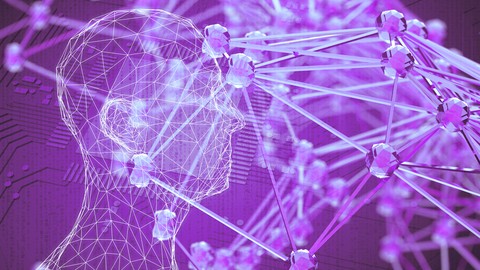
PyTorch: Deep Learning with PyTorch – Masterclass!: 2-in-1
PyTorch: Deep Learning with PyTorch – Masterclass!: 2-in-1, available at $59.99, has an average rating of 4.25, with 65 lectures, 1 quizzes, based on 74 reviews, and has 592 subscribers.
You will learn about Build your neural network using Deep Learning techniques in PyTorch. Build artificial neural networks in Python with GPU acceleration. Use Auto-Encoders in PyTorch to remove noise from images. Perform Reinforcement Learning to solve OpenAI'sCartpole task. Extend your knowledge of Deep Learning by using PyTorch to solve your own machine learning problems. Create a Convolutional Neural Network (CNN) for image recognition. Predict share prices with Recurrent Neural Network and Long Short-Term Memory Network (LSTM). Detect credit card fraud with autoencoders. Develop a movie recommendation system using Boltzmann Machines. Use AutoEncoders to develop recommendation systems to rate a movie. Detect the shape and color of a given picture or an object using PyTorch This course is ideal for individuals who are Python programmers, Data Science professionals who would like to practically implement PyTorch and explore its unique features in their Deep Learning projects. It is particularly useful for Python programmers, Data Science professionals who would like to practically implement PyTorch and explore its unique features in their Deep Learning projects.
Enroll now: PyTorch: Deep Learning with PyTorch – Masterclass!: 2-in-1
Summary
Title: PyTorch: Deep Learning with PyTorch – Masterclass!: 2-in-1
Price: $59.99
Average Rating: 4.25
Number of Lectures: 65
Number of Quizzes: 1
Number of Published Lectures: 65
Number of Published Quizzes: 1
Number of Curriculum Items: 66
Number of Published Curriculum Objects: 66
Original Price: $199.99
Quality Status: approved
Status: Live
What You Will Learn
- Build your neural network using Deep Learning techniques in PyTorch.
- Build artificial neural networks in Python with GPU acceleration.
- Use Auto-Encoders in PyTorch to remove noise from images.
- Perform Reinforcement Learning to solve OpenAI'sCartpole task.
- Extend your knowledge of Deep Learning by using PyTorch to solve your own machine learning problems.
- Create a Convolutional Neural Network (CNN) for image recognition.
- Predict share prices with Recurrent Neural Network and Long Short-Term Memory Network (LSTM).
- Detect credit card fraud with autoencoders.
- Develop a movie recommendation system using Boltzmann Machines.
- Use AutoEncoders to develop recommendation systems to rate a movie.
- Detect the shape and color of a given picture or an object using PyTorch
Who Should Attend
- Python programmers, Data Science professionals who would like to practically implement PyTorch and explore its unique features in their Deep Learning projects.
Target Audiences
- Python programmers, Data Science professionals who would like to practically implement PyTorch and explore its unique features in their Deep Learning projects.
PyTorch: written in Python, is grabbing the attention of all data science professionals due to its ease of use over other libraries and its use of dynamic computation graphs.
PyTorch is a Deep Learning framework that is a boon for researchers and data scientists. It supports Graphic Processing Units and is a platform that provides maximum flexibility and speed. With PyTorch, you can dynamically build neural networks and easily perform advanced Artificial Intelligence tasks.
