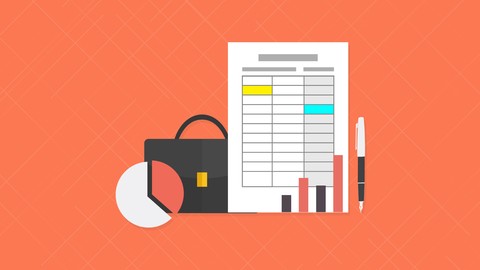
Applied Multivariate Analysis with R
Applied Multivariate Analysis with R, available at $59.99, has an average rating of 3.75, with 75 lectures, based on 377 reviews, and has 4453 subscribers.
You will learn about Conceptualize and apply multivariate skills and "hands-on" techniques using R software in analyzing real data. Create novel and stunning 2D and 3D multivariate data visualizations with R. Set up and estimate a Principal Components Analysis (PCA). Formulate and estimate a Multidimensional Scaling (MDS) problem. Group similar (or dissimilar) data with Cluster Analysis techniques. Estimate and interpret an Exploratory Factor Analysis (EFA). Specify and estimate a Structural Equation Model (SEM) using RAM notation in R. Be knowledgeable about SEM simulation capabilities from the R SIMSEM package. This course is ideal for individuals who are Anyone interested in using multivariate analysis technques as a basis for data mining, statistical modeling, and structural equation modeling (SEM) estimation. or Practicing quantitative analysis professionals including college and university faculty seeking to learn new multivariate data analysis skills. or Undergraduate students looking for jobs in predictive or business analytics fields. or Graduate students wishing to learn more applied data analysis techniques and approaches. It is particularly useful for Anyone interested in using multivariate analysis technques as a basis for data mining, statistical modeling, and structural equation modeling (SEM) estimation. or Practicing quantitative analysis professionals including college and university faculty seeking to learn new multivariate data analysis skills. or Undergraduate students looking for jobs in predictive or business analytics fields. or Graduate students wishing to learn more applied data analysis techniques and approaches.
Enroll now: Applied Multivariate Analysis with R
Summary
Title: Applied Multivariate Analysis with R
Price: $59.99
Average Rating: 3.75
Number of Lectures: 75
Number of Published Lectures: 75
Number of Curriculum Items: 75
Number of Published Curriculum Objects: 75
Original Price: $84.99
Quality Status: approved
Status: Live
What You Will Learn
- Conceptualize and apply multivariate skills and "hands-on" techniques using R software in analyzing real data.
- Create novel and stunning 2D and 3D multivariate data visualizations with R.
- Set up and estimate a Principal Components Analysis (PCA).
- Formulate and estimate a Multidimensional Scaling (MDS) problem.
- Group similar (or dissimilar) data with Cluster Analysis techniques.
- Estimate and interpret an Exploratory Factor Analysis (EFA).
- Specify and estimate a Structural Equation Model (SEM) using RAM notation in R.
- Be knowledgeable about SEM simulation capabilities from the R SIMSEM package.
Who Should Attend
- Anyone interested in using multivariate analysis technques as a basis for data mining, statistical modeling, and structural equation modeling (SEM) estimation.
- Practicing quantitative analysis professionals including college and university faculty seeking to learn new multivariate data analysis skills.
- Undergraduate students looking for jobs in predictive or business analytics fields.
- Graduate students wishing to learn more applied data analysis techniques and approaches.
Target Audiences
- Anyone interested in using multivariate analysis technques as a basis for data mining, statistical modeling, and structural equation modeling (SEM) estimation.
- Practicing quantitative analysis professionals including college and university faculty seeking to learn new multivariate data analysis skills.
- Undergraduate students looking for jobs in predictive or business analytics fields.
- Graduate students wishing to learn more applied data analysis techniques and approaches.
