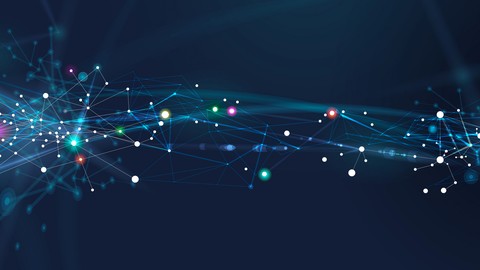
Data Science Projects with Python
Data Science Projects with Python, available at $59.99, has an average rating of 4.65, with 63 lectures, 6 quizzes, based on 17 reviews, and has 155 subscribers.
You will learn about Install the required packages to set up a data science coding environment Load data into a Jupyter Notebook running Python Use Matplotlib to create data visualizations Fit a model using scikit-learn Use lasso and ridge regression to reduce overfitting Fit and tune a random forest model and compare performance with logistic regression Create visuals using the output of the Jupyter Notebook This course is ideal for individuals who are If you are a data analyst, data scientist, or a business analyst who wants to get started with using Python and machine learning techniques to analyze data and predict outcomes, this book is for you. It is particularly useful for If you are a data analyst, data scientist, or a business analyst who wants to get started with using Python and machine learning techniques to analyze data and predict outcomes, this book is for you.
Enroll now: Data Science Projects with Python
Summary
Title: Data Science Projects with Python
Price: $59.99
Average Rating: 4.65
Number of Lectures: 63
Number of Quizzes: 6
Number of Published Lectures: 63
Number of Published Quizzes: 6
Number of Curriculum Items: 69
Number of Published Curriculum Objects: 69
Original Price: $199.99
Quality Status: approved
Status: Live
What You Will Learn
- Install the required packages to set up a data science coding environment
- Load data into a Jupyter Notebook running Python
- Use Matplotlib to create data visualizations
- Fit a model using scikit-learn
- Use lasso and ridge regression to reduce overfitting
- Fit and tune a random forest model and compare performance with logistic regression
- Create visuals using the output of the Jupyter Notebook
Who Should Attend
- If you are a data analyst, data scientist, or a business analyst who wants to get started with using Python and machine learning techniques to analyze data and predict outcomes, this book is for you.
Target Audiences
- If you are a data analyst, data scientist, or a business analyst who wants to get started with using Python and machine learning techniques to analyze data and predict outcomes, this book is for you.
Data Science Projects with Python is designed to give you practical guidance on industry-standard data analysis and machine learning tools in Python, with the help of realistic data. The course will help you understand how you can use pandas and Matplotlib to critically examine a dataset with summary statistics and graphs and extract the insights you seek to derive. You will continue to build on your knowledge as you learn how to prepare data and feed it to machine learning algorithms, such as regularized logistic regression and random forest, using the scikit-learn package. You’ll discover how to tune the algorithms to provide the best predictions on new and, unseen data.
As you delve into later chapters, you’ll be able to understand the working and output of these algorithms and gain insight into not only the predictive capabilities of the models but also their reasons for making these predictions.
About the Author
Stephen Klosterman is a machine learning data scientist at CVS Health. He enjoys helping to frame problems in a data science context and delivering machine learning solutions that business stakeholders understand and value. His education includes a Ph.D. in biology from Harvard University, where he was an assistant teacher of the data science course.
Barbora Stetinova works in an Automotive industry earned experience in data science and machine learning, leading small team, leading strategical projects and in controlling topics for 13 years. Since Sept 2018 she is a member of IT department participating on the Data science implementation in an automotive company.
In parallel, since Aug 2017, she is also engaged in strategical group projects for the automotive company and with side contract as an analytical external consultant for different industries (retail, sensorics, building) at Leadership Synergy Community. She is also a data science trainer for Elderberry data, specialized in MS Excel and Knime analytics platform in both face-to-face and elearning forms (available on Udemy).