This comprehensive 2-in-1 course takes a practical approach and is filled with real-world examples to help you create your own application using PyTorch! Begin with exploring PyTorch and the impact it has made on Deep Learning. Design and implement powerful neural networks to solve some impressive problems in a step-by-step manner. Build a Convolutional Neural Network (CNN) for image recognition. Also, predict share prices with Recurrent Neural Network and Long Short-Term Memory Network (LSTM). You’ll learn how to detect credit card fraud with autoencoders and much more!
By the end of the course, you’ll conquer the world of PyTorch to build useful and effective Deep Learning models with the PyTorch Deep Learning framework with the help of real-world examples!
Contents and Overview
This training program includes 2 complete courses, carefully chosen to give you the most comprehensive training possible.
The first course, Deep Learning with PyTorch, covers building useful and effective deep learning models with the PyTorch Deep Learning framework. In this course, you will learn how to accomplish useful tasks using Convolutional Neural Networks to process spatial data such as images and using Recurrent Neural Networks to process sequential data such as texts. You will explore how you can make use of unlabeled data using Auto-Encoders. You will also be training a neural network to learn how to balance a pole all by itself, using Reinforcement Learning. Throughout this journey, you will implement various mechanisms of the PyTorch framework to do these tasks. By the end of the video course, you will have developed a good understanding of, and feeling for, the algorithms and techniques used. You’ll have a good knowledge of how PyTorch works and how you can use it in to solve your daily machine learning problems.
The second course, Deep Learning Projects with PyTorch, covers creating deep learning models with the help of real-world examples. The course starts with the fundamentals of PyTorch and how to use basic commands. Next, you’ll learn about Convolutional Neural Networks (CNN) through an example of image recognition, where you’ll look into images from a machine perspective. The next project shows you how to predict character sequence using Recurrent Neural Networks (RNN) and Long Short Term Memory Network (LSTM). Then you’ll learn to work with autoencoders to detect credit card fraud. After that, it’s time to develop a system using Boltzmann Machines, where you’ll recommend whether to watch a movie or not. By the end of the course, you’ll be able to start using PyTorch to build Deep Learning models by implementing practical projects in the real world. So, grab this course as it will take you through interesting real-world projects to train your first neural nets.
By the end of the course, you’ll conquer the world of PyTorch to build useful and effective Deep Learning models with the PyTorch Deep Learning framework!
About the Authors
-
AnandSahais a software professional with 15 years’ experience in developing enterprise products and services. Back in 2007, he worked with machine learning to predict call patterns at TATA Communications. At Symantec and Veritas, he worked on various features of an enterprise backup product used by Fortune 500 companies. Along the way, he nurtured his interests in Deep Learning by attending Coursera and Udacity MOOCs. He is passionate about Deep Learning and its applications; so much so that he quit Veritas at the beginning of 2017 to focus full time on Deep Learning practices. Anand built pipelines to detect and count endangered species from aerial images, trained a robotic arm to pick and place objects, and implemented NIPS papers. His interests lie in computer vision and model optimization.
-
AshishSingh Bhatia is a learner, reader, seeker, and developer at the core. He has over 10 years of IT experience in different domains, including banking, ERP, and education. He is persistently passionate about Python, Java, R, and web and mobile development. He is always ready to explore new technologies.