Applied Multivariate Analysis (MVA) with R is a practical, conceptual and applied “hands-on” course that teaches students how to perform various specific MVA tasks using real data sets and R software. It is an excellent and practical background course for anyone engaged with educational or professional tasks and responsibilities in the fields of data mining or predictive analytics, statistical or quantitative modeling (including linear, GLM and/or non-linear modeling, covariance-based Structural Equation Modeling (SEM) specification and estimation, and/or variance-based PLS Path Model specification and estimation. Students learn all about the nature of multivariate data and multivariate analysis. Students specifically learn how to create and estimate: covariance and correlation matrices; Principal Components Analyses (PCA); Multidimensional Scaling (MDS); Cluster Analysis; Exploratory Factor Analyses (EFA); and SEM model estimation. The course also teaches how to create dozens of different dazzling 2D and 3D multivariate data visualizations using R software. All software, R scripts, datasets and slides used in all lectures are provided in the course materials. The course is structured as a series of seven sections, each addressing a specific MVA topic and each section culminating with one or more “hands-on” exercises for the students to complete before proceeding to reinforce learning the presented MVA concepts and skills. The course is an excellent vehicle to acquire “real-world” predictive analytics skills that are in high demand today in the workplace. The course is also a fertile source of relevant skills and knowledge for graduate students and faculty who are required to analyze and interpret research data.
Course Curriculum
Chapter 1: Introduction to Multivariate Data and Analysis
Lecture 1: Introduction to Multivariate Analysis (MVA) Course
Lecture 2: Materials for Section 1 Introduction to MV Data and Analysis
Lecture 3: What is "Multivariate Analysis" ?
Lecture 4: Missing Values and the Measure Dataset
Lecture 5: Other Multivariate Datasets
Lecture 6: Covariance, Correlation and Distance (part 1)
Lecture 7: Covariance, Correlation and Distance (part 2)
Lecture 8: Covariance, Correlation and Distance (part 3)
Lecture 9: The Multivariate Normal Density Function
Lecture 10: Setting Up Normality Plots
Lecture 11: Drawing Normality Plots
Lecture 12: Covariance, Correlation and Normality Exercises
Chapter 2: Visualizing Multivariate Data
Lecture 1: Materials and Exercises for Visualizing Multivariate Data Section
Lecture 2: Covariance and Correlation Matrices with Missing Data (part 1)
Lecture 3: Covariance and Correlation Matrices with Missing Data (part 2)
Lecture 4: Univariate and Multivariate QQPlots of Pottery Data
Lecture 5: Converting Covariance to Correlation Matrices
Lecture 6: Plots for Marginal Distributions
Lecture 7: Outlier Identification
Lecture 8: Chi, Bubble, and other Glyph Plots
Lecture 9: Scatterplot Matrix
Lecture 10: Kernel Density Estimators
Lecture 11: 3-Dimensional and Trellis (Lattice Package) Graphics
Lecture 12: More Trellis (Lattice Package) Graphics
Lecture 13: Bivariate Boxplot and ChiPlot Visualizations Exercises
Chapter 3: Principal Components Analysis (PCA)
Lecture 1: Materials for Principal Components Analysis (PCA) Section
Lecture 2: Bivariate Boxplot Visualization Exercise Solution
Lecture 3: ChiPlot Visualization Exercise Solution
Lecture 4: What is a "Principal Components Analysis" (PCA) ?
