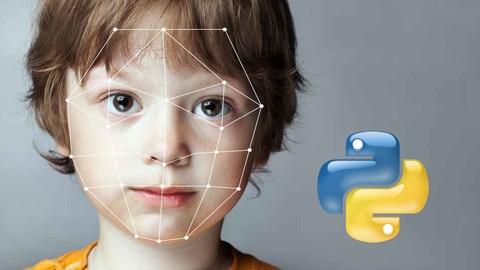
Computer Vision: Face Recognition Quick Starter in Python
Computer Vision: Face Recognition Quick Starter in Python, available at $89.99, has an average rating of 4.72, with 94 lectures, based on 425 reviews, and has 6564 subscribers.
You will learn about Face Detection from Images Face Detection from Realtime Videos Emotion Detection, Age-Gender Prediction Face Recognition from Images, Realtime Videos This course is ideal for individuals who are Beginners or who wants to start with Python based Face Recognition It is particularly useful for Beginners or who wants to start with Python based Face Recognition.
Enroll now: Computer Vision: Face Recognition Quick Starter in Python
Summary
Title: Computer Vision: Face Recognition Quick Starter in Python
Price: $89.99
Average Rating: 4.72
Number of Lectures: 94
Number of Published Lectures: 94
Number of Curriculum Items: 94
Number of Published Curriculum Objects: 94
Original Price: $109.99
Quality Status: approved
Status: Live
What You Will Learn
- Face Detection from Images
- Face Detection from Realtime Videos
- Emotion Detection, Age-Gender Prediction
- Face Recognition from Images, Realtime Videos
Who Should Attend
- Beginners or who wants to start with Python based Face Recognition
Target Audiences
- Beginners or who wants to start with Python based Face Recognition
Hi There!
welcome to my new course ‘Face Recognition with Deep Learning using Python’. This is an updated course from my Computer Vision series which covers Python Deep Learning based Face Detection, Face Recognition, Emotion , Gender and Age Classification using all popular models including Haar Cascade, HOG, SSD, MMOD, MTCNN, EigenFace, FisherFace, VGGFace, FaceNet, OpenFace, DeepFace
Face Detection and Face Recognition is the most used applications of Computer Vision. Using these techniques, the computer will be able to extract one or more faces in an image or video and then compare it with the existing data to identify the people in that image.
Face Detection and Face Recognition is widely used by governments and organizations for surveillance and policing. We are also making use of it daily in many applications like face unlocking of cell phones etc.
This course will be a quick starter for people who wants to dive deep into face recognition using Python without having to deal with all the complexities and mathematics associated with typical Deep Learning process.
We will be using a python library called face-recognition which uses simple classes and methods to get the face recognition implemented with ease. We are also using OpenCV, Dlib and Pillow for python as supporting libraries.
Let’s now see the list of interesting topics that are included in this course.
At first we will have an introductory theory session about Face Detection and Face Recognition technology.
After that, we are ready to proceed with preparing our computer for python coding by downloading and installing the anaconda package. Then we will install the rest of dependencies and libraries that we require including the dlib, face-recognition, opencv etc and will try a small program to see if everything is installed fine.
Most of you may not be coming from a python based programming background. The next few sessions and examples will help you get the basic python programming skill to proceed with the sessions included in this course. The topics include Python assignment, flow-control, functions and data structures.
Then we will have an introduction to the basics and working of face detectors which will detect human faces from a given media. We will try the python code to detect the faces from a given image and will extract the faces as separate images.
Then we will go ahead with face detection from a video. We will be streaming the real-time live video from the computer’s webcam and will try to detect faces from it. We will draw rectangle around each face detected in the live video.
In the next session, we will customize the face detection program to blur the detected faces dynamically from the webcam video stream.
After that we will try facial expression recognition using pre-trained deep learning model and will identify the facial emotions from the real-time webcam video as well as static images
And then we will try Age and Gender Prediction using pre-trained deep learning model and will identify the Age and Gender from the real-time webcam video as well as static images
After face detection, we will have an introduction to the basics and working of face recognition which will identify the faces already detected.
