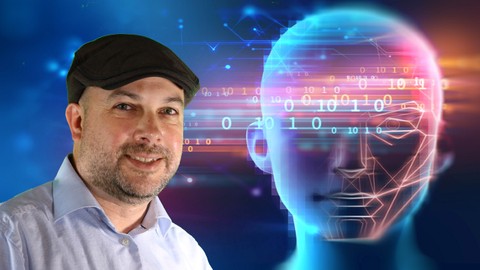
Building Recommender Systems with Machine Learning and AI
Building Recommender Systems with Machine Learning and AI, available at $99.99, has an average rating of 4.45, with 133 lectures, based on 3080 reviews, and has 46874 subscribers.
You will learn about Understand and apply user-based and item-based collaborative filtering to recommend items to users Create recommendations using deep learning at massive scale Build recommendation engines with neural networks and Restricted Boltzmann Machines (RBM's) Make session-based recommendations with recurrent neural networks and Gated Recurrent Units (GRU) Build a framework for testing and evaluating recommendation algorithms with Python Apply the right measurements of a recommender system's success Build recommender systems with matrix factorization methods such as SVD and SVD++ Apply real-world learnings from Netflix and YouTube to your own recommendation projects Combine many recommendation algorithms together in hybrid and ensemble approaches Use Apache Spark to compute recommendations at large scale on a cluster Use K-Nearest-Neighbors to recommend items to users Solve the "cold start" problem with content-based recommendations Understand solutions to common issues with large-scale recommender systems This course is ideal for individuals who are Software developers interested in applying machine learning and deep learning to product or content recommendations or Engineers working at, or interested in working at large e-commerce or web companies or Computer Scientists interested in the latest recommender system theory and research It is particularly useful for Software developers interested in applying machine learning and deep learning to product or content recommendations or Engineers working at, or interested in working at large e-commerce or web companies or Computer Scientists interested in the latest recommender system theory and research.
Enroll now: Building Recommender Systems with Machine Learning and AI
Summary
Title: Building Recommender Systems with Machine Learning and AI
Price: $99.99
Average Rating: 4.45
Number of Lectures: 133
Number of Published Lectures: 131
Number of Curriculum Items: 133
Number of Published Curriculum Objects: 131
Original Price: $22.99
Quality Status: approved
Status: Live
What You Will Learn
- Understand and apply user-based and item-based collaborative filtering to recommend items to users
- Create recommendations using deep learning at massive scale
- Build recommendation engines with neural networks and Restricted Boltzmann Machines (RBM's)
- Make session-based recommendations with recurrent neural networks and Gated Recurrent Units (GRU)
- Build a framework for testing and evaluating recommendation algorithms with Python
- Apply the right measurements of a recommender system's success
- Build recommender systems with matrix factorization methods such as SVD and SVD++
- Apply real-world learnings from Netflix and YouTube to your own recommendation projects
- Combine many recommendation algorithms together in hybrid and ensemble approaches
- Use Apache Spark to compute recommendations at large scale on a cluster
- Use K-Nearest-Neighbors to recommend items to users
- Solve the "cold start" problem with content-based recommendations
- Understand solutions to common issues with large-scale recommender systems
Who Should Attend
- Software developers interested in applying machine learning and deep learning to product or content recommendations
- Engineers working at, or interested in working at large e-commerce or web companies
- Computer Scientists interested in the latest recommender system theory and research
Target Audiences
- Software developers interested in applying machine learning and deep learning to product or content recommendations
- Engineers working at, or interested in working at large e-commerce or web companies
- Computer Scientists interested in the latest recommender system theory and research
Updated with Neural Collaborative Filtering (NCF), Tensorflow Recommenders (TFRS) and Generative Adversarial Networks for recommendations (GANs)
Learn how to build machine learning recommendation systems from one of Amazon’s pioneers in the field. Frank Kane spent over nine years at Amazon, where he managed and led the development of many of Amazon’s personalized product recommendation systems.
You’ve seen automated recommendations everywhere – on Netflix’s home page, on YouTube, and on Amazon as these machine learning algorithms learn about your unique interests, and show the best products or content for you as an individual. These technologies have become central to the largest, most prestigious tech employers out there, and by understanding how they work, you’ll become very valuable to them.
We’ll cover tried and true recommendation algorithms based on neighborhood-based collaborative filtering, and work our way up to more modern techniques including matrix factorization and even deep learning with artificial neural networks. Along the way, you’ll learn from Frank’s extensive industry experience to understand the real-world challenges you’ll encounter when applying these algorithms at large scale and with real-world data.
