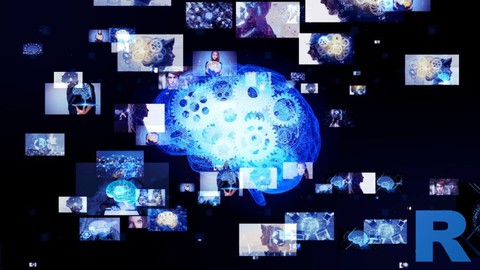
R Ultimate 2024: R for Data Science and Machine Learning
R Ultimate 2024: R for Data Science and Machine Learning, available at $79.99, has an average rating of 4.83, with 204 lectures, based on 193 reviews, and has 2811 subscribers.
You will learn about learn all aspects of R from Basics, over Data Science, to Machine Learning and Deep Learning learn R basics (data types, structures, variables, and more) learn R programming (writing loops, functions, and more) data im- and export basic data manipulation (piping, filtering, aggregation of results, data reshaping, set operations, joining datasets) data visualisation (different packages are learned, e.g. ggplot, plotly, leaflet, dygraphs) advanced data manipulation (outlier detection, missing data handling, regular expressions) regression models (create and apply regression models) model evaluation (What is underfitting and overfitting? Why is data splitted into training and testing? What are resampling techniques?) regularization (What is regularization? How can you apply it?) classification models (understand different algorithms and learn how to apply logistic regression, decision trees, random forests, support vector machines) association rules (learn the apriori model) clustering (kmeans, hierarchical clustering, DBscan) dimensionality reduction (factor analysis, principal component analysis) Reinforcement Learning (upper confidence bound) Deep Learning (deep learning for multi-target regression, binary and multi-label classification) Deep Learning (learn image classification with convolutional neural networks) Deep Learning (learn about Semantic Segmentation) Deep Learning (Recurrent Neural Networks, LSTMs) More on Deep Learning, e.g. Autoencoders, pretrained models, … R/Shiny for web application development and deployment This course is ideal for individuals who are R beginners interested in learning R or data science practitioners who want to deepen their knowledge or developers who want to learn different aspects of Machine Learning It is particularly useful for R beginners interested in learning R or data science practitioners who want to deepen their knowledge or developers who want to learn different aspects of Machine Learning.
Enroll now: R Ultimate 2024: R for Data Science and Machine Learning
Summary
Title: R Ultimate 2024: R for Data Science and Machine Learning
Price: $79.99
Average Rating: 4.83
Number of Lectures: 204
Number of Published Lectures: 204
Number of Curriculum Items: 204
Number of Published Curriculum Objects: 204
Original Price: €69.99
Quality Status: approved
Status: Live
What You Will Learn
- learn all aspects of R from Basics, over Data Science, to Machine Learning and Deep Learning
- learn R basics (data types, structures, variables, and more)
- learn R programming (writing loops, functions, and more)
- data im- and export
- basic data manipulation (piping, filtering, aggregation of results, data reshaping, set operations, joining datasets)
- data visualisation (different packages are learned, e.g. ggplot, plotly, leaflet, dygraphs)
- advanced data manipulation (outlier detection, missing data handling, regular expressions)
- regression models (create and apply regression models)
- model evaluation (What is underfitting and overfitting? Why is data splitted into training and testing? What are resampling techniques?)
- regularization (What is regularization? How can you apply it?)
- classification models (understand different algorithms and learn how to apply logistic regression, decision trees, random forests, support vector machines)
- association rules (learn the apriori model)
- clustering (kmeans, hierarchical clustering, DBscan)
- dimensionality reduction (factor analysis, principal component analysis)
- Reinforcement Learning (upper confidence bound)
- Deep Learning (deep learning for multi-target regression, binary and multi-label classification)
- Deep Learning (learn image classification with convolutional neural networks)
- Deep Learning (learn about Semantic Segmentation)
- Deep Learning (Recurrent Neural Networks, LSTMs)
- More on Deep Learning, e.g. Autoencoders, pretrained models, …
- R/Shiny for web application development and deployment
Who Should Attend
- R beginners interested in learning R
- data science practitioners who want to deepen their knowledge
- developers who want to learn different aspects of Machine Learning
Target Audiences
- R beginners interested in learning R
- data science practitioners who want to deepen their knowledge
- developers who want to learn different aspects of Machine Learning
You want to be able to perform your own data analyses with R? You want to learn how to get business-critical insights out of your data? Or you want to get a job in this amazing field? In all of these cases, you found the right course!
We will start with the very Basics of R, like data types and -structures, programming of loops and functions, data im- and export.
Then we will dive deeper into data analysis: we will learn how to manipulate data by filtering, aggregating results, reshaping data, set operations, and joining datasets. We will discover different visualisation techniques for presenting complex data. Furthermore find out to present interactive timeseries data, or interactive geospatial data.
Advanced data manipulation techniques are covered, e.g. outlier detection, missing data handling, and regular expressions.
We will cover all fieldsof Machine Learning: Regressionand Classificationtechniques, Clustering, Association Rules, Reinforcement Learning, and, possibly most importantly, Deep Learning for Regression, Classification, Convolutional Neural Networks, Autoencoders, Recurrent Neural Networks, …
You will also learn to develop web applications and how to deploy them with R/Shiny.
For each field, different algorithms are shown in detail: their core concepts are presented in 101 sessions. Here, you will understand how the algorithm works. Then we implement it together in lab sessions. We develop code, before I encourage you to work on exercise on your own, before you watch my solution examples. With this knowledge you can clearly identify a problem at hand and develop a plan of attack to solