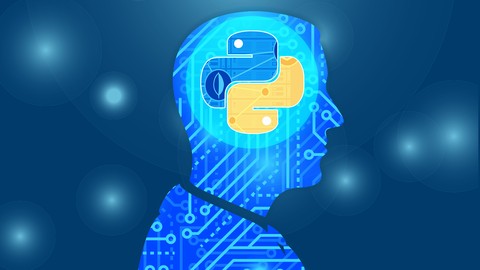
Machine Learning with Python from Scratch
Machine Learning with Python from Scratch, available at $54.99, has an average rating of 4.35, with 64 lectures, based on 329 reviews, and has 4622 subscribers.
You will learn about Have an understand of Machine Learning and how to apply it in your own programs Understand and be able to use Pythons main scientific libraries for Data analysis – Numpy, Pandas, Matplotlib and Seaborn. Understand and be able to use artificial neural networks Obtain a solid understand of machine learning in general Potential for a new job in the future. This course is ideal for individuals who are Students who wish to take their basic Python skills to the next level by mastering Pythons various scientific libraries or Students who want to understand and apply Machine Learning into their own programs or Students wanting to empower themselves with machine learning. It is particularly useful for Students who wish to take their basic Python skills to the next level by mastering Pythons various scientific libraries or Students who want to understand and apply Machine Learning into their own programs or Students wanting to empower themselves with machine learning.
Enroll now: Machine Learning with Python from Scratch
Summary
Title: Machine Learning with Python from Scratch
Price: $54.99
Average Rating: 4.35
Number of Lectures: 64
Number of Published Lectures: 64
Number of Curriculum Items: 64
Number of Published Curriculum Objects: 64
Original Price: $69.99
Quality Status: approved
Status: Live
What You Will Learn
- Have an understand of Machine Learning and how to apply it in your own programs
- Understand and be able to use Pythons main scientific libraries for Data analysis – Numpy, Pandas, Matplotlib and Seaborn.
- Understand and be able to use artificial neural networks
- Obtain a solid understand of machine learning in general
- Potential for a new job in the future.
Who Should Attend
- Students who wish to take their basic Python skills to the next level by mastering Pythons various scientific libraries
- Students who want to understand and apply Machine Learning into their own programs
- Students wanting to empower themselves with machine learning.
Target Audiences
- Students who wish to take their basic Python skills to the next level by mastering Pythons various scientific libraries
- Students who want to understand and apply Machine Learning into their own programs
- Students wanting to empower themselves with machine learning.
Machine Learning is a hot topic! Python Developers who understand how to work with Machine Learning are in high demand.
But how do you get started?
Maybe you tried to get started with Machine Learning, but couldn’t find decent tutorials online to bring you up to speed, fast.
Maybe the information you found was too basic, and didn’t give you the real-world Machine learning skills using Python that you needed.
Or maybe the information got bogged down in complex math explanations and was too difficult to relate to.
Whatever the reason, you are in the right place if you want to progress your skills in Machine Language using Python.
This course will help you to understand the main machine learning algorithms using Python, and how to apply them in your own projects.
But what exactly is Machine Learning?
It’s a field of computer science that gives computers the ability to “learn” – e.g. continually improve performance on a specific task, with data, without being explicitly programmed.
Why is it important?
Machine learning is often used to solve tasks considered too complex for humans to solve. We create algorithms and apply a bunch of data to that algorithm and let the computer process (execute) the algorithm and search for a model (solution).
Because of the practical applications of machine learning, such as self driving cars (one example) there is huge interest from companies and government in Machine learning, and as a result, there are a a lot of opportunities for Python developers who are skilled in this field.
If you want to increase your career options, then understanding and being able to work with Machine Learning with your own Python programs should be high on your list of priorities.
What will you learn in this course?
For starters, you will learn about the main scientific libraries in Python for data analysis such as Numpy, Pandas, Matplotlib and Seaborn.
You’ll then learn about artificial neural networks and how to work with machine learning models using them.
You obtain a solid background in machine learning and be able to apply that knowledge directly in your own programs.
What are the Main topics included in the course?
Data Analysis with Numpy, Pandas, Matplotlib and Seaborn.
The machine learning schema.
Overfitting and Underfitting
K Fold Cross Validation
Classification metrics
Regularization: Lasso, Ridge and ElasticNet
Logistic Regression
Support Vector Machines for Regression and Classification
Naive Bayes Classifier
Decision Trees and Random Forest
KNN classifier
Hyperparameter Optimization: GridSearchCV
Principal Component Analysis (PCA)
Linear Discriminant Analysis (LDA)
Kernel Principal Component Analysis (KPCA)
Ensemble methods: Bagging
AdaBoost
K means clustering analysis
Regression model and evaluation
Linear and Polynomial Regression
SVM, KNN, and Random Forest for Regression
RANSAC Regression
Neural Networks: Constructing our own MLP.
Perceptron and Multilayer Perceptron
And don’t worry if you do not understand some, or all of these terms. By the end of the course you will know what they are and how to use them.
Why enrolling in this course is the best decision you can make.
This course helps you to understand the difficult concepts of Machine learning in a unique way. Rather than just focusing on complex maths explanaitons, simpler explanations with charts, and info displays are included.
Many examples and genuinely useful code snippets are also included to make it even easier to learn and understand.
After completing this course, you will have the necessary skills to apply Machine learning in your own projects.
The sooner you sign up for this course, the sooner you will have the skills and knowledge you need to increase your job or consulting opportunities. Your new job or consulting opportunity awaits! �
Why not get started today?
Click the Signup button to sign up for the course!