However, this course is very hands-on; you’ll develop your own framework for evaluating and combining many different recommendation algorithms together, and you’ll even build your own neural networks using Tensorflowto generate recommendations from real-world movie ratings from real people. We’ll cover:
-
Building a recommendation engine
-
Evaluatingrecommender systems
-
Content-based filtering using item attributes
-
Neighborhood-based collaborative filtering with user-based, item-based, and KNN CF
-
Model-based methods including matrix factorization and SVD
-
Applying deep learning, AI, and artificial neural networks to recommendations
-
Using the latest frameworks from Tensorflow (TFRS) and Amazon Personalize.
-
Session-based recommendations with recursive neural networks
-
Building modern recommenders with neural collaborative filtering
-
Scaling to massive data sets with Apache Spark machine learning, Amazon DSSTNE deep learning, and AWS SageMaker with factorization machines
-
Real-world challenges and solutions with recommender systems
-
Case studies from YouTubeand Netflix
-
Building hybrid, ensemble recommenders
-
“Bleeding edge alerts” covering the latest research in the field of recommender systems
This comprehensive course takes you all the way from the early days of collaborative filtering, to bleeding-edge applications of deep neural networks and modern machine learning techniques for recommending the best items to every individual user.
The coding exercises in this course use the Pythonprogramming language. We include an intro to Python if you’re new to it, but you’ll need some prior programming experience in order to use this course successfully. Learning how to code is not the focus of this course; it’s the algorithms we’re primarily trying to teach, along with practical examples. We also include a short introduction to deep learning if you are new to the field of artificial intelligence, but you’ll need to be able to understand new computer algorithms.
High-quality, hand-edited English closed captions are included to help you follow along.
I hope to see you in the course soon!
Course Curriculum
Chapter 1: Getting Started
Lecture 1: Udemy 101: Getting the Most From This Course
Lecture 2: Important note
Lecture 3: Note: Alternate dataset download location
Lecture 4: [Activity] Install Anaconda, course materials, and create movie recommendations!
Lecture 5: Course Roadmap
Lecture 6: What Is a Recommender System?
Lecture 7: Types of Recommenders
Lecture 8: Understanding You through Implicit and Explicit Ratings
Lecture 9: Top-N Recommender Architecture
Lecture 10: [Quiz] Review the basics of recommender systems.
Chapter 2: Introduction to Python [Optional]
Lecture 1: [Activity] The Basics of Python
Lecture 2: Data Structures in Python
Lecture 3: Functions in Python
Lecture 4: [Exercise] Booleans, loops, and a hands-on challenge
Chapter 3: Evaluating Recommender Systems
Lecture 1: Train/Test and Cross Validation
Lecture 2: Accuracy Metrics (RMSE, MAE)
Lecture 3: Top-N Hit Rate – Many Ways
Lecture 4: Coverage, Diversity, and Novelty
Lecture 5: Churn, Responsiveness, and A/B Tests
Lecture 6: [Quiz] Review ways to measure your recommender.
Lecture 7: [Activity] Walkthrough of RecommenderMetrics.py
Lecture 8: [Activity] Walkthrough of TestMetrics.py
Lecture 9: [Activity] Measure the Performance of SVD Recommendations
Chapter 4: A Recommender Engine Framework
Lecture 1: Our Recommender Engine Architecture
Lecture 2: [Activity] Recommender Engine Walkthrough, Part 1
Lecture 3: [Activity] Recommender Engine Walkthrough, Part 2
Lecture 4: [Activity] Review the Results of our Algorithm Evaluation.
Chapter 5: Content-Based Filtering
Lecture 1: Content-Based Recommendations, and the Cosine Similarity Metric
Lecture 2: K-Nearest-Neighbors and Content Recs
Lecture 3: [Activity] Producing and Evaluating Content-Based Movie Recommendations
Lecture 4: A Note on Using Implicit Ratings.
Lecture 5: [Activity] Bleeding Edge Alert! Mise en Scene Recommendations
Lecture 6: [Exercise] Dive Deeper into Content-Based Recommendations
Chapter 6: Neighborhood-Based Collaborative Filtering
Lecture 1: Measuring Similarity, and Sparsity
Lecture 2: Similarity Metrics
Lecture 3: User-based Collaborative Filtering
Lecture 4: [Activity] User-based Collaborative Filtering, Hands-On
Lecture 5: Item-based Collaborative Filtering
Lecture 6: [Activity] Item-based Collaborative Filtering, Hands-On
Lecture 7: [Exercise] Tuning Collaborative Filtering Algorithms
Lecture 8: [Activity] Evaluating Collaborative Filtering Systems Offline
Lecture 9: [Exercise] Measure the Hit Rate of Item-Based Collaborative Filtering
Lecture 10: KNN Recommenders
Lecture 11: [Activity] Running User and Item-Based KNN on MovieLens
Lecture 12: [Exercise] Experiment with different KNN parameters.
