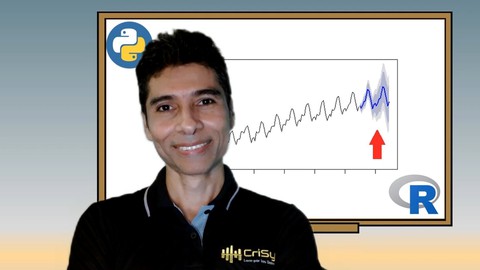
Time Series Analysis with Python and R
Time Series Analysis with Python and R, available at $19.99, has an average rating of 4.75, with 43 lectures, 2 quizzes, based on 6 reviews, and has 41 subscribers.
You will learn about Learn the basic statistical concepts and techniques used in time series analysis. Learn some basic statements to do a time series analysis using Python. Learn some basic statements to do a time series analysis using R. Explain in your own terms, how to perform a time series analysis. Identify the type of model to apply in a time series. This course is ideal for individuals who are Students who wish to acquire or improve their skills in data analysis through time series techniques. or Python developers who want to improve their skills using time series techniques. or Data Analysts. or Beginning python and r developers interested in data science. or Professionals in areas such as marketing, finance, retail, budget, production stock, and so forth. It is particularly useful for Students who wish to acquire or improve their skills in data analysis through time series techniques. or Python developers who want to improve their skills using time series techniques. or Data Analysts. or Beginning python and r developers interested in data science. or Professionals in areas such as marketing, finance, retail, budget, production stock, and so forth.
Enroll now: Time Series Analysis with Python and R
Summary
Title: Time Series Analysis with Python and R
Price: $19.99
Average Rating: 4.75
Number of Lectures: 43
Number of Quizzes: 2
Number of Published Lectures: 43
Number of Published Quizzes: 2
Number of Curriculum Items: 45
Number of Published Curriculum Objects: 45
Original Price: $19.99
Quality Status: approved
Status: Live
What You Will Learn
- Learn the basic statistical concepts and techniques used in time series analysis.
- Learn some basic statements to do a time series analysis using Python.
- Learn some basic statements to do a time series analysis using R.
- Explain in your own terms, how to perform a time series analysis.
- Identify the type of model to apply in a time series.
Who Should Attend
- Students who wish to acquire or improve their skills in data analysis through time series techniques.
- Python developers who want to improve their skills using time series techniques.
- Data Analysts.
- Beginning python and r developers interested in data science.
- Professionals in areas such as marketing, finance, retail, budget, production stock, and so forth.
Target Audiences
- Students who wish to acquire or improve their skills in data analysis through time series techniques.
- Python developers who want to improve their skills using time series techniques.
- Data Analysts.
- Beginning python and r developers interested in data science.
- Professionals in areas such as marketing, finance, retail, budget, production stock, and so forth.
There are several reasons why it is desirable to study a time series.
In general, we can say that, the study of a time series has as main objectives:
-
Describe
-
Predict
-
Explain
-
Control
One of the most important reasons for studying time series is for the purpose of making forecasts about the analyzed time series.
The reason that forecasting is so important is that prediction of future events is critical input into many types of planning and decision-making processes, with application to areas such Marketing, Finance Risk Management, Economics, Industrial Process Control, Demography, and so forth.
Despite the wide range of problem situations that require forecasts, there are only two broad types of forecasting techniques. These are Qualitative methods and Quantitative methods.
Qualitative forecasting techniques are often subjective in nature and require judgment on the part of experts.
Quantitative forecasting techniques make formal use of historical data and a forecasting model. The model formally summarizes patterns in the data and expresses a statistical relationship between previous (Tn-1), and current values (Tn), of the variable.
In other words, the forecasting model is used to extrapolate past and current behavior into the future. That’s what we’ll be learning in this course.
Regardless of your objective, this course is oriented to provide you with the basic foundations and knowledge, as well as a practical application, in the study of time series.
Students will find valuable resources, in addition to the video lessons, it has a large number of laboratories, which will allow you to apply in a practical way the concepts described in each lecture.
