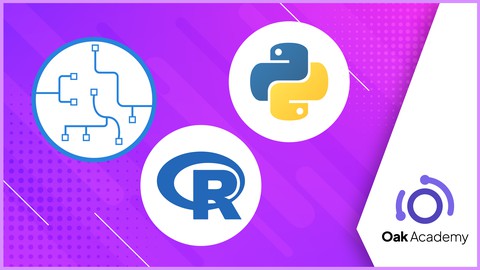
Complete Python Data Science, Deep Learning, R Programming
Complete Python Data Science, Deep Learning, R Programming, available at $19.99, has an average rating of 4.3, with 134 lectures, 15 quizzes, based on 28 reviews, and has 2447 subscribers.
You will learn about Python, Python Data science, Machine learning, Deep Learning, R, Numpy, Pandas, Matplotlib Python instructors on Udemy specialize in everything from software development to data analysis, and are known for their effective, friendly instruction Fundamental stuff of Python and its library Numpy What is the AI, Machine Learning and Deep Learning History of Machine Learning Turing Machine and Turing Test The Logic of Machine Learning such as Machine Learning models and algorithms, Gathering data, Data pre-processing, Training and testing the model etc. What is Artificial Neural Network (ANN) Anatomy of NN Tensor Operations Machine learning is constantly being applied to new industries and new problems. Whether you’re a marketer, video game designer, or programmer, I am here to hel Machine learning isn’t just useful for predictive texting or smartphone voice recognition. The Engine of NN Keras Tensorflow Convolutional Neural Network Recurrent Neural Network and LTSM Transfer Learning Machine Learning Deep Learning Machine Learning with Python Python Programming Deep Learning with Python If you have some programming experience, Python might be the language for you Learn Fundamentals of Python for effectively using Data Science Data Manipulation Learn how to handle with big data Learn how to manipulate the data Learn how to produce meaningful outcomes Learn Fundamentals of Python for effectively using Data Science Numpy arrays Series and Features Combining Dataframes, Data Munging and how to deal with Missing Data How to use Matplotlib library and start to journey in Data Visualization Also, why you should learn Python and Pandas Library Learn Data Science with Python Examine and manage data structures Handle wide variety of data science challenges Select columns and filter rows Arrange the order and create new variables Create, subset, convert or change any element within a vector or data frame Transform and manipulate an existing and real data The Logic of Matplotlib What is Matplotlib Using Matplotlib Pyplot – Pylab – Matplotlib Figure, Subplot, Multiplot, Axes, Figure Customization Data Visualization Plot Customization Grid, Spines, Ticks Basic Plots in Matplotlib Seaborn library with these topics What is Seaborn Controlling Figure Aesthetics Color Palettes Basic Plots in Seaborn Multi-Plots in Seaborn Regression Plots and Squarify Geoplotlib with these topics What is Geoplotlib Tile Providers and Custom Layers R and Python in the same course. You decide which one you would go for! R was built as a statistical language, it suits much better to do statistical learning and R is a statistical programming software favoured by many academia Since R was built as a statistical language, it suits much better to do statistical learning. You will learn R and Python from scratch Because data can mean an endless number of things, it’s important to choose the right visualization tools for the job. you’re interested in learning Tableau, D3 js, After Effects, or Python, has a course for you. Learn how to use NumPy, Pandas, Seaborn , Matplotlib , Machine Learning, and more! What is Python? Python is a general-purpose, object-oriented, high-level programming language. Python vs. R: what is the Difference? Python and R are two of today's most popular programming tools. What does it mean that Python is object-oriented? Python is a multi-paradigm language, which means that it supports many programming approaches. What are the limitations of Python? Python is a widely used, general-purpose programming language, but it has some limitations. How is Python used? Python is a general programming language used widely across many industries and platforms. What is data science? We have more data than ever before. But data alone cannot tell us much about the world around us. What does a data scientist do? Data Scientists use machine learning to discover hidden patterns in large amounts of raw data to shed light on real problems. What are the most popular coding languages for data science? Python is the most popular programming language for data science. Python, on the other hand, is a better choice for machine learning with its flexibility for production It represents the way statisticians think pretty well, so anyone with a formal statistics background can use R easily. Python Data Science, Python deep learning, machine learning This course is ideal for individuals who are Anyone interested in data sciences or Anyone who plans a career in data scientist, or Software developer whom want to learn data science, or Anyone eager to learn Data Science with no coding background or Statisticians, academic researchers, economists, analysts and business people or Professionals working in analytics or related fields or Anyone who is particularly interested in big data, machine learning and data intelligence or People wwho want to learn python data science, deep learning, R programming, machine learning It is particularly useful for Anyone interested in data sciences or Anyone who plans a career in data scientist, or Software developer whom want to learn data science, or Anyone eager to learn Data Science with no coding background or Statisticians, academic researchers, economists, analysts and business people or Professionals working in analytics or related fields or Anyone who is particularly interested in big data, machine learning and data intelligence or People wwho want to learn python data science, deep learning, R programming, machine learning.
Enroll now: Complete Python Data Science, Deep Learning, R Programming
Summary
Title: Complete Python Data Science, Deep Learning, R Programming
Price: $19.99
Average Rating: 4.3
Number of Lectures: 134
Number of Quizzes: 15
Number of Published Lectures: 134
Number of Published Quizzes: 15
Number of Curriculum Items: 149
Number of Published Curriculum Objects: 149
Original Price: $199.99
Quality Status: approved
Status: Live
What You Will Learn
- Python, Python Data science, Machine learning, Deep Learning, R, Numpy, Pandas, Matplotlib
- Python instructors on Udemy specialize in everything from software development to data analysis, and are known for their effective, friendly instruction
- Fundamental stuff of Python and its library Numpy
- What is the AI, Machine Learning and Deep Learning
- History of Machine Learning
- Turing Machine and Turing Test
- The Logic of Machine Learning such as Machine Learning models and algorithms, Gathering data, Data pre-processing, Training and testing the model etc.
- What is Artificial Neural Network (ANN)
- Anatomy of NN
- Tensor Operations
- Machine learning is constantly being applied to new industries and new problems. Whether you’re a marketer, video game designer, or programmer, I am here to hel
- Machine learning isn’t just useful for predictive texting or smartphone voice recognition.
- The Engine of NN
- Keras
- Tensorflow
- Convolutional Neural Network
- Recurrent Neural Network and LTSM
- Transfer Learning
- Machine Learning
- Deep Learning
- Machine Learning with Python
- Python Programming
- Deep Learning with Python
- If you have some programming experience, Python might be the language for you
- Learn Fundamentals of Python for effectively using Data Science
- Data Manipulation
- Learn how to handle with big data
- Learn how to manipulate the data
- Learn how to produce meaningful outcomes
- Learn Fundamentals of Python for effectively using Data Science
- Numpy arrays
- Series and Features
- Combining Dataframes, Data Munging and how to deal with Missing Data
- How to use Matplotlib library and start to journey in Data Visualization
- Also, why you should learn Python and Pandas Library
- Learn Data Science with Python
- Examine and manage data structures
- Handle wide variety of data science challenges
- Select columns and filter rows
- Arrange the order and create new variables
- Create, subset, convert or change any element within a vector or data frame
- Transform and manipulate an existing and real data
- The Logic of Matplotlib
- What is Matplotlib
- Using Matplotlib
- Pyplot – Pylab – Matplotlib
- Figure, Subplot, Multiplot, Axes,
- Figure Customization
- Data Visualization
- Plot Customization
- Grid, Spines, Ticks
- Basic Plots in Matplotlib
- Seaborn library with these topics
- What is Seaborn
- Controlling Figure Aesthetics
- Color Palettes
- Basic Plots in Seaborn
- Multi-Plots in Seaborn
- Regression Plots and Squarify
- Geoplotlib with these topics
- What is Geoplotlib
- Tile Providers and Custom Layers
- R and Python in the same course. You decide which one you would go for!
