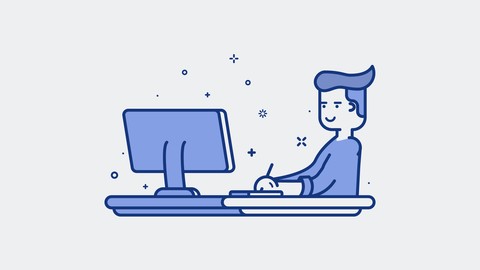
Learning Path: OpenCV: Real-Time Computer Vision with OpenCV
Learning Path: OpenCV: Real-Time Computer Vision with OpenCV, available at $34.99, has an average rating of 4.45, with 62 lectures, based on 74 reviews, and has 707 subscribers.
You will learn about Learn about the computer vision workflows and understand the basic image matrix format and filters Understand the segmentation and feature extraction techniques Learn how to remove backgrounds from a static scene to identify moving objects for video surveillance Use the new OpenCV functions for text detection and recognition with Tesseract Master logistic regression and regularization techniques Solve image segmentation problem using k-means clustering Load models trained with popular deep learning libraries such as Caffe This course is ideal for individuals who are This Learning Path is for developers who have a basic understanding of computer vision and image processing and want to develop interesting computer vision applications with OpenCV. It is particularly useful for This Learning Path is for developers who have a basic understanding of computer vision and image processing and want to develop interesting computer vision applications with OpenCV.
Enroll now: Learning Path: OpenCV: Real-Time Computer Vision with OpenCV
Summary
Title: Learning Path: OpenCV: Real-Time Computer Vision with OpenCV
Price: $34.99
Average Rating: 4.45
Number of Lectures: 62
Number of Published Lectures: 62
Number of Curriculum Items: 62
Number of Published Curriculum Objects: 62
Original Price: $199.99
Quality Status: approved
Status: Live
What You Will Learn
- Learn about the computer vision workflows and understand the basic image matrix format and filters
- Understand the segmentation and feature extraction techniques
- Learn how to remove backgrounds from a static scene to identify moving objects for video surveillance
- Use the new OpenCV functions for text detection and recognition with Tesseract
- Master logistic regression and regularization techniques
- Solve image segmentation problem using k-means clustering
- Load models trained with popular deep learning libraries such as Caffe
Who Should Attend
- This Learning Path is for developers who have a basic understanding of computer vision and image processing and want to develop interesting computer vision applications with OpenCV.
Target Audiences
- This Learning Path is for developers who have a basic understanding of computer vision and image processing and want to develop interesting computer vision applications with OpenCV.
Are you looking forward to developing interesting computer vision applications? If yes, then this Learning Path is for you.
Packt’s Video Learning Paths are a series of individual video products put together in a logical and stepwise manner such that each video builds on the skills learned in the video before it.
Computer vision and machine learning concepts are frequently used together in practical projects based on computer vision. Whether you are completely new to the concept of computer vision or have a basic understanding of it, this Learning Path will be your guide to understanding the basic OpenCV concepts and algorithms through amazing real-world examples and projects.
OpenCV is a cross-platform, open source library that is used for face recognition, object tracking, and image and video processing. By learning the basic concepts of computer vision algorithms, models, and OpenCV’s API, you will be able to develop different types of real-world applications.
Starting from the installation of OpenCV on your system and understanding the basics of image processing, we swiftly move on to creating optical flow video analysis and text recognition in complex scenes. You’ll explore the commonly used computer vision techniques to build your own OpenCV projects from scratch. Next, we’ll teach you how to work with the various OpenCV modules for statistical modeling and machine learning. You’ll start by preparing your data for analysis, learn about supervised and unsupervised learning, and see how to use them. Finally, you’ll learn to implement efficient models using the popular machine learning techniques such as classification, regression, decision trees, K-nearest neighbors, boosting, and neural networks with the aid of C++ and OpenCV.
By the end of this Learning Path, you will be familiar with the basics of OpenCV such as matrix operations, filters, and histograms, as well as more advanced concepts such as segmentation, machine learning, complex video analysis, and text recognition.
Meet Your Experts:
We have combined the best works of the following esteemed authors to ensure that your learning journey is smooth:
David Millán Escriváwas eight years old when he wrote his first program on an 8086 PC with Basic language, which enabled the 2D plotting of basic equations. In 2005, he finished his studies in IT through the Universitat Politécnica de Valencia with honors in human-computer interaction supported by computer vision with OpenCV (v0.96).
Prateek Joshi is an artificial intelligence researcher, published author of five books, and TEDx speaker. He is the founder of Pluto AI, a venture-funded Silicon Valley startup building an analytics platform for smart water management powered by deep learning.
Joe Minichino is a computer vision engineer for Hoolux Medical by day and a developer of the NoSQL database LokiJS by night. At Hoolux, he leads the development of an Android computer vision-based advertising platform for the medical industry.