The labs are written in two of the most important languages in data science. These are python and r.
Course Curriculum
Chapter 1: Environment Preparation
Lecture 1: Install Anaconda Individual Edition
Lecture 2: First Anaconda Execution
Lecture 3: Install RStudio Free Edition
Lecture 4: First R lab execution
Chapter 2: Time Series – Concepts
Lecture 1: Basic Concepts
Lecture 2: Time Series Components
Lecture 3: Time Series Components Labs
Lecture 4: Time Series Decomposition Analysis
Lecture 5: Time Series Decomposition Labs
Chapter 3: Data Wrangling
Lecture 1: Loading Data
Lecture 2: Loading Data Labs
Lecture 3: Summary Data Part 1
Lecture 4: Summary Data Part Lab 1
Lecture 5: Summary Data – Part 2
Lecture 6: Summary Data Part Lab 2
Chapter 4: Differencing
Lecture 1: Differencing and Random Walk
Lecture 2: Differencing and Random Walk Lab
Lecture 3: Order Differencing
Lecture 4: Order Differencing Labs
Chapter 5: Time Series Models bases
Lecture 1: Autoregressive Model
Lecture 2: Moving Average Model
Lecture 3: Moving Average Model Lab
Lecture 4: BackShift Operator
Lecture 5: Difference Operator
Lecture 6: Auto Correlation Function
Lecture 7: Partial Autocorrelation Function
Lecture 8: Partial Autocorrelation Function Labs
Chapter 6: Time Series Models
Lecture 1: ARMA Model
Lecture 2: ARMA Model Labs
Lecture 3: ARIMA Model
Lecture 4: ARIMA Model Lab
Lecture 5: Dickey Fuller Test
Lecture 6: Dickey Fuller Test Lab
Lecture 7: Ljung-Box Q-statistics
Lecture 8: Model Basic Steps
Lecture 9: SARIMA Model
Lecture 10: SARIMA Model Labs
Chapter 7: Forecasting
Lecture 1: Forecast
Lecture 2: Simple Exponential Smoothing – Part 1
Lecture 3: Simple Exponential Smoothing – Part 2
Lecture 4: Simple Exponential Smoothing Labs
Lecture 5: Holt's Exponential Smoothing
Lecture 6: Holt's Exponential Smoothing Labs
Instructors
-
Christian Cisne
Statistician and Computers Programmer
Rating Distribution
- 1 stars: 0 votes
- 2 stars: 0 votes
- 3 stars: 0 votes
- 4 stars: 2 votes
- 5 stars: 4 votes
Frequently Asked Questions
How long do I have access to the course materials?
You can view and review the lecture materials indefinitely, like an on-demand channel.
Can I take my courses with me wherever I go?
Definitely! If you have an internet connection, courses on Udemy are available on any device at any time. If you don’t have an internet connection, some instructors also let their students download course lectures. That’s up to the instructor though, so make sure you get on their good side!
You may also like
- Digital Marketing Foundation Course
- Google Shopping Ads Digital Marketing Course
- Multi Cloud Infrastructure for beginners
- Master Lead Generation: Grow Subscribers & Sales with Popups
- Complete Copywriting System : write to sell with ease
- Product Positioning Masterclass: Unlock Market Traction
- How to Promote Your Webinar and Get More Attendees?
- Digital Marketing Courses
- Create music with Artificial Intelligence in this new market
- Create CONVERTING UGC Content So Brands Will Pay You More
- Podcast: The top 8 ways to monetize by Podcasting
- TikTok Marketing Mastery: Learn to Grow & Go Viral
- Free Digital Marketing Basics Course in Hindi
- MailChimp Free Mailing Lists: MailChimp Email Marketing
- Automate Digital Marketing & Social Media with Generative AI
- Google Ads MasterClass – All Advanced Features
- Online Course Creator: Create & Sell Online Courses Today!
- Introduction to SEO – Basic Principles of SEO
- Affiliate Marketing For Beginners: Go From Novice To Pro
- Effective Website Planning Made Simple