- R was built as a statistical language, it suits much better to do statistical learning and R is a statistical programming software favoured by many academia
- Since R was built as a statistical language, it suits much better to do statistical learning.
- You will learn R and Python from scratch
- Because data can mean an endless number of things, it’s important to choose the right visualization tools for the job.
- you’re interested in learning Tableau, D3 js, After Effects, or Python, has a course for you.
- Learn how to use NumPy, Pandas, Seaborn , Matplotlib , Machine Learning, and more!
- What is Python? Python is a general-purpose, object-oriented, high-level programming language.
- Python vs. R: what is the Difference? Python and R are two of today's most popular programming tools.
- What does it mean that Python is object-oriented? Python is a multi-paradigm language, which means that it supports many programming approaches.
- What are the limitations of Python? Python is a widely used, general-purpose programming language, but it has some limitations.
- How is Python used? Python is a general programming language used widely across many industries and platforms.
- What is data science? We have more data than ever before. But data alone cannot tell us much about the world around us.
- What does a data scientist do? Data Scientists use machine learning to discover hidden patterns in large amounts of raw data to shed light on real problems.
- What are the most popular coding languages for data science? Python is the most popular programming language for data science.
- Python, on the other hand, is a better choice for machine learning with its flexibility for production
- It represents the way statisticians think pretty well, so anyone with a formal statistics background can use R easily.
- Python Data Science, Python deep learning, machine learning
Who Should Attend
- Anyone interested in data sciences
- Anyone who plans a career in data scientist,
- Software developer whom want to learn data science,
- Anyone eager to learn Data Science with no coding background
- Statisticians, academic researchers, economists, analysts and business people
- Professionals working in analytics or related fields
- Anyone who is particularly interested in big data, machine learning and data intelligence
- People wwho want to learn python data science, deep learning, R programming, machine learning
Target Audiences
- Anyone interested in data sciences
- Anyone who plans a career in data scientist,
- Software developer whom want to learn data science,
- Anyone eager to learn Data Science with no coding background
- Statisticians, academic researchers, economists, analysts and business people
- Professionals working in analytics or related fields
- Anyone who is particularly interested in big data, machine learning and data intelligence
- People wwho want to learn python data science, deep learning, R programming, machine learning
Welcome to Complete Python Data Science, Deep Learning, R Programmingcourse.
Python Data Science A-Z, Data Science with Machine Learning A-Z, Deep Learning A-Z, Pandas, Numpy and R Statistics
Data science, python data science, r statistics, machine learning, deep learning, data visualization, NumPy, pandas, data science with r, r, complete data science, maths for data science, data science a-z
Data Science A-Z, Python Data Science with Machine Learning, Deep Learning, Pandas, Numpy, Data visualization, and R
Ready for the Data Science career?
-
Are you curious about Data Science and looking to start your self-learning journey into the world of data?
-
Are you an experienced developer looking for a landing in Data Science!
In both cases, you are at the right place!
The two most popular programming tools for data science work are Python and R at the moment. It is hard to pick one out of those two amazingly flexible data analytics languages. Both are free and open-source.
Train up with a top-rated data science course on Udemy. Gain in-demand skills and help organizations forecast product and service demands for the future. From machine learning to data mining to data analysis, we’ve got a data science course to help you progress on your career path.
R for statistical analysis and Python as a general-purpose programming language. For anyone interested in machine learning, working with large datasets, or creating complex data visualizations, they are absolutely essential.
With my full-stack Data Science course, you will be able to learn R and Python together.
If you have some programming experience, Python might be the language for you. R was built as a statistical language, it suits much better to do statistical learning with R programming.
But do not worry! In this course, you will have a chance to learn both and will decide which one fits your niche!
Throughout the course’s first part, you will learn the most important tools in R that will allow you to do data science. By using the tools, you will be easily handling big data, manipulating it, and producing meaningful outcomes.