In the next session, We will try the python code to identify the names of people and their the faces from a given image and will draw a rectangle around the face with their names on it.
Then, like as we did in face detection we will go ahead with face recognition from a video. We will be streaming the real-time live video from the computer’s webcam and will try to identify and name the faces in it. We will draw rectangle around each face detected and beneath that their names in the live video.
Most times during coding, along with the face matching decision, we may need to know how much matching the face is. For that we will get a parameter called face distance which is the magnitude of matching of two faces. We will later convert this face distance value to face matching percentage using simple mathematics.
In the coming two sessions, we will learn how to tweak the face landmark points used for face detection. We will draw line joining these face land mark points so that we can visualize the points in the face which the computer is used for evaluation.
Taking the landmark points customization to the next level, we will use the landmark points to create a custom face make-up for the face image.
Till now we were using the face-recognition third party library to achieve most of the functionality. But from now onwards we will try the face-recognition pipeline steps which includes face detection, face alignment, face feature extraction verification and classification separately one by one using popular libraries. We will have an introduction about these in this session.
In the next session, we will start with face detection. We will divide them into traditional face detection methods and modern methods which involves CNN.
At first we will try the Haar Cascade object detection algorithm for face detection. We will try it at first for still images and later we will implement it for saved videos as well as live web cam videos.
Another popular algorithm for face detection is HOG or Histogram of Oriented Gradients. At first we will have an introduction to the working of HOG algorithm and then we will try the HOG method for images, videos and real-time web cam stream.
The next face detection algorithm we will try is SSD or Single Shot Detection. We will repeat the same functionality exercises for SSD also. And then comes MMOD. We will repeat the same functionality exercises for SSD also.
Then the MMOD, the Max-Margin Object Detection. We will repeat the same functionality exercises for MTCNN also.
Then comes the next algorithm which is MTCNN, the Multi-task Cascaded Convolutional Networks. We will repeat the same functionality exercises including image, video and real time stream for MTCNN also.
Finally we will have a quick comparison between the performance of these face detection algorithms.
After face detection, we will go ahead with face alignment. We will use the popular Dlib library python implementation to perform the face alignment for image, video and video streams.
After face alignment exercises, we will proceed with face verification and classification where the actual face recognition is happening. At first we will have an introduction about face classification. We will divide the techniques into traditional face recognition methods and modern methods which involves CNN.
At first we will try the techniques Eigenface Fisherface and LBPH, the Local Binary Pattern Histogram. We will have a short introduction about these algorithms and will then proceed with
preparing the image dataset for these algorithms.
Then we will set up the prerequisite for them. Later we will proceed with face detection using MTCNN and preprocessing of the detected face for recognition. Then the exercises involving training with the image dataset and trying prediction for images. We will then save this model so that we can load it later and do prediction without having to go through training again.
We will also try it for pre-saved videos and real time webcam stream. Once we are done with that we will have a quick comparison of the Eigenface Fisherface and LBPH algorithms.
That’s all the traditional ways, now we will proceed with deep learning face recognition. At first using the popular VGGNet model for face recognition called VGGface. We will have an introduction to VGG face and then we will implement VGGface face verification for images. Later we will try VGGface face verification for videos as well as realtime streams.
And then we have an introduction to FaceNet, OpenFace and DeepFace Models. We will use a popular easy to use open source python face recognition framework called deepface to implement the rest of popular deep learning techniques.
We will install deepface to our computer and then try it at first for face detection and face alignment. Then we will try deepface for face one to one verification. Later with few changes, we can use it for face classification which involves an one to many comparison. deepface can also be used for performing face analysis involving gender, age, emotion, ethnicity etc
That’s all about the topics which are currently included in this quick course. The code, images and libraries used in this course has been uploaded and shared in a folder. I will include the link to download them in the last session or the resource section of this course. You are free to use the code in your projects with no questions asked.
