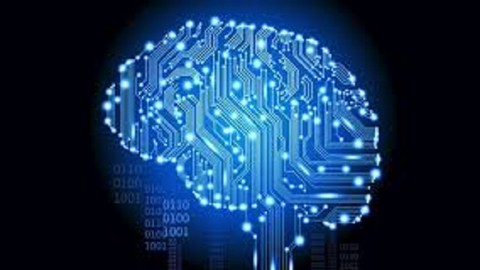
Complete Machine Learning course
Complete Machine Learning course, available at $49.99, has an average rating of 4.3, with 20 lectures, based on 38 reviews, and has 96 subscribers.
You will learn about Basics of machine learning Linear Regression Logistic Regression KNN alogrithm Clustering K-Means Clustering Principal component analysis Data preprocsseing EDA The Machine Learning Process Naive Bayes Classifier Supervised learning and unsupervised learning Confusion Matrix The Elbow Method Feature Scaling Feature Scaling Make Predictions Splitting your data into a Training set and a Test set Classification Machine Learning preparation Ordinary Least Squares Accuracy Decision Tree algorithm Random forest algorithm This course is ideal for individuals who are Anyone interested in Data Science or Data Science professionals or Machine learning engineer or Learner who want to use Machine Learning to their CV or career toolkit It is particularly useful for Anyone interested in Data Science or Data Science professionals or Machine learning engineer or Learner who want to use Machine Learning to their CV or career toolkit.
Enroll now: Complete Machine Learning course
Summary
Title: Complete Machine Learning course
Price: $49.99
Average Rating: 4.3
Number of Lectures: 20
Number of Published Lectures: 20
Number of Curriculum Items: 20
Number of Published Curriculum Objects: 20
Original Price: $22.99
Quality Status: approved
Status: Live
What You Will Learn
- Basics of machine learning
- Linear Regression
- Logistic Regression
- KNN alogrithm
- Clustering
- K-Means Clustering
- Principal component analysis
- Data preprocsseing
- EDA
- The Machine Learning Process
- Naive Bayes Classifier
- Supervised learning and unsupervised learning
- Confusion Matrix
- The Elbow Method
- Feature Scaling
- Feature Scaling
- Make Predictions
- Splitting your data into a Training set and a Test set
- Classification
- Machine Learning preparation
- Ordinary Least Squares
- Accuracy
- Decision Tree algorithm
- Random forest algorithm
Who Should Attend
- Anyone interested in Data Science
- Data Science professionals
- Machine learning engineer
- Learner who want to use Machine Learning to their CV or career toolkit
Target Audiences
- Anyone interested in Data Science
- Data Science professionals
- Machine learning engineer
- Learner who want to use Machine Learning to their CV or career toolkit
This course will cover following topics
1. Basics of machine learning
2. Supervised and unsupervised learning
3. Linear regression
4. Logistic regression
5. KNN Algorithm
6. Naive Bayes Classifier
7. Random forest Algorithm
8. Decision Tree Algorithm
7. Principal component analysis
8. K means clustering
9. Agglomerative clustering
10. There will practical exercise based on Linear regression, Logistic regression ,Naive Bayes, KNN algorithm, Random forest, Decision tree, K Means, PCA .
11. There will be quiz for each topics and total 200 Questions on machine learning course
We will look first in to linear Regression, where we will learn to predict continuous variables and this will details of Simple and Multiple Linear Regression, Ordinary Least Squares, Testing your Model, R Squared and Adjusted R Squared.
We will get full details of Logistic Regression, which is by far the most popular model for Classification. We will learn all about Maximum Likelihood, Feature Scaling, The Confusion Matrix, Accuracy Ratios and you will build your very first Logistic Regression
We will look in to Naive bias classifier which will give full details of Bayes Theorem, implementation of Naive bias in machine learning. This can be used in Spam Filtering, Text analysis, Recommendation Systems.
Random forest algorithm can be used in regression and classification problems. This gives good accuracy even if
data is incomplete.
Decision Tree is a Supervised learning technique that can be used for both classification and Regression problems, but mostly it is preferred for solving Classification problems.
We will look in to KNN algorithm which will working way of KNN algorithm, compute KNN distance matrix, Makowski distance, live examples of implementation of KNN in industry.
We will look in to PCA, K means clustering, Agglomerative clustering which will be part of unsupervised learning.
Along all part of machine supervised and unsupervised learning , we will be following data reading , data prerprocessing, EDA, data scaling, preparation of training and testing data along machine learning model selection , implemention and prediction of models.
Course Curriculum
Chapter 1: Basics of machine learning
Lecture 1: Introduction to Machine learning course
Lecture 2: Basics of machine learning, data in machine learning
Lecture 3: Supervised learning, Unsupervised learning , advantages and disadvantages of ML
Lecture 4: ML life cycle, Exploratory data analysis , ML Challenges and libraries
Chapter 2: Linear Regression
Lecture 1: Linear and multiple linear regression, cost function, gradient decent method
Lecture 2: practical exercise – car price prediction model using linear regression
Lecture 3: Assumptions, Advantages and disadvantage, best practices, MAE, MAPE,MSE L regres
Chapter 3: Logistic regression
Lecture 1: Logistic regression
Lecture 2: pratical exerice – Heart disease analysis using logistic regression
Chapter 4: KNN Algorithm
Lecture 1: KNN Algorithm
Lecture 2: Practical exercise using KNN Algorithm for Tumor classification
Chapter 5: Naïve Bayes Algorithm
Lecture 1: Naïve Bayes Algorithm
Lecture 2: Practical excerise using Navie Bayes for SPAMs
Chapter 6: Random forest algorithm
Lecture 1: Random forest alorgthim
Lecture 2: Practical example using Random forest algorithm
Chapter 7: decision tree algorithm
Lecture 1: decision tree algorithm
Lecture 2: Practical example using decision tree algorithm
Chapter 8: Unsupervised learning
Lecture 1: Unsupervised learning , type of unsupervised learning, adv and disadvantages etc
Chapter 9: PCA and live exercise on unsupervised learning.
Lecture 1: Principal component analysis
Lecture 2: live exercise on unsupervised learning.
Instructors
-
Satyendra Singh (NCFM and NSIM certified ) Technical and analyst, portfolio manager,Machine learning expert
Stock market Analyst (NSE ACEDEMY NCMP- Level 1 Awarded)
Rating Distribution
- 1 stars: 5 votes
- 2 stars: 2 votes
- 3 stars: 1 votes
- 4 stars: 2 votes
- 5 stars: 28 votes
Frequently Asked Questions
How long do I have access to the course materials?
You can view and review the lecture materials indefinitely, like an on-demand channel.
Can I take my courses with me wherever I go?
Definitely! If you have an internet connection, courses on Udemy are available on any device at any time. If you don’t have an internet connection, some instructors also let their students download course lectures. That’s up to the instructor though, so make sure you get on their good side!
You may also like
- Digital Marketing Foundation Course
- Google Shopping Ads Digital Marketing Course
- Multi Cloud Infrastructure for beginners
- Master Lead Generation: Grow Subscribers & Sales with Popups
- Complete Copywriting System : write to sell with ease
- Product Positioning Masterclass: Unlock Market Traction
- How to Promote Your Webinar and Get More Attendees?
- Digital Marketing Courses
- Create music with Artificial Intelligence in this new market
- Create CONVERTING UGC Content So Brands Will Pay You More
- Podcast: The top 8 ways to monetize by Podcasting
- TikTok Marketing Mastery: Learn to Grow & Go Viral
- Free Digital Marketing Basics Course in Hindi
- MailChimp Free Mailing Lists: MailChimp Email Marketing
- Automate Digital Marketing & Social Media with Generative AI
- Google Ads MasterClass – All Advanced Features
- Online Course Creator: Create & Sell Online Courses Today!
- Introduction to SEO – Basic Principles of SEO
- Affiliate Marketing For Beginners: Go From Novice To Pro
- Effective Website Planning Made Simple