Throughout the course’s second part, we will teach you how to use Python to analyze data, create beautiful visualizations, and use powerful machine learning algorithms and we will also do a variety of exercises to reinforce what we have learned in this Python for Data Science course.
We will open the door of the Data Science world and will move deeper. You will learn the fundamentals of Python and its beautiful libraries such as Numpy, Pandas, and Matplotlib step by step. Then, we will transform and manipulate real data. For the manipulation, we will use the tidyverse package, which involves dplyr and other necessary packages.
At the end of the course, you will be able to select columns, filter rows, arrange the order, create new variables, group by and summarize your data simultaneously.
Because data can mean an endless number of things, it’s important to choose the right visualization tools for the job. Whether you’re interested in learning Tableau, D3.js, After Effects, or Python, Udemy has a course for you.
In this course, we will learn what is data visualization and how does it work with python.
This course has suitable for everybody who is interested data visualization concept.
First of all, in this course, we will learn some fundamentals of pyhton, and object oriented programming ( OOP ). These are our first steps in our Data Visualisation journey. After then we take a our journey to Data Science world. Here we will take a look data literacy and data science concept. Then we will arrive at our next stop. Numpy library. Here we learn the what is numpy and how we can use it. After then we arrive at our next stop. Pandas library. And now our journey becomes an adventure. In this adventure we’ll enter the Matplotlib world then we exit the Seaborn world. Then we’ll try to understand how we can visualize our data, data viz. But our journey won’t be over. Then we will arrive our final destination. Geographical drawing or best known as Geoplotlib in tableau data visualization.
Learn python and how to use it to python data analysis and visualization, present data. Includes tons of code data vizualisation.
In this course, you will learn data analysis and visualization in detail.
Also during the course you will learn:
The Logic of Matplotlib
-
What is Matplotlib
-
Using Matplotlib
-
Pyplot – Pylab – Matplotlib – Excel
-
Figure, Subplot, Multiplot, Axes,
-
Figure Customization
-
Plot Customization
-
Grid, Spines, Ticks
-
Basic Plots in Matplotlib
-
Overview of Jupyter Notebook and Google Colab
-
Seaborn library with these topics
-
What is Seaborn
-
Controlling Figure Aesthetics
-
Color Palettes
-
Basic Plots in Seaborn
-
Multi-Plots in Seaborn
-
Regression Plots and Squarify
-
-
Geoplotlib with these topics
-
What is Geoplotlib
-
Tile Providers and Custom Layers
-
In this course you will learn;
-
How to use Anaconda and Jupyter notebook,
-
Fundamentals of Python such as
-
Datatypes in Python,
-
Lots of datatype operators, methods and how to use them,
-
Conditional concept, if statements
-
The logic of Loops and control statements
-
Functions and how to use them
-
How to use modules and create your own modules
-
Data science and Data literacy concepts
-
Fundamentals of Numpy for Data manipulation such as
-
Numpy arrays and their features
-
How to do indexing and slicing on Arrays
-
Lots of stuff about Pandas for data manipulation such as
-
Pandas series and their features
-
Dataframes and their features
-
Hierarchical indexing concept and theory
-
Groupby operations
-
The logic of Data Munging
-
How to deal effectively with missing data effectively
-
Combining the Data Frames
-
How to work with Dataset files
-
And also you will learn fundamentals thing about Matplotlib library such as
-
Pyplot, Pylab and Matplotlb concepts
-
What Figure, Subplot and Axes are
-
How to do figure and plot customization
-
Examining and Managing Data Structures in R
-
Atomic vectors
-
Lists
-
Arrays
-
Matrices
-
Data frames
-
Tibbles
-
Factors
-
Data Transformation in R
-
Transform and manipulate a deal data
-
Tidyverse and more
-
Python
-
Python data science
-
R, r programming
-
r statistics
-
machine learning
-
deep learning
-
numpy
-
pandas
This course has suitable for everybody who interested in Machine Learning and Deep Learning concepts in Data Science.