Course Curriculum
Chapter 1: Deep Learning with PyTorch
Lecture 1: The Course Overview
Lecture 2: Introduction to PyTorch
Lecture 3: Installing PyTorch on Linux and Windows
Lecture 4: Installing CUDA
Lecture 5: Introduction to Tensors and Variables
Lecture 6: Working with PyTorch and NumPy
Lecture 7: Working with PyTorch and GPU
Lecture 8: Handling Datasets in PyTorch
Lecture 9: Deep Learning Using PyTorch
Lecture 10: Building a Simple Neural Network
Lecture 11: Loss Functions in PyTorch
Lecture 12: Optimizers in PyTorch
Lecture 13: Training the Neural Network
Lecture 14: Saving and Loading a Trained Neural Network
Lecture 15: Training the Neural Network on a GPU
Lecture 16: Computer Vision Motivation
Lecture 17: Convolutional Neural Networks
Lecture 18: The Convolution Operation
Lecture 19: Concepts – Strides, Padding, and Pooling
Lecture 20: Loading and Using MNIST Dataset
Lecture 21: Building the Model
Lecture 22: Training and Testing
Lecture 23: Sequence Models Motivation
Lecture 24: Word Embedding
Lecture 25: Recurrent Neural Networks
Lecture 26: Building a Text Generation Model in PyTorch
Lecture 27: Training and Testing
Lecture 28: Autoencoders Motivation
Lecture 29: How Autoencoders Work
Lecture 30: Types of Autoencoders
Lecture 31: Building Denoising Autoencoder Using PyTorch
Lecture 32: Training and Testing
Lecture 33: Reinforcement Learning Motivation
Lecture 34: Reinforcement Learning Concepts
Lecture 35: DQN, Experience Replay
Lecture 36: The OpenAI Gym Environment
Lecture 37: Building the Cartpole Agent Using DQN
Lecture 38: Training and Testing
Chapter 2: Deep Learning Projects with PyTorch
Lecture 1: The Course Overview
Lecture 2: Using PyTorch
Lecture 3: Understanding Regression
Lecture 4: Linear Regression and Logistic Regression
Lecture 5: Understanding Convolutional Neural Network
Lecture 6: Looking into Images from a Machine Perspective
Lecture 7: Making CNN
Lecture 8: Pooling Layers
Lecture 9: Output Layer
Lecture 10: Understanding Recurrent Neural Network
Lecture 11: Making RNN for Prediction
Lecture 12: Why LSTM?
Lecture 13: Moving to LSTM
Lecture 14: Getting Ready with Data
Lecture 15: Developing a Model
Lecture 16: Getting Output
Lecture 17: Introduction to Boltzmann Machines
Lecture 18: Getting Ready for Recommender System
Lecture 19: Making Boltzmann Machines
Lecture 20: Getting Output
Lecture 21: Introduction to Autoencoders
Lecture 22: Getting Ready for Recommender System
Lecture 23: Making Autoencoders
Lecture 24: Getting Output
Lecture 25: Getting Ready with Data
Lecture 26: Developing a Model
Lecture 27: Getting Output
Instructors
-
Packt Publishing
Tech Knowledge in Motion
Rating Distribution
- 1 stars: 0 votes
- 2 stars: 6 votes
- 3 stars: 19 votes
- 4 stars: 24 votes
- 5 stars: 25 votes
Frequently Asked Questions
How long do I have access to the course materials?
You can view and review the lecture materials indefinitely, like an on-demand channel.
Can I take my courses with me wherever I go?
Definitely! If you have an internet connection, courses on Udemy are available on any device at any time. If you don’t have an internet connection, some instructors also let their students download course lectures. That’s up to the instructor though, so make sure you get on their good side!
You may also like
- Best Public Speaking Courses to Learn in March 2025
- Best Affiliate Marketing Courses to Learn in March 2025
- Best Email Marketing Courses to Learn in March 2025
- Best Social Media Management Courses to Learn in March 2025
- Best SEO Optimization Courses to Learn in March 2025
- Best Content Creation Courses to Learn in March 2025
- Best Game Development Courses to Learn in March 2025
- Best Software Testing Courses to Learn in March 2025
- Best Big Data Courses to Learn in March 2025
- Best Internet Of Things Courses to Learn in March 2025
- Best Quantum Computing Courses to Learn in March 2025
- Best Cloud Computing Courses to Learn in March 2025
- Best 3d Modeling Courses to Learn in March 2025
- Best Mobile App Development Courses to Learn in March 2025
- Best Graphic Design Courses to Learn in March 2025
- Best Videography Courses to Learn in March 2025
- Best Photography Courses to Learn in March 2025
- Best Language Learning Courses to Learn in March 2025
- Best Product Management Courses to Learn in March 2025
- Best Investing Courses to Learn in March 2025