Master Lead Generation: Grow Subscribers & Sales with Popups
- Complete Copywriting System : write to sell with ease
- Product Positioning Masterclass: Unlock Market Traction
- How to Promote Your Webinar and Get More Attendees?
- Digital Marketing Courses
- Create music with Artificial Intelligence in this new market
- Create CONVERTING UGC Content So Brands Will Pay You More
- Podcast: The top 8 ways to monetize by Podcasting
- TikTok Marketing Mastery: Learn to Grow & Go Viral
- Free Digital Marketing Basics Course in Hindi
- MailChimp Free Mailing Lists: MailChimp Email Marketing
- Automate Digital Marketing & Social Media with Generative AI
- Google Ads MasterClass – All Advanced Features
- Online Course Creator: Create & Sell Online Courses Today!
- Introduction to SEO – Basic Principles of SEO
- Affiliate Marketing For Beginners: Go From Novice To Pro
- Effective Website Planning Made Simple