Course Curriculum
Chapter 1: Environment Setup
Lecture 1: L1-Anaconda
Lecture 2: L2-Jupyter Notebook
Chapter 2: Data Analysis
Lecture 1: L1-Introduction
Lecture 2: L2-Numpy: Array Concept and Math Operations
Lecture 3: L3-Numpy: Indexing, Slicing and Iterating
Lecture 4: L4-Numpy: Shape Manipulation
Lecture 5: L5-Numpy: Linear Algebra
Lecture 6: L6-Pandas: Data structures and properties
Lecture 7: L7-Pandas: Operations
Lecture 8: L8-Pandas: Applying Functions
Lecture 9: L9-Pandas: Importing and Exporting data
Lecture 10: L10-Pandas: Merge-Join-Concat-Group by
Lecture 11: L11-Pandas: Statistics with Pandas
Lecture 12: L12-Time Series with Pandas
Lecture 13: L13-Matplotlib basics
Lecture 14: L14-Matplotlib Subplots and Axes
Lecture 15: L15-Matplotlib: Object Oriented Method
Lecture 16: L16-Matplotlib: Color Maps
Lecture 17: L17-Matplotlib: Statistical Graphs part1
Lecture 18: L18-Matplotlib: Statistical Graphs part2
Lecture 19: L19-Seaborn: Basics
Lecture 20: L20-Seaborn: Color Palette
Lecture 21: L21-Seaborn: Categorical Data
Lecture 22: L22-Seaborn: Numerical Data
Chapter 3: Machine Learning
Lecture 1: L1-Introduction to Machine Learning
Lecture 2: L2-Overfitting and Underfitting
Lecture 3: L3-KFold Cross Validation
Lecture 4: L4-Classification Metrics
Lecture 5: L5-Logistic Regression
Lecture 6: L6-Plotting Decision Boundaries
Lecture 7: L7-Naive Bayes Classifier
Lecture 8: L8-Suppor Vector Machines for Classification
Lecture 9: L9-Decision Trees
Lecture 10: L10-Random Forest
Lecture 11: L11-KNN
Lecture 12: L12-GridSearchCV
Lecture 13: L13-K-Means
Lecture 14: L14-Principal Component Analysis(PCA)
Lecture 15: L15-Linear Discriminant Analysis(LDA)
Lecture 16: L16-Kernel Principal Component Analysis(KPCA)
Lecture 17: L17-Ensemble Methods(Bagging)
Lecture 18: L18-AdaBoost
Lecture 19: L19-Regression Model and Metrics
Lecture 20: L20-Linear Regression
Lecture 21: L21-Regularization with Lasso, Ridge and ElasticNet
Lecture 22: L22-Polynomial Regression
Lecture 23: L23-SVM, KNN and Random Forest for Regression
Lecture 24: L24-RANSAC Regression
Chapter 4: Neural Networks
Lecture 1: L1-Neural Networks Concepts-Part 1
Lecture 2: L2-Neural Networks Concepts-Part 2
Lecture 3: L3-Loss Functions
Lecture 4: L4-Activation Functions
Lecture 5: L5-Optimization of ANNs
Lecture 6: L6-Constructing an ANN with Python-part1
Lecture 7: L7-Constructing an ANN with Python-part2
Lecture 8: L8-Constructing an ANN with Python-part3
Lecture 9: L9-Perceptron with Scikit Learn
Lecture 10: L10-Multilayer Perceptron with Scikit Learn
Chapter 5: Applications
Lecture 1: L1-Datasets
Lecture 2: L2-ANN for Regression Part 1
Lecture 3: L3-ANN for Regression Part 2
Lecture 4: L4-Recognizing Handwritten Digits
Chapter 6: Extra Information – Source code, and other stuff
Lecture 1: Source Code
Lecture 2: Bonus Lecture and Information
Instructors
-
Tim Buchalka's Learn Programming Academy
Professional Programmers and Teachers – 2M students -
CARLOS QUIROS
Industrial Engineer and Data Scientist
Rating Distribution
- 1 stars: 18 votes
- 2 stars: 16 votes
- 3 stars: 58 votes
- 4 stars: 131 votes
- 5 stars: 106 votes
Frequently Asked Questions
How long do I have access to the course materials?
You can view and review the lecture materials indefinitely, like an on-demand channel.
Can I take my courses with me wherever I go?
Definitely! If you have an internet connection, courses on Udemy are available on any device at any time. If you don’t have an internet connection, some instructors also let their students download course lectures. That’s up to the instructor though, so make sure you get on their good side!
You may also like
- Best Video Editing Courses to Learn in March 2025
- Best Music Production Courses to Learn in March 2025
- Best Animation Courses to Learn in March 2025
- Best Digital Illustration Courses to Learn in March 2025
- Best Renewable Energy Courses to Learn in March 2025
- Best Sustainable Living Courses to Learn in March 2025
- Best Ethical AI Courses to Learn in March 2025
- Best Cybersecurity Fundamentals Courses to Learn in March 2025
- Best Smart Home Technology Courses to Learn in March 2025
- Best Holistic Health Courses to Learn in March 2025
- Best Nutrition And Diet Planning Courses to Learn in March 2025
- Best Yoga Instruction Courses to Learn in March 2025
- Best Stress Management Courses to Learn in March 2025
- Best Mindfulness Meditation Courses to Learn in March 2025
- Best Life Coaching Courses to Learn in March 2025
- Best Career Development Courses to Learn in March 2025
- Best Relationship Building Courses to Learn in March 2025
- Best Parenting Skills Courses to Learn in March 2025
- Best Home Improvement Courses to Learn in March 2025
- Best Gardening Courses to Learn in March 2025