Course Curriculum
Chapter 1: OpenCV 3 by Example
Lecture 1: The Course Overview
Lecture 2: The Human Visual System and Understanding Image Content
Lecture 3: What Can You Do with OpenCV?
Lecture 4: Installing OpenCV
Lecture 5: Basic CMakeConfiguration and Creating a Library
Lecture 6: Managing Dependencies
Lecture 7: Making the Script More Complex
Lecture 8: Images and Matrices
Lecture 9: Reading/Writing Images
Lecture 10: Reading Videos and Cameras
Lecture 11: Other Basic Object Types
Lecture 12: Basic Matrix Operations, Data Persistence, and Storage
Lecture 13: The OpenCVUser Interface and a Basic GUI
Lecture 14: The Graphical User Interface with QT
Lecture 15: Adding Slider and Mouse Events to Our Interfaces
Lecture 16: Adding Buttons to a User Interface
Lecture 17: OpenGL Support
Lecture 18: Generating a CMakeScript File
Lecture 19: Creating the Graphical User Interface
Lecture 20: Drawing a Histogram
Lecture 21: Image Color Equalization
Lecture 22: Lomography Effect
Lecture 23: The CartoonizeEffect
Lecture 24: Isolating Objects in a Scene
Lecture 25: Creating an Application for AOI
Lecture 26: Preprocessing the Input Image
Lecture 27: Segmenting Our Input Image
Lecture 28: Introducing Machine Learning Concepts
Lecture 29: Computer Vision and the Machine Learning Workflow
Lecture 30: Automatic Object Inspection Classification Example
Lecture 31: Feature Extraction
Lecture 32: Understanding Haar Cascades
Lecture 33: What Are Integral Images
Lecture 34: Overlaying a Facemask in a Live Video
Lecture 35: Get Your Sunglasses On
Lecture 36: Tracking Your Nose, Mouth, and Ears
Lecture 37: Background Subtraction
Lecture 38: Frame Differencing
Lecture 39: The Mixture of Gaussians Approach
Lecture 40: Morphological Image processing
Lecture 41: Other Morphological Operators
Lecture 42: Tracking Objects of a Specific Color
Lecture 43: Building an Interactive Object Tracker
Lecture 44: Detecting Points Using the Harris Corner Detector
Lecture 45: Shi-Tomasi Corner Detector
Lecture 46: Feature-Based Tracking
Lecture 47: Introducing Optical Character Recognition
Lecture 48: The Preprocessing Step
Lecture 49: Installing Tesseract OCR on Your Operating System
Lecture 50: Using Tesseract OCR Library
Chapter 2: Machine Learning with Open CV and Python
Lecture 1: The Course Overview
Lecture 2: The Basics of Machine Learning
Lecture 3: Creating Training Data and Extracting Information
Lecture 4: Extracting Features
Lecture 5: K-Nearest Neighbors
Lecture 6: Logistic Regression
Lecture 7: Normal Bayes Classifier
Lecture 8: Decision Trees
Lecture 9: Support Vector Machines
Lecture 10: Artificial Neural Networks
Lecture 11: Unsupervised Learning
Lecture 12: Deep Learning
Instructors
-
Packt Publishing
Tech Knowledge in Motion
Rating Distribution
- 1 stars: 6 votes
- 2 stars: 6 votes
- 3 stars: 11 votes
- 4 stars: 24 votes
- 5 stars: 27 votes
Frequently Asked Questions
How long do I have access to the course materials?
You can view and review the lecture materials indefinitely, like an on-demand channel.
Can I take my courses with me wherever I go?
Definitely! If you have an internet connection, courses on Udemy are available on any device at any time. If you don’t have an internet connection, some instructors also let their students download course lectures. That’s up to the instructor though, so make sure you get on their good side!
You may also like
- Digital Marketing Foundation Course
- Google Shopping Ads Digital Marketing Course
- Multi Cloud Infrastructure for beginners
- Master Lead Generation: Grow Subscribers & Sales with Popups
- Complete Copywriting System : write to sell with ease
- Product Positioning Masterclass: Unlock Market Traction
- How to Promote Your Webinar and Get More Attendees?
- Digital Marketing Courses
- Create music with Artificial Intelligence in this new market
- Create CONVERTING UGC Content So Brands Will Pay You More
- Podcast: The top 8 ways to monetize by Podcasting
- TikTok Marketing Mastery: Learn to Grow & Go Viral
- Free Digital Marketing Basics Course in Hindi
- MailChimp Free Mailing Lists: MailChimp Email Marketing
- Automate Digital Marketing & Social Media with Generative AI
- Google Ads MasterClass – All Advanced Features
- Online Course Creator: Create & Sell Online Courses Today!
- Introduction to SEO – Basic Principles of SEO
- Affiliate Marketing For Beginners: Go From Novice To Pro
- Effective Website Planning Made Simple