Lecture 13: Bleeding Edge Alert! Translation-Based Recommendations
Chapter 7: Matrix Factorization Methods
Lecture 1: Principal Component Analysis (PCA)
Lecture 2: Singular Value Decomposition
Lecture 3: [Activity] Running SVD and SVD++ on MovieLens
Lecture 4: Improving on SVD
Lecture 5: [Exercise] Tune the hyperparameters on SVD
Lecture 6: Bleeding Edge Alert! Sparse Linear Methods (SLIM)
Chapter 8: Introduction to Deep Learning [Optional]
Lecture 1: Deep Learning Introduction
Lecture 2: Deep Learning Pre-Requisites
Lecture 3: History of Artificial Neural Networks
Lecture 4: [Activity] Playing with Tensorflow
Lecture 5: Training Neural Networks
Lecture 6: Tuning Neural Networks
Lecture 7: Activation Functions: More Depth
Lecture 8: Introduction to Tensorflow
Lecture 9: Important Tensorflow setup note!
Lecture 10: [Activity] Handwriting Recognition with Tensorflow, part 1
Lecture 11: [Activity] Handwriting Recognition with Tensorflow, part 2
Lecture 12: Introduction to Keras
Lecture 13: [Activity] Handwriting Recognition with Keras
Lecture 14: Classifier Patterns with Keras
Lecture 15: [Exercise] Predict Political Parties of Politicians with Keras
Lecture 16: Intro to Convolutional Neural Networks (CNN's)
Lecture 17: CNN Architectures
Lecture 18: [Activity] Handwriting Recognition with Convolutional Neural Networks (CNNs)
Lecture 19: Intro to Recurrent Neural Networks (RNN's)
Lecture 20: Training Recurrent Neural Networks
Lecture 21: [Activity] Sentiment Analysis of Movie Reviews using RNN's and Keras
Lecture 22: Tuning Neural Networks
Lecture 23: Neural Network Regularization Techniques
Lecture 24: Generative Adversarial Networks (GAN's)
Lecture 25: GAN's in Action
Lecture 26: [Activity] Generating images of clothing with Generative Adversarial Networks
Chapter 9: Deep Learning for Recommender Systems
Lecture 1: Intro to Deep Learning for Recommenders
Lecture 2: Restricted Boltzmann Machines (RBM's)
Lecture 3: [Activity] Recommendations with RBM's, part 1
Lecture 4: [Activity] Recommendations with RBM's, part 2
Lecture 5: [Activity] Evaluating the RBM Recommender
Lecture 6: [Exercise] Tuning Restricted Boltzmann Machines
Lecture 7: Exercise Results: Tuning a RBM Recommender
Lecture 8: Auto-Encoders for Recommendations: Deep Learning for Recs
Lecture 9: [Activity] Recommendations with Deep Neural Networks
Lecture 10: Clickstream Recommendations with RNN's
Lecture 11: [Exercise] Get GRU4Rec Working on your Desktop
Lecture 12: Exercise Results: GRU4Rec in Action
Lecture 13: Bleeding Edge Alert! Generative Adversarial Networks for Recommendations
Instructors
-
Sundog Education by Frank Kane
Join over 800K students learning ML, AI, AWS, and Data Eng. -
Frank Kane
Ex-Amazon Sr. Engineer and Sr. Manager, CEO Sundog Education -
Sundog Education Team
Sundog Education Team
Rating Distribution
- 1 stars: 60 votes
- 2 stars: 42 votes
- 3 stars: 238 votes
- 4 stars: 969 votes
- 5 stars: 1770 votes
Frequently Asked Questions
How long do I have access to the course materials?
You can view and review the lecture materials indefinitely, like an on-demand channel.
Can I take my courses with me wherever I go?
Definitely! If you have an internet connection, courses on Udemy are available on any device at any time. If you don’t have an internet connection, some instructors also let their students download course lectures. That’s up to the instructor though, so make sure you get on their good side!
- The Practical BPMN 2.0 Master Class
- Productivity and Efficiency Hacks
- IIBA CBAP CCBA ECBA Business Analysis Certification – Part 6
- Revealed: Excel Power Query One Hour Bootcamp (Great Stuff)
- Unlock the story from data
- PMI-ACP Certification: Planning and Monitoring Iterations
- Shopware Template Training Advanced – English
- Advanced Applied SQL for Business Intelligence and Analytics
- Writing/Editing Tips & Tricks – Writing Like A Pro
- Fashion Brand Launch: Wholesale Strategies
- SalesVu POS Rep Training Course
- Create A Code Of Conduct That Reflect Your Company's Values
- No-Code AI for Civil Engineers
- Introduction to Cybersecurity
- Lean Six Sigma Green Belt
- Mastering Agile Capacity Planning with Live Demo
- Effective Sales Skills
- How to Actually Open a Restaurant: The Only Course You Need
- Notion for Project Management – The Ultimate Course
- Effective Delegation: Mastering Task Management and AI Tools