First of all, in this course, we will learn some fundamental stuff of Python and the Numpy library. These are our first steps in our Deep Learning journey. After then we take a little trip to Machine Learning Python history. Then we will arrive at our next stop. Machine Learning in Python Programming. Here we learn the machine learning concepts, machine learning a-z workflow, models and algorithms, and what is neural network concept. After then we arrive at our next stop. Artificial Neural network. And now our journey becomes an adventure. In this adventure we’ll enter the Keras world then we exit the Tensorflow world. Then we’ll try to understand the Convolutional Neural Network concept. But our journey won’t be over. Then we will arrive at Recurrent Neural Network and LTSM. We’ll take a look at them. After a while, we’ll trip to the Transfer Learning concept. And then we arrive at our final destination. Projects in Python Bootcamp. Our play garden. Here we’ll make some interesting machine learning models with the information we’ve learned along our journey.
In this course, we will start from the very beginning and go all the way to the end of “Deep Learning” with examples.
The Logic of Machine Learning such as Machine Learning models and algorithms, Gathering data, Data pre-processing, Training and testing the model etc.
Before we start this course, we will learn which environments we can be used for developing deep learning projects.
-
Artificial Neural Network with these topics
-
What is ANN
-
Anatomy of NN
-
Tensor Operations
-
The Engine of NN
-
Keras
-
Tensorflow
-
-
Convolutional Neural Network
-
Recurrent Neural Network and LTSM
-
Transfer Learning
-
Reinforcement Learning
And we will do many exercises. Finally, we will also have 4 different final projects covering all of Python subjects.
What is data science?
We have more data than ever before. But data alone cannot tell us much about the world around us. We need to interpret the information and discover hidden patterns. This is where data science comes in. Data science uses algorithms to understand raw data. The main difference between data science and traditional data analysis is its focus on prediction. Data science seeks to find patterns in data and use those patterns to predict future data. It draws on machine learning to process large amounts of data, discover patterns, and predict trends. Data science includes preparing, analyzing, and processing data. It draws from many scientific fields, and as a science, it progresses by creating new algorithms to analyze data and validate current methods.
What does a data scientist do?
Data Scientists use machine learning to discover hidden patterns in large amounts of raw data to shed light on real problems. This requires several steps. First, they must identify a suitable problem. Next, they determine what data are needed to solve such a situation and figure out how to get the data. Once they obtain the data, they need to clean the data. The data may not be formatted correctly, it might have additional unnecessary data, it might be missing entries, or some data might be incorrect. Data Scientists must, therefore, make sure the data is clean before they analyze the data. To analyze the data, they use machine learning techniques to build models. Once they create a model, they test, refine, and finally put it into production.
What are the most popular coding languages for data science?
Python is the most popular programming language for data science. It is a universal language that has a lot of libraries available. It is also a good beginner language. R is also popular; however, it is more complex and designed for statistical analysis. It might be a good choice if you want to specialize in statistical analysis. You will want to know either Python or R and SQL. SQL is a query language designed for relational databases. Data scientists deal with large amounts of data, and they store a lot of that data in relational databases. Those are the three most-used programming languages. Other languages such as Java, C++, JavaScript, and Scala are also used, albeit less so. If you already have a background in those languages, you can explore the tools available in those languages. However, if you already know another programming language, you will likely be able to pick up Python very quickly.
How long does it take to become a data scientist?
This answer, of course, varies. The more time you devote to learning new skills, the faster you will learn. It will also depend on your starting place. If you already have a strong base in mathematics and statistics, you will have less to learn. If you have no background in statistics or advanced mathematics, you can still become a data scientist; it will just take a bit longer. Data science requires lifelong learning, so you will never really finish learning. A better question might be, “How can I gauge whether I know enough to become a data scientist?” Challenge yourself to complete data science projects using open data. The more you practice, the more you will learn, and the more confident you will become. Once you have several projects that you can point to as good examples of your skillset as a data scientist, you are ready to enter the field.