Course Curriculum
Chapter 1: Data Exploration and Cleaning
Lecture 1: Course Overview
Lecture 2: Installation and Setup
Lecture 3: Lesson Overview
Lecture 4: Python and the Anaconda Package Management System
Lecture 5: Different Types of Data Science Problems
Lecture 6: Loading the Case Study Data with Jupyter and pandas
Lecture 7: Getting Familiar with Data and Performing Data Cleaning
Lecture 8: Boolean Masks
Lecture 9: Data Quality Assurance and Exploration
Lecture 10: Deep Dive: Categorical Features
Lecture 11: Exploring the Financial History Features in the Dataset
Lecture 12: Lesson Summary
Lecture 13: Actitvity 1 : Exploring Remaining Financial Features in the Dataset
Lecture 14: Solution 1 : Exploring Remaining Financial Features in the Dataset
Chapter 2: Introduction to Scikit-Learn and Model Evaluation
Lecture 1: Lesson Overview
Lecture 2: Exploring the Response Variable and Concluding the Initial Exploration
Lecture 3: Introduction to Scikit-Learn
Lecture 4: Model Performance Metrics for Binary Classification
Lecture 5: True Positive Rate, False Positive Rate, and Confusion Matrix
Lecture 6: Obtaining Predicted Probabilities from a Trained Logistic Regression Model
Lecture 7: Lesson Summary
Lecture 8: Activity 2: Performing Logistic Regression and Creating a Precision-Recall Curve
Lecture 9: Solution 2: Performing Logistic Regression and Creating a Precision-Recall Curve
Chapter 3: Details of Logistic Regression and Feature Exploration
Lecture 1: Lesson Overview
Lecture 2: Examining the Relationships between Features and the Response
Lecture 3: Finer Points of the F-test: Equivalence to t-test for Two Classes and Cautions
Lecture 4: Univariate Feature Selection: What It Does and Doesn't Do
Lecture 5: Generalized Linear Models (GLMs)
Lecture 6: Lesson Summary
Lecture 7: Activity 3: Fitting a Logistic Regression Model & Directly Using the Coefficient
Lecture 8: Solution 3: Fitting a Logistic Regression Model & Directly Using the Coefficient
Chapter 4: The Bias-Variance Trade-off
Lecture 1: Lesson Overview
Lecture 2: Estimating the Coefficients and Intercepts of Logistic Regression
Lecture 3: Assumptions of Logistic Regression
Lecture 4: How Many Features Should You Include?
Lecture 5: Lasso (L1) and Ridge (L2) Regularization
Lecture 6: Cross Validation: Choosing the Regularization Parameter and Other Hyperparameter
Lecture 7: Reducing Overfitting on the Synthetic Data Classification Problem
Lecture 8: Options for Logistic Regression in Scikit-Learn
Lecture 9: Lesson Summary
Lecture 10: Activity 4: Cross-Validation and Feature Engineering with the Case Study Data
Lecture 11: Solution 4: Cross-Validation and Feature Engineering with the Case Study Data
Chapter 5: Decision Trees and Random Forests
Lecture 1: Lesson Overview
Lecture 2: Decision Trees
Lecture 3: Training Decision Trees: Node Impurity
Lecture 4: Using Decision Trees: Advantages and Predicted Probabilities
Lecture 5: Random Forests: Ensembles of Decision Trees
Lecture 6: Fitting a Random Forest
Lecture 7: Lesson Summary
Lecture 8: Activity 5: Cross-Validation Grid Search with Random Forest
Lecture 9: Solution 5: Cross-Validation Grid Search with Random Forest
Chapter 6: Imputation of Missing Data, Financial Analysis, and Delivery to Client
Lecture 1: Lesson Overview
Lecture 2: Review of Modeling Results
Lecture 3: Dealing with Missing Data: Imputation Strategies
Lecture 4: Cleaning the Dataset
Lecture 5: Mode and Random Imputation of PAY_1
Lecture 6: A Predictive Model for PAY_1
Lecture 7: Using the Imputation Model and Comparing it to Other Methods
Lecture 8: Financial Analysis
Lecture 9: Final Thoughts on Delivering the Predictive Model to the Client
Lecture 10: Lesson Summary
Lecture 11: Activity 6: Deriving Financial Insights
Lecture 12: Solution 6: Deriving Financial Insights
Instructors
-
Packt Publishing
Tech Knowledge in Motion
Rating Distribution
- 1 stars: 1 votes
- 2 stars: 0 votes
- 3 stars: 3 votes
- 4 stars: 3 votes
- 5 stars: 10 votes
Frequently Asked Questions
How long do I have access to the course materials?
You can view and review the lecture materials indefinitely, like an on-demand channel.
Can I take my courses with me wherever I go?
Definitely! If you have an internet connection, courses on Udemy are available on any device at any time. If you don’t have an internet connection, some instructors also let their students download course lectures. That’s up to the instructor though, so make sure you get on their good side!
You may also like
- Best Cybersecurity Fundamentals Courses to Learn in March 2025
- Best Smart Home Technology Courses to Learn in March 2025
- Best Holistic Health Courses to Learn in March 2025
- Best Nutrition And Diet Planning Courses to Learn in March 2025
- Best Yoga Instruction Courses to Learn in March 2025
- Best Stress Management Courses to Learn in March 2025
- Best Mindfulness Meditation Courses to Learn in March 2025
- Best Life Coaching Courses to Learn in March 2025
- Best Career Development Courses to Learn in March 2025
- Best Relationship Building Courses to Learn in March 2025
- Best Parenting Skills Courses to Learn in March 2025
- Best Home Improvement Courses to Learn in March 2025
- Best Gardening Courses to Learn in March 2025
- Best Sewing And Knitting Courses to Learn in March 2025
- Best Interior Design Courses to Learn in March 2025
- Best Writing Courses Courses to Learn in March 2025
- Best Storytelling Courses to Learn in March 2025
- Best Creativity Workshops Courses to Learn in March 2025
- Best Resilience Training Courses to Learn in March 2025
- Best Emotional Intelligence Courses to Learn in March 2025