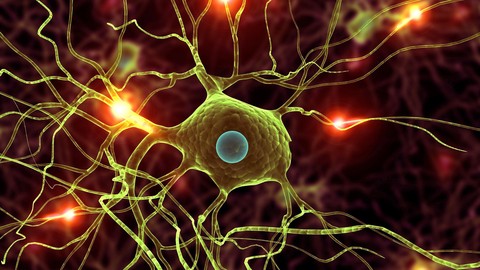
Data Science: Modern Deep Learning in Python
Data Science: Modern Deep Learning in Python, available at $109.99, has an average rating of 4.64, with 97 lectures, based on 3523 reviews, and has 39051 subscribers.
You will learn about Apply momentum to backpropagation to train neural networks Apply adaptive learning rate procedures like AdaGrad, RMSprop, and Adam to backpropagation to train neural networks Understand the basic building blocks of TensorFlow Build a neural network in TensorFlow Write a neural network using Keras Write a neural network using PyTorch Understand the difference between full gradient descent, batch gradient descent, and stochastic gradient descent Understand and implement dropout regularization Understand and implement batch normalization Understand the basic building blocks of Theano Build a neural network in Theano Write a neural network using CNTK Write a neural network using MXNet Understand important foundations for OpenAI ChatGPT, GPT-4, DALL-E, Midjourney, and Stable Diffusion This course is ideal for individuals who are Students and professionals who want to deepen their machine learning knowledge or Data scientists who want to learn more about deep learning or Data scientists who already know about backpropagation and gradient descent and want to improve it with stochastic batch training, momentum, and adaptive learning rate procedures like RMSprop or Those who do not yet know about backpropagation or softmax should take my earlier course, deep learning in Python, first It is particularly useful for Students and professionals who want to deepen their machine learning knowledge or Data scientists who want to learn more about deep learning or Data scientists who already know about backpropagation and gradient descent and want to improve it with stochastic batch training, momentum, and adaptive learning rate procedures like RMSprop or Those who do not yet know about backpropagation or softmax should take my earlier course, deep learning in Python, first.
Enroll now: Data Science: Modern Deep Learning in Python
Summary
Title: Data Science: Modern Deep Learning in Python
Price: $109.99
Average Rating: 4.64
Number of Lectures: 97
Number of Published Lectures: 90
Number of Curriculum Items: 97
Number of Published Curriculum Objects: 90
Original Price: $199.99
Quality Status: approved
Status: Live
What You Will Learn
- Apply momentum to backpropagation to train neural networks
- Apply adaptive learning rate procedures like AdaGrad, RMSprop, and Adam to backpropagation to train neural networks
- Understand the basic building blocks of TensorFlow
- Build a neural network in TensorFlow
- Write a neural network using Keras
- Write a neural network using PyTorch
- Understand the difference between full gradient descent, batch gradient descent, and stochastic gradient descent
- Understand and implement dropout regularization
- Understand and implement batch normalization
- Understand the basic building blocks of Theano
- Build a neural network in Theano
- Write a neural network using CNTK
- Write a neural network using MXNet
- Understand important foundations for OpenAI ChatGPT, GPT-4, DALL-E, Midjourney, and Stable Diffusion
Who Should Attend
- Students and professionals who want to deepen their machine learning knowledge
- Data scientists who want to learn more about deep learning
- Data scientists who already know about backpropagation and gradient descent and want to improve it with stochastic batch training, momentum, and adaptive learning rate procedures like RMSprop
- Those who do not yet know about backpropagation or softmax should take my earlier course, deep learning in Python, first
Target Audiences
- Students and professionals who want to deepen their machine learning knowledge
- Data scientists who want to learn more about deep learning
- Data scientists who already know about backpropagation and gradient descent and want to improve it with stochastic batch training, momentum, and adaptive learning rate procedures like RMSprop
- Those who do not yet know about backpropagation or softmax should take my earlier course, deep learning in Python, first
Ever wondered how AI technologies like OpenAI ChatGPT, GPT-4, DALL-E, Midjourney, and Stable Diffusion really work? In this course, you will learn the foundations of these groundbreaking applications.
This course continues where my first course, Deep Learning in Python, left off. You already know how to build an artificial neural network in Python, and you have a plug-and-play script that you can use for TensorFlow. Neural networks are one of the staples of machine learning, and they are always a top contender in Kaggle contests. If you want to improve your skills with neural networks and deep learning, this is the course for you.
You already learned about backpropagation, but there were a lot of unanswered questions. How can you modify it to improve training speed? In this course you will learn about batch and stochastic gradient descent, two commonly used techniques that allow you to train on just a small sample of the data at each iteration, greatly speeding up training time.
You will also learn about momentum, which can be helpful for carrying you through local minima and prevent you from having to be too conservative with your learning rate. You will also learn about adaptive learning rate techniques like AdaGrad, RMSprop, and Adam which can also help speed up your training.
Because you already know about the fundamentals of neural networks, we are going to talk about more modern techniques, like dropout regularizationand batch normalization, which we will implement in both TensorFlow and Theano. The course is constantly being updated and more advanced regularization techniques are coming in the near future.
In my last course, I just wanted to give you a little sneak peak at TensorFlow. In this course we are going to start from the basics so you understand exactly what’s going on – what are TensorFlow variables and expressions and how can you use these building blocks to create a neural network? We are also going to look at a library that’s been around much longer and is very popular for deep learning – Theano. With this library we will also examine the basic building blocks – variables, expressions, and functions – so that you can build neural networks in Theano with confidence.
Theano was the predecessor to all modern deep learning libraries today. Today, we have almost TOO MANY options. Keras, PyTorch, CNTK (Microsoft), MXNet (Amazon / Apache), etc. In this course, we cover all of these! Pick and choose the one you love best.
Because one of the main advantages of TensorFlow and Theano is the ability to use the GPU to speed up training, I will show you how to set up a GPU-instance on AWS and compare the speed of CPU vs GPU for training a deep neural network.
With all this extra speed, we are going to look at a real dataset – the famous MNIST dataset (images of handwritten digits) and compare against various benchmarks. This is THE dataset researchers look at first when they want to ask the question, “does this thing work?”
These images are important part of deep learning history and are still used for testing today. Every deep learning expert should know them well.
This course focuses on “how to build and understand“, not just “how to use”. Anyone can learn to use an API in 15 minutes after reading some documentation. It’s not about “remembering facts”, it’s about “seeing for yourself” via experimentation. It will teach you how to visualize what’s happening in the model internally. If you want more than just a superficial look at machine learning models, this course is for you.
“If you can’t implement it, you don’t understand it”
-
Or as the great physicist Richard Feynman said: “What I cannot create, I do not understand”.
-
My courses are the ONLY courses where you will learn how to implement machine learning algorithms from scratch
-
Other courses will teach you how to plug in your data into a library, but do you really need help with 3 lines of code?
-
After doing the same thing with 10 datasets, you realize you didn’t learn 10 things. You learned 1 thing, and just repeated the same 3 lines of code 10 times…
Suggested Prerequisites:
-
Know about gradient descent
-
Probability and statistics
-
Python coding: if/else, loops, lists, dicts, sets
-
Numpy coding: matrix and vector operations, loading a CSV file
-
Know how to write a neural network with Numpy
WHAT ORDER SHOULD I TAKE YOUR COURSES IN?:
-
Check out the lecture “Machine Learning and AI Prerequisite Roadmap” (available in the FAQ of any of my courses, including the free Numpy course)
Course Curriculum
Chapter 1: Introduction and Outline
Lecture 1: Introduction and Outline
Lecture 2: Where to get the Code
Lecture 3: How to Succeed in this Course
Chapter 2: Review
Lecture 1: Review (pt 1): Neuron Predictions
Lecture 2: Review (pt 2): Neuron Learning
Lecture 3: Review (pt 3): Artificial Neural Networks
Lecture 4: Review Exercise Prompt
Lecture 5: Review Code (pt 1)
Lecture 6: Review Code (pt 2)
Lecture 7: Review Summary
Chapter 3: Stochastic Gradient Descent and Mini-Batch Gradient Descent
Lecture 1: Stochastic Gradient Descent and Mini-Batch Gradient Descent (Theory)
Lecture 2: SGD Exercise Prompt
Lecture 3: Stochastic Gradient Descent and Mini-Batch Gradient Descent (Code pt 1)
Lecture 4: Stochastic Gradient Descent and Mini-Batch Gradient Descent (Code pt 2)
Chapter 4: Momentum and adaptive learning rates
Lecture 1: Using Momentum to Speed Up Training
Lecture 2: Nesterov Momentum
Lecture 3: Momentum in Code
Lecture 4: Variable and adaptive learning rates
Lecture 5: Constant learning rate vs. RMSProp in Code
Lecture 6: Adam Optimization (pt 1)
Lecture 7: Adam Optimization (pt 2)
Lecture 8: Adam in Code
Lecture 9: Suggestion Box
Chapter 5: Choosing Hyperparameters
Lecture 1: Hyperparameter Optimization: Cross-validation, Grid Search, and Random Search
Lecture 2: Sampling Logarithmically
Lecture 3: Grid Search in Code
Lecture 4: Modifying Grid Search
Lecture 5: Random Search in Code
Chapter 6: Weight Initialization
Lecture 1: Weight Initialization Section Introduction
Lecture 2: Vanishing and Exploding Gradients
Lecture 3: Weight Initialization
Lecture 4: Local vs. Global Minima
Lecture 5: Weight Initialization Section Summary
Chapter 7: Theano
Lecture 1: Theano Basics: Variables, Functions, Expressions, Optimization
Lecture 2: Building a neural network in Theano
Lecture 3: Is Theano Dead?
Chapter 8: TensorFlow
Lecture 1: TensorFlow Basics: Variables, Functions, Expressions, Optimization
Lecture 2: Building a neural network in TensorFlow
Lecture 3: What is a Session? (And more)
Chapter 9: GPU Speedup, Homework, and Other Misc Topics
Lecture 1: Setting up a GPU Instance on Amazon Web Services
Lecture 2: Installing NVIDIA GPU-Accelerated Deep Learning Libraries on your Home Computer
Lecture 3: Can Big Data be used to Speed Up Backpropagation?
Lecture 4: How to Improve your Theano and Tensorflow Skills
Lecture 5: Theano vs. TensorFlow
Chapter 10: Transition to the 2nd Half of the Course
Lecture 1: Transition to the 2nd Half of the Course
Chapter 11: Project: Facial Expression Recognition
Lecture 1: Facial Expression Recognition Project Introduction
Lecture 2: Facial Expression Recognition Problem Description
Lecture 3: The class imbalance problem
Lecture 4: Utilities walkthrough
Lecture 5: Class-Based ANN in Theano
Lecture 6: Class-Based ANN in TensorFlow
Lecture 7: Facial Expression Recognition Project Summary
Chapter 12: Modern Regularization Techniques
Lecture 1: Modern Regularization Techniques Section Introduction
Lecture 2: Dropout Regularization
Lecture 3: Dropout Intuition
Lecture 4: Noise Injection
Lecture 5: Modern Regularization Techniques Section Summary
Chapter 13: Batch Normalization
Lecture 1: Batch Normalization Introduction
Lecture 2: Exponentially-Smoothed Averages
Lecture 3: Batch Normalization Theory
Lecture 4: Batch Normalization Tensorflow (part 1)
Lecture 5: Batch Normalization Tensorflow (part 2)
Lecture 6: Batch Normalization Theano (part 1)
Lecture 7: Batch Normalization Theano (part 2)
Lecture 8: Noise Perspective
Lecture 9: Batch Normalization Summary
Chapter 14: Keras
Lecture 1: Keras Discussion
Lecture 2: Keras in Code
Lecture 3: Keras Functional API
Lecture 4: How to easily convert Keras into Tensorflow 2.0 code
Chapter 15: PyTorch
Lecture 1: PyTorch Basics
Lecture 2: PyTorch Dropout
Lecture 3: PyTorch Batch Norm
Chapter 16: PyTorch, CNTK, and MXNet
Lecture 1: PyTorch, CNTK, and MXNet
Chapter 17: Deep Learning Review Topics
Lecture 1: What's the difference between "neural networks" and "deep learning"?
Lecture 2: Manually Choosing Learning Rate and Regularization Penalty
Chapter 18: Setting Up Your Environment (FAQ by Student Request)
Lecture 1: Pre-Installation Check
Lecture 2: Anaconda Environment Setup
Lecture 3: How to install Numpy, Scipy, Matplotlib, Pandas, IPython, Theano, and TensorFlow
Chapter 19: Extra Help With Python Coding for Beginners (FAQ by Student Request)
Lecture 1: How to Code by Yourself (part 1)
Lecture 2: How to Code by Yourself (part 2)
Instructors
-
Lazy Programmer Inc.
Artificial intelligence and machine learning engineer
Rating Distribution
- 1 stars: 33 votes
- 2 stars: 44 votes
- 3 stars: 141 votes
- 4 stars: 1095 votes
- 5 stars: 2210 votes
Frequently Asked Questions
How long do I have access to the course materials?
You can view and review the lecture materials indefinitely, like an on-demand channel.
Can I take my courses with me wherever I go?
Definitely! If you have an internet connection, courses on Udemy are available on any device at any time. If you don’t have an internet connection, some instructors also let their students download course lectures. That’s up to the instructor though, so make sure you get on their good side!
You may also like
- Digital Marketing Foundation Course
- Google Shopping Ads Digital Marketing Course
- Multi Cloud Infrastructure for beginners
- Master Lead Generation: Grow Subscribers & Sales with Popups
- Complete Copywriting System : write to sell with ease
- Product Positioning Masterclass: Unlock Market Traction
- How to Promote Your Webinar and Get More Attendees?
- Digital Marketing Courses
- Create music with Artificial Intelligence in this new market
- Create CONVERTING UGC Content So Brands Will Pay You More
- Podcast: The top 8 ways to monetize by Podcasting
- TikTok Marketing Mastery: Learn to Grow & Go Viral
- Free Digital Marketing Basics Course in Hindi
- MailChimp Free Mailing Lists: MailChimp Email Marketing
- Automate Digital Marketing & Social Media with Generative AI
- Google Ads MasterClass – All Advanced Features
- Online Course Creator: Create & Sell Online Courses Today!
- Introduction to SEO – Basic Principles of SEO
- Affiliate Marketing For Beginners: Go From Novice To Pro
- Effective Website Planning Made Simple