What is R and why is it useful?
The R programming language was created specifically for statistical programming. Many find it useful for data handling, cleaning, analysis, and representation. R is also a popular language for data science projects. Much of the data used for data science can be messy and complex. The programming language has features and libraries available geared toward cleaning up unorganized data and making complex data structures easier to handle that can’t be found in other languages. It also provides powerful data visualization tools to help data scientists find patterns in large sets of data and present the results in expressive reports. Machine learning is another area where the R language is useful. R gives developers an extensive selection of machine learning libraries that will help them find trends in data and predict future events.
What careers use R?
R is a popular programming language for data science, business intelligence, and financial analysis. Academic, scientific, and non-profit researchers use the R language to glean answers from data. R is also widely used in market research and advertising to analyze the results of marketing campaigns and user data. The language is used in quantitative analysis, where its data analysis capabilities give financial experts the tools they need to manage portfolios of stocks, bonds, and other assets. Data scientists use R in many industries to turn data into insights and predict future trends with its machine learning capabilities. Data analysts use R to extract data, analyze it, and turn it into reports that can help enterprises make better business decisions. Data visualization experts use R to turn data into visually appealing graphs and charts.
Is R difficult to learn?
Whether R is hard to learn depends on your experience. After all, R is a programming language designed for mathematicians, statisticians, and business analysts who may have no coding experience. For some beginning users, it is relatively simple to learn R. It can have a learning curve if you are a business analyst who is only familiar with graphical user interfaces since R is a text-based programming language. But compared to other programming languages, users usually find R easier to understand. R also may have an unfamiliar syntax for programmers who are used to other programming languages, but once they learn the syntax, the learning process becomes more straightforward. Beginners will also find that having some knowledge of mathematics, statistics, and probabilities makes learning R easier.
Python vs. R: What is the Difference?
Python and R are two of today’s most popular programming tools. When deciding between Python and R, you need to think about your specific needs. On one hand, Python is relatively easy for beginners to learn, is applicable across many disciplines, has a strict syntax that will help you become a better coder, and is fast to process large datasets. On the other hand, R has over 10,000 packages for data manipulation, is capable of easily making publication-quality graphics, boasts superior capability for statistical modeling, and is more widely used in academia, healthcare, and finance.
What is Python?
Python is a general-purpose, object-oriented, high-level programming language. Whether you work in artificial intelligence or finance or are pursuing a career in web development or data science, Python is one of the most important skills you can learn. Python’s simple syntax is especially suited for desktop, web, and business applications. Python’s design philosophy emphasizes readability and usability. Python was developed on the premise that there should be only one way (and preferably, one obvious way) to do things, a philosophy that resulted in a strict level of code standardization. The core programming language is quite small and the standard library is also large. In fact, Python’s large library is one of its greatest benefits, providing different tools for programmers suited for a variety of tasks.
Python vs. R: what is the Difference?
Python and R are two of today’s most popular programming tools. When deciding between Python and R, you need to think about your specific needs. On one hand, Python is relatively easy for beginners to learn, is applicable across many disciplines, has a strict syntax that will help you become a better coder, and is fast to process large datasets. On the other hand, R has over 10,000 packages for data manipulation, is capable of easily making publication-quality graphics, boasts superior capability for statistical modeling, and is more widely used in academia, healthcare, and finance.
What does it mean that Python is object-oriented?
Python is a multi-paradigm language, which means that it supports many programming approaches. Along with procedural and functional programming styles, Python also supports the object-oriented style of programming. In object-oriented programming, a developer completes a programming project by creating Python objects in code that represent objects in the actual world. These objects can contain both the data and functionality of the real-world object. To generate an object in Python you need a class. You can think of a class as a template. You create the template once, and then use the template to create as many objects as you need. Python classes have attributes to represent data and methods that add functionality. A class representing a car may have attributes like color, speed, and seats and methods like driving, steering, and stopping. The concept of combining data with functionality in an object is called encapsulation, a core concept in the object-oriented programming paradigm.