Lecture 5: PCA Basics with R: Blood Data (part 1)
Lecture 6: PCA Basics with R: Blood Data (part 2)
Lecture 7: PCA with Head Size Data (part 1)
Lecture 8: PCA with Head Size Data (part 2)
Lecture 9: PCA with Heptathlon Data (part 1)
Lecture 10: PCA with Heptathlon Data (part 2)
Lecture 11: PCA with Heptathlon Data (part 3)
Lecture 12: PCA Criminal Convictions Exercise
Chapter 4: Multidimensional Scaling (MDS)
Lecture 1: Materials for Multidimensional Scaling Section
Lecture 2: PCA Criminal Convictions Exercise Solution
Lecture 3: Introduction to Multidimensional Scaling
Lecture 4: Classical Multidimensional Scaling (part 1)
Lecture 5: Classical Multidimensional Scaling (part 2)
Lecture 6: Classical Multidimensional Scaling: Skulls Data
Lecture 7: Non-Metric Multidimensional Scaling Example: Voting Behavior
Lecture 8: Non-Metric Multidimensional Scaling Example: WW II Leaders
Lecture 9: Multidimensional Scaling Exercise: Water Voles
Chapter 5: Cluster Analysis
Lecture 1: Materials for Cluster Analysis Section
Lecture 2: MDS Water Voles Exercise Solution
Lecture 3: Introduction to Cluster Analysis
Lecture 4: Hierarchical Clustering Distance Techniques
Lecture 5: Hierarchical Clustering of Measures Data
Lecture 6: Hierarchical Clustering of Fighter Jets
Lecture 7: K-Means Clustering of Crime Data (part 1)
Lecture 8: K-Means Clustering of Crime Data (part 2)
Lecture 9: Clustering of Romano-British Pottery Data
Lecture 10: K-Means Classifying of Exoplanets
Lecture 11: Model-Based Clustering of Exoplanets
Lecture 12: Finite Mixture Model-Based Analysis
Lecture 13: Cluster Analysis Neighborhood and Stripes Plots
Lecture 14: K-Means Cluster Analysis Crime Data Exercise
Chapter 6: Exploratory Factor Analysis (EFA)
Lecture 1: Materials for Exploratory Factor Analysis (EFA) Section
Lecture 2: K-Means Crime Data Exercise Solution
Lecture 3: Introduction to Exploratory Factor Analysis (EFA)
Lecture 4: The factanal() Function Explained
Lecture 5: EFA Life Data Example
Lecture 6: EFA Drug Use Data Example
Lecture 7: Comparing EFA with Confirmatory Factor Analysis (CFA)
Lecture 8: EFA Exercise
Chapter 7: Introduction to Structural Equation Modeling (SEM), QGraph, and SIMSEM
Lecture 1: Introduction to the SEM, QGraph and SIMSEM Course Section with Materials
Lecture 2: Exploratory Factor Analysis (EFA) Exercise Solution
Lecture 3: Specify and Estimate Drug Use SEM Model
Lecture 4: Specify and Estimate Alienation SEM Model
Lecture 5: QGraph Visualizations
Lecture 6: SIMSEM Package Simulation Capabilities (part 1)
Lecture 7: SIMSEM Package Simulation Capabilities (part 2)
Instructors
-
Geoffrey Hubona, Ph.D.
Associate Professor of MIS and Data Analytics
Rating Distribution
- 1 stars: 19 votes
- 2 stars: 27 votes
- 3 stars: 61 votes
- 4 stars: 136 votes
- 5 stars: 134 votes
Frequently Asked Questions
How long do I have access to the course materials?
You can view and review the lecture materials indefinitely, like an on-demand channel.
Can I take my courses with me wherever I go?
Definitely! If you have an internet connection, courses on Udemy are available on any device at any time. If you don’t have an internet connection, some instructors also let their students download course lectures. That’s up to the instructor though, so make sure you get on their good side!
You may also like
- Best Cybersecurity Fundamentals Courses to Learn in March 2025
- Best Smart Home Technology Courses to Learn in March 2025
- Best Holistic Health Courses to Learn in March 2025
- Best Nutrition And Diet Planning Courses to Learn in March 2025
- Best Yoga Instruction Courses to Learn in March 2025
- Best Stress Management Courses to Learn in March 2025
- Best Mindfulness Meditation Courses to Learn in March 2025
- Best Life Coaching Courses to Learn in March 2025
- Best Career Development Courses to Learn in March 2025
- Best Relationship Building Courses to Learn in March 2025
- Best Parenting Skills Courses to Learn in March 2025
- Best Home Improvement Courses to Learn in March 2025
- Best Gardening Courses to Learn in March 2025
- Best Sewing And Knitting Courses to Learn in March 2025
- Best Interior Design Courses to Learn in March 2025
- Best Writing Courses Courses to Learn in March 2025
- Best Storytelling Courses to Learn in March 2025
- Best Creativity Workshops Courses to Learn in March 2025
- Best Resilience Training Courses to Learn in March 2025
- Best Emotional Intelligence Courses to Learn in March 2025