Also after completing this course, you will be provided with a course completion certificate which will add value to your portfolio.
So that’s all for now, see you soon in the class room. Happy learning and have a great time.
Course Curriculum
Chapter 1: Course Introduction and Table of Contents
Lecture 1: Course Introduction and Table of Contents
Chapter 2: Introduction to Face Recognition
Lecture 1: Introduction to Face Recognition
Chapter 3: Environment Setup: Installing Anaconda Package
Lecture 1: Environment Setup: Installing Anaconda Package
Chapter 4: Python Basics (Optional)
Lecture 1: Python Basics – Assignment
Lecture 2: Python Basics – Flow Control
Lecture 3: Python Basics – Data Structures
Lecture 4: Python Basics – Functions
Chapter 5: Setting up Environment – Additional Dependencies (With DLib Fixes)
Lecture 1: Setting up Environment – Additional Dependencies – Part 1
Lecture 2: Attempt 1 : Install Dlib for Anaconda the formal way
Lecture 3: Attempt 2: Installing DLib using Visual Studio Build Tools
Lecture 4: Setting up Environment – Additional Dependencies (With DLib Fixes) – Part 2
Chapter 6: (Optional) DLib Error : Downgrading Python and Fixing
Lecture 1: (Optional) DLib Error : Downgrading Python and Fixing
Chapter 7: Introduction to Face Detectors
Lecture 1: Introduction to Face Detectors
Chapter 8: Face Detection Implementation
Lecture 1: Face Detection Implementation – Part 1
Lecture 2: Face Detection Implementation – Part 2
Chapter 9: Optional: cv2.imshow() Not Responding Issue Fix
Lecture 1: Optional: cv2.imshow() Not Responding Issue Fix
Lecture 2: Optional Fix 2: cv2.imshow() Not Responding
Chapter 10: Realtime face detection from WebCam
Lecture 1: Realtime face detection – Part 1
Lecture 2: Realtime face detection – Part 2
Chapter 11: Video Face Detection
Lecture 1: Video Face Detection
Chapter 12: Realtime face detection – Face Blurring
Lecture 1: Realtime face detection – Face Blurring
Chapter 13: Real-time Facial Expression Detection – Installing Libraries
Lecture 1: Fix: if 'conda install' fails to resolve
Lecture 2: Real-time Facial Expression Detection – Installing Libraries
Lecture 3: IMPORTANT FIX: "ImportError: Keras requires TensorFlow 2.2 or higher' Windows OS
Lecture 4: 'keras.preprocessing.image' has no attribute 'img_to_array' Error Fix
Chapter 14: Real-time Facial Expression Detection – Implementation
Lecture 1: Real-time Facial Expression Detection – Implementation – Part 1
Lecture 2: Real-time Facial Expression Detection – Implementation – Part 2
Lecture 3: Fix for TypeError: Could not locate class 'Sequential'
Lecture 4: TensorFlow "Module Not Found" Error Fix (Optional) – Do ONLY if you have error
Lecture 5: Error: module 'keras.preprocessing.image' has no attribute 'img_to_array'
Chapter 15: Video Facial Expression Detection
Lecture 1: Video Facial Expression Detection
Chapter 16: Image Facial Expression Detection
Lecture 1: Image Facial Expression Detection
Chapter 17: Real-time Age and Gender Detection Introduction
Lecture 1: Real-time Age and Gender Detection Introduction
Chapter 18: Real-time Age and Gender Detection Implementation
Lecture 1: Real-time Age and Gender Detection Implementation
Chapter 19: Image Age and Gender Detection Implementation
Lecture 1: Image Age and Gender Detection Implementation
Chapter 20: Introduction to Face Recognition
Lecture 1: Introduction to Face Recognition
Chapter 21: Face Recognition Implementation
Lecture 1: Face Recognition Implementation – Part 1
Lecture 2: Face Recognition Implementation – Part 2
Chapter 22: Realtime Face Recognition
Lecture 1: Realtime Face Recognition – Part 1