I am glad that I took this course. There was always something to learn in every lesson. The Jupyter notebooks provided are very helpful. The two milestone projects and the final capstone project helped me gain a lot of confidence. Moreover, there were short challenges, assignments, and quizzes which also helped a lot.
This course’s approach is very practical and easy to understand, especially for beginners. Thank you for the excellent course.
Why would you want to take this course?
Our answer is simple: The quality of teaching.
When you enroll, you will feel the OAK Academy’s seasoned instructors’ expertise.
Fresh Content
It’s no secret how technology is advancing at a rapid rate and it’s crucial to stay on top of the latest knowledge. With this course, you will always have a chance to follow the latest data science trends.
Video and Audio Production Quality
All our content is created/produced as high-quality video/audio to provide you the best learning experience.
You will be,
-
Seeing clearly
-
Hearing clearly
-
Moving through the course without distractions
You’ll also get:
-
Lifetime Access to The Course
-
Fast & Friendly Support in the Q&A section
-
Udemy Certificate of Completion Ready for Download
Dive in now!
Complete Python Data Science, Deep Learning, R Programming
We offer full support, answering any questions.
See you in the course!
Course Curriculum
Chapter 1: Introduction to Complete Data Science, Deep Learning, R | Data Science 2021
Lecture 1: Be Smart and Use Data But How: Answer is Data Science with Python
Lecture 2: Project Files and Course Documents: Data Science, Python data science
Lecture 3: FAQ about Complete data science with R, deep learning, machine learning
Chapter 2: Setting Up Python for Mac and Windows: Python Data Science
Lecture 1: Installing Anaconda for Windows
Lecture 2: Installing Anaconda for Mac, Python Data Science
Lecture 3: Let's Meet Jupyter Notebook for Windows
Lecture 4: Basics of Jupyter Notebook for Mac
Chapter 3: Fundamentals of Python
Lecture 1: Data Types in Python
Lecture 2: Operators in Python
Lecture 3: Conditionals in Python
Lecture 4: Loops in Python
Lecture 5: Lists, Tuples, Dictionaries and Sets in Python
Lecture 6: Data Type Operators and Methods in Python
Lecture 7: Modules in Python
Lecture 8: Functions in Python
Lecture 9: Exercise Analyse in Python
Lecture 10: Exercise Solution in Python
Chapter 4: Object Oriented Programming
Lecture 1: Logic of Object Oriented Programming
Lecture 2: Constructor in Object Oriented Programming
Lecture 3: Methods in Object Oriented Programming
Lecture 4: Inheritance in Object Oriented Programming
Lecture 5: Overriding and Overloading in OOP
Chapter 5: Python For Data Science: Data Science
Lecture 1: What Is Data Science?
Lecture 2: Data Literacy
Chapter 6: Using Numpy for Data Manipulation
Lecture 1: What is Numpy?
Lecture 2: Array and Features in Numpy Python
Lecture 3: Array Operators in Numpy
Lecture 4: Indexing and Slicing in Numpy Python
Lecture 5: Numpy Exercises
Chapter 7: Pandas: Using Pandas for Data Manipulation
Lecture 1: What is Pandas?
Lecture 2: Series and Features
Chapter 8: Data Frame with Pandas
Lecture 1: Data Frame Attributes and Methods
Lecture 2: Data Frame Attributes and Methods Part – II
Lecture 3: Data Frame Attributes and Methods Part – III
Lecture 4: Multi Index in Pandas
Lecture 5: Groupby Operations in Pandas
Lecture 6: Missing Data and Data Munging in Pandas
Lecture 7: Missing Data and Data Munging Part II
Lecture 8: How We Deal with Missing Data?
Lecture 9: Combining Data Frames in Pandas
Lecture 10: Combining Data Frames Part – II
Lecture 11: Work with Dataset Files in Pandas
Chapter 9: Matplotlib
Lecture 1: What is Matplotlib
Lecture 2: Using Matplotlib
Lecture 3: Pyplot – Pylab – Matplotlib
Lecture 4: Figure, Subplot and Axes in Matplotlib
Lecture 5: Figure Customization in Matplotlib
Lecture 6: Plot Customization in matplotlib
Lecture 7: Grid, Spines, Ticks in python
Lecture 8: Basic Plots in Matplotlib I
Lecture 9: Basic Plots in Matplotlib II
Chapter 10: Seaborn
Lecture 1: What is Seaborn?
Lecture 2: Controlling Figure Aesthetics in Seaborn
Lecture 3: Example in Seaborn
Lecture 4: Color Palettes in Seaborn
Lecture 5: Basic Plots in Seaborn
Lecture 6: Multi-Plots in Seaborn
Lecture 7: Regression Plots and Squarify in Seaborn
Chapter 11: Geoplotlib
Lecture 1: What is Geoplotlib?
Lecture 2: Example – 1
Lecture 3: Example – 2
Lecture 4: Example – 3
Chapter 12: Data Science: Hands-On Projects
Lecture 1: Analyse Data With Different Data Sets: Titanic Project
Lecture 2: Titanic Project Answers in python projects
Lecture 3: Project II: Bike Sharing
Lecture 4: Bike Sharing Project Answers
Lecture 5: Project III: Housing and Property Sales
Lecture 6: Answer for Housing and Property Sales Project
Lecture 7: Project IV: English Premier League
Lecture 8: Answers for English Premier League Project
Chapter 13: Machine Learning
Lecture 1: AI, Machine Learning and Deep Learning
Lecture 2: History of Machine Learning
Lecture 3: Turing Machine and Turing Test
Lecture 4: What is Deep Learning
Lecture 5: Learning representations from data
Lecture 6: Workflow of Machine Learning
Lecture 7: Machine Learning Methods
Lecture 8: Supervised Machine Learning Methods – 1
Lecture 9: Supervised Machine Learning Methods – 2
Instructors
-
Oak Academy
Web & Mobile Development, IOS, Android, Ethical Hacking, IT -
OAK Academy Team
instructor
Rating Distribution
- 1 stars: 0 votes
- 2 stars: 2 votes
- 3 stars: 1 votes
- 4 stars: 5 votes
- 5 stars: 20 votes
Frequently Asked Questions
How long do I have access to the course materials?
You can view and review the lecture materials indefinitely, like an on-demand channel.
Can I take my courses with me wherever I go?
Definitely! If you have an internet connection, courses on Udemy are available on any device at any time. If you don’t have an internet connection, some instructors also let their students download course lectures. That’s up to the instructor though, so make sure you get on their good side!
You may also like
- Ultimate all royalty free contents for youtubers or bloggers
- Complete WhatsApp Marketing Course
- YouTube 1M Subscribers Guide – Proven ways for YouTube Subs
- Sales Funnel Blueprint For Beginners
- Instagram Business Marketing Game Plan 10k+ Paying Customers
- Earning Online Made Easy: CPA Marketing for Beginners
- MarketHealth Mastery: Dominating Sales in 90 Days
- How to Use Instagram Video for Business and Building a Brand
- YouTube Piggyback Method – Unlimited Cheap Traffic
- Learning Search Engine Optimization (SEO) from Scratch
- Rock Your Newsletter
- Google My Business – Complete Listing Optimization Training
- Workflow in Salesforce
- Mastering Invideo AI – A Complete Guide to Text-to-Video
- Ultimate Content Marketing Workshop ⎢Certificate Course 2024
- Copywriting For Beginners Course- Start Making Money Today
- Mastering Facebook Ads: From Beginner to Expert
- Content Creation with Midjourney
- Search Engine Marketing (SEM): #1 Step-by-Step Tutorial
- The Sales Funnel Cheat Sheet Collection – 4 Courses In 1