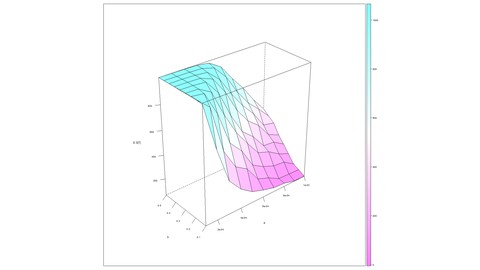
R Programming for Simulation and Monte Carlo Methods
R Programming for Simulation and Monte Carlo Methods, available at $64.99, has an average rating of 3.7, with 107 lectures, based on 448 reviews, and has 4859 subscribers.
You will learn about Use R software to program probabilistic simulations, often called Monte Carlo simulations. Use R software to program mathematical simulations and to create novel mathematical simulation functions. Use existing R functions and understand how to write their own R functions to perform simulated inference estimates, including likelihoods and confidence intervals, and to model other cases of stochastic simulation. Be able to generate different different families (and moments) of both discrete and continuous random variables. Be able to simulate parameter estimation, Monte-Carlo Integration of both continuous and discrete functions, and variance reduction techniques. This course is ideal for individuals who are You do NOT need to be experienced with R software and you do NOT need to be an experienced programmer. or Course is good for practicing quantitative analysis professionals. or Course is good for graduate students seeking research data and scenario analysis skills. or Anyone interested in learning more about programming statistical applications with R software would benefit from this course. It is particularly useful for You do NOT need to be experienced with R software and you do NOT need to be an experienced programmer. or Course is good for practicing quantitative analysis professionals. or Course is good for graduate students seeking research data and scenario analysis skills. or Anyone interested in learning more about programming statistical applications with R software would benefit from this course.
Enroll now: R Programming for Simulation and Monte Carlo Methods
Summary
Title: R Programming for Simulation and Monte Carlo Methods
Price: $64.99
Average Rating: 3.7
Number of Lectures: 107
Number of Published Lectures: 107
Number of Curriculum Items: 107
Number of Published Curriculum Objects: 107
Original Price: $159.99
Quality Status: approved
Status: Live
What You Will Learn
- Use R software to program probabilistic simulations, often called Monte Carlo simulations.
- Use R software to program mathematical simulations and to create novel mathematical simulation functions.
- Use existing R functions and understand how to write their own R functions to perform simulated inference estimates, including likelihoods and confidence intervals, and to model other cases of stochastic simulation.
- Be able to generate different different families (and moments) of both discrete and continuous random variables.
- Be able to simulate parameter estimation, Monte-Carlo Integration of both continuous and discrete functions, and variance reduction techniques.
Who Should Attend
- You do NOT need to be experienced with R software and you do NOT need to be an experienced programmer.
- Course is good for practicing quantitative analysis professionals.
- Course is good for graduate students seeking research data and scenario analysis skills.
- Anyone interested in learning more about programming statistical applications with R software would benefit from this course.
Target Audiences
- You do NOT need to be experienced with R software and you do NOT need to be an experienced programmer.
- Course is good for practicing quantitative analysis professionals.
- Course is good for graduate students seeking research data and scenario analysis skills.
- Anyone interested in learning more about programming statistical applications with R software would benefit from this course.
R Programming for Simulation and Monte Carlo Methods focuses on using R software to program probabilistic simulations, often called Monte Carlo Simulations. Typical simplified “real-world” examples include simulating the probabilities of a baseball player having a ‘streak’ of twenty sequential season games with ‘hits-at-bat’ or estimating the likely total number of taxicabs in a strange city when one observes a certain sequence of numbered cabs pass a particular street corner over a 60 minute period. In addition to detailing half a dozen (sometimes amusing) ‘real-world’ extended example applications, the course also explains in detail how to use existing R functions, and how to write your own R functions, to perform simulated inference estimates, including likelihoods and confidence intervals, and other cases of stochastic simulation. Techniques to use R to generate different characteristics of various families of random variables are explained in detail. The course teaches skills to implement various approaches to simulate continuous and discrete random variable probability distribution functions, parameter estimation, Monte-Carlo Integration, and variance reduction techniques. The course partially utilizes the Comprehensive R Archive Network (CRAN) spuRspackage to demonstrate how to structure and write programs to accomplish mathematical and probabilistic simulations using R statistical software.
Course Curriculum
Chapter 1: Review of Vectors, Matrices, Lists and Functions
Lecture 1: Course Introduction
Lecture 2: Install R and RStudio
Lecture 3: Review: Vectors, Matrices, Lists (part 1)
Lecture 4: Review: Vectors, Matrices, Lists (part 2)
Lecture 5: Sequences and Replications (part 1)
Lecture 6: Sort and Order
Lecture 7: Using Matrices (part 2)
Lecture 8: Sequences and Replications (part 2)
Lecture 9: Creating a Matrix (part 1)
Lecture 10: List Structures and Horsekicks (part 1)
Lecture 11: Dpois() Function and Horsekicks (part 2)
Lecture 12: Sampling from a Dataframe
Lecture 13: Section 1 Exercises
Chapter 2: Simulation Examples: Tossing a Coin
Lecture 1: R Expressions Exercises Answers (part 1)
Lecture 2: R Expressions Exercises Answers (part 2)
Lecture 3: Introduction to Simulation: A Game of Tossing a Coin (part 1)
Lecture 4: Introduction to Simulation: A Game of Tossing a Coin (part 2)
Lecture 5: Write a Simulation Function (part 1)
Lecture 6: Write a Simulation Function (part 2)
Lecture 7: Continue Coin Tossing Simulation (part 3)
Lecture 8: Continue Coin Tossing Simulation (part 4)
Chapter 3: Simulation Examples: Returning Checked Hats
Lecture 1: Random Permutations: Hat Problem (part 1)
Lecture 2: Random Permutations: Hat Problem (part 2 )
Lecture 3: Random Permutations: Hat Problem (part 3)
Lecture 4: Random Permutations: Hat Problem (part 4)
Lecture 5: Random Permutations: Hat Problem (part 5)
Lecture 6: Random Permutations: Hat Problem (part 6)
Lecture 7: Checking Hats Exercise
Chapter 4: Simulation Examples: Collecting Baseball Cards and "Streaky" Behavior
Lecture 1: Solution to Checking Hats Exercise
Lecture 2: Collecting Baseball Cards Simulation (part 1)
Lecture 3: Collecting Baseball Cards Simulation (part 2)
Lecture 4: Collecting Baseball Cards Simulation (part 3)
Lecture 5: Collecting Baseball Cards Simulation (part 4)
Lecture 6: Collecting Quarters Exercise
Lecture 7: Collecting State Quarters Exercise Solution
Lecture 8: "Streaky" Baseball Batting Behavior (part 1)
Lecture 9: "Streaky" Baseball Batting Behavior (part 2)
Lecture 10: "Streaky" Baseball Batting Behavior (part 3)
Lecture 11: "Streaky" Behavior Exercise
Chapter 5: Monte Carlo Methods for Inference
Lecture 1: Solution to "Streaky" Behavior Exercise
Lecture 2: Using Monte Carlo Simulation to Estimate Inference
Lecture 3: Sleepless in Seattle (part 1)
Lecture 4: Sleepless in Seattle (part 2)
Lecture 5: Applying Monte Carlo Methods to Inference (part 1)
Lecture 6: Applying Monte Carlo Methods to Inference (part 2)
Lecture 7: Applying Monte Carlo Methods to Inference (part 3)
Lecture 8: Applying Monte Carlo Methods to Inference (part 4)
Lecture 9: Applying Monte Carlo Methods to Inference (part 5)
Lecture 10: Comparing Estimators: The Taxi Problem (part 1)
Lecture 11: Comparing Estimators: The Taxi Problem (part 2)
Lecture 12: Late to Class Again ? Exercise
Chapter 6: Stochastic Simulation and Random Variable Generation
Lecture 1: Late to Class Again Exercise Solution
Lecture 2: What is Stochastic Simulation ?
Lecture 3: Simulation and Random Variable Generation (part 1)
Lecture 4: Simulation and Random Variable Generation (part 2)
Lecture 5: Simulation and Random Variable Generation (part 3)
Lecture 6: Simulating Discrete Random Variables (part 1)
Lecture 7: Simulating Discrete Random Variables (part 2)
Lecture 8: Simulating Discrete Random Variables (part 3)
Lecture 9: Root Finding: Newton-Raphson Technique (part 1)
Lecture 10: Root Finding: Newton-Raphson Technique (part 2)
Lecture 11: Create Random Variables Exercise
Chapter 7: Inverse and General Transforms
Lecture 1: Create Random Variables Exercise Solution (part 1)
Lecture 2: Create Random Variables Exercise Solution (part 2)
Lecture 3: Inverse Transforms (part 1)
Lecture 4: Inverse Transforms (part 2)
Lecture 5: General Transformations (part 1)
Lecture 6: General Transformations (part 2)
Lecture 7: Accept-Reject Method (part 1)
Lecture 8: Accept-Reject Method (part 2)
Lecture 9: Accept-Reject Methods (part 3)
Lecture 10: Random Variable (Poisson) Exercise 2
Chapter 8: Simulating Numerical Integration
Lecture 1: Random Variable Exercise Solution (part 1)
Lecture 2: Random Variable Exercise Solution (part 2)
Lecture 3: Introduction to Simulating Numerical Integration (part 1)
Lecture 4: Introduction to Simulating Numerical Integration (part 2)
Lecture 5: Simpson's Rule for Trapezoidal Approximation
Lecture 6: Simulating Numerical Integration (part 1)
Lecture 7: Simulating Numerical Integration (part 2)
Lecture 8: More on Simpson's Rule
Lecture 9: Simpson's Rule with phi Functions
Lecture 10: Phi Functions Exercises
Lecture 11: Hit and Miss (part 1)
Lecture 12: Hit and Miss (part 2)
Chapter 9: Permutation Tests
Lecture 1: Phi Functions (Numerical Integration) Exercise Solution
Lecture 2: Permutation Tests on a Distribution: Chckwts Example (part 1)
Lecture 3: Permutation Tests on a Distribution: Chckwts Example (part 2)
Lecture 4: Permutation Tests on a Distribution: Chckwts Example (part 3)
Lecture 5: Permutation Tests on a Distribution: Chckwts Example (part 4)
Lecture 6: Finish Permutation Tests and an Exercise
Chapter 10: Simulation Case Studies: Seed Dispersal
Instructors
-
Geoffrey Hubona, Ph.D.
Associate Professor of MIS and Data Analytics
Rating Distribution
- 1 stars: 14 votes
- 2 stars: 33 votes
- 3 stars: 72 votes
- 4 stars: 159 votes
- 5 stars: 170 votes
Frequently Asked Questions
How long do I have access to the course materials?
You can view and review the lecture materials indefinitely, like an on-demand channel.
Can I take my courses with me wherever I go?
Definitely! If you have an internet connection, courses on Udemy are available on any device at any time. If you don’t have an internet connection, some instructors also let their students download course lectures. That’s up to the instructor though, so make sure you get on their good side!
You may also like
- Best Emotional Intelligence Courses to Learn in March 2025
- Best Time Management Courses to Learn in March 2025
- Best Remote Work Strategies Courses to Learn in March 2025
- Best Freelancing Courses to Learn in March 2025
- Best E-commerce Strategies Courses to Learn in March 2025
- Best Personal Branding Courses to Learn in March 2025
- Best Stock Market Trading Courses to Learn in March 2025
- Best Real Estate Investing Courses to Learn in March 2025
- Best Financial Technology Courses to Learn in March 2025
- Best Agile Methodologies Courses to Learn in March 2025
- Best Project Management Courses to Learn in March 2025
- Best Leadership Skills Courses to Learn in March 2025
- Best Public Speaking Courses to Learn in March 2025
- Best Affiliate Marketing Courses to Learn in March 2025
- Best Email Marketing Courses to Learn in March 2025
- Best Social Media Management Courses to Learn in March 2025
- Best SEO Optimization Courses to Learn in March 2025
- Best Content Creation Courses to Learn in March 2025
- Best Game Development Courses to Learn in March 2025
- Best Software Testing Courses to Learn in March 2025