Lecture 2: Realtime Face Recognition – Part 2
Chapter 23: Video Face Recognition
Lecture 1: Video Face Recognition
Chapter 24: Face Distance
Lecture 1: Face Distance – Part 1
Lecture 2: Face Distance – Part 2
Chapter 25: Face Landmarks Visualization
Lecture 1: Face Landmarks Visualization – Part 1
Lecture 2: Face Landmarks Visualization – Part 2
Chapter 26: Multi Face Landmarks
Lecture 1: Multi Face Landmarks
Chapter 27: Multi Face Landmarks from Real-time and Pre-saved Video
Lecture 1: Multi Face Landmarks from Real-time and Pre-saved Video
Chapter 28: Face Makeup Using Face Landmarks
Lecture 1: Face Makeup Using Face Landmarks
Chapter 29: Real-time Face Makeup
Lecture 1: Real-time Face Makeup
Chapter 30: Face Detection using Other popular libraries Intro
Lecture 1: Face Detection using Other popular libraries Intro
Chapter 31: Face Detection Image using Haar Cascade
Lecture 1: Face Detection Image using Haar Cascade – Part 1
Lecture 2: Face Detection Image using Haar Cascade – Part 2
Chapter 32: Video and Realtime Face Detection using Haar Cascade
Lecture 1: Video and Realtime Face Detection using Haar Cascade
Chapter 33: Face Detection using HOG Introduction
Lecture 1: Face Detection using HOG Introduction
Chapter 34: Face Detection from Image using HOG
Lecture 1: Face Detection from Image using HOG
Chapter 35: Face Detection from Video and Webcam using HOG
Lecture 1: Face Detection from Video and Webcam using HOG
Chapter 36: SSD Face Detection Introduction
Lecture 1: SSD Face Detection Introduction
Chapter 37: Face Detection from Image using SSD
Lecture 1: Face Detection from Image using SSD part 1
Lecture 2: Face Detection from Image using SSD part 2
Chapter 38: Face Detection From Video and Webcam using SSD
Lecture 1: Face Detection From Video and Webcam using SSD
Chapter 39: Face Detection from Image using MMOD
Lecture 1: Face Detection from Image using MMOD
Instructors
-
Abhilash Nelson
Computer Engineering Master & Senior Programmer at Dubai
Rating Distribution
- 1 stars: 2 votes
- 2 stars: 7 votes
- 3 stars: 41 votes
- 4 stars: 159 votes
- 5 stars: 216 votes
Frequently Asked Questions
How long do I have access to the course materials?
You can view and review the lecture materials indefinitely, like an on-demand channel.
Can I take my courses with me wherever I go?
Definitely! If you have an internet connection, courses on Udemy are available on any device at any time. If you don’t have an internet connection, some instructors also let their students download course lectures. That’s up to the instructor though, so make sure you get on their good side!
You may also like
- Learn to Fix iPhones and Start a Delivery Service
- Make Money With High Ticket Sales (Even If You're A Newbie)
- Masterclass: Start a Profitable Internet Cafe Business
- Google Analytics for Shopify: A Complete Step-by-Step Guide
- Creating Amazing Property Video (Using Your Smartphone)
- The Fundamentals of Business Intelligence (BI)
- Outsource Easier!
- Amazon SEO & Listing Optimization SECRETS to Double Sales
- Strategic Workforce Planning: A Fundamental Beginner's Guide
- Start A Start Hustle And Build A Second Income
- SPA RETAIL 101 – how to create a sales culture in your Spa?
- Beyond Upwork: How to Find Freelance Clients Outside Upwork
- How to manage event venue
- Ultimate Amazon FBA Mastery Course – Start With Any Budget
- The Gaming Youtube Masterclass
- Power BI Business User
- PMI – PgMP | 2024 Real Practice Exams (1150 Questions)
- Six Sigma: Certified Lean Six Sigma Green Belt | Accredited
- International B2B Trade Shows Management
- Project Management Guide for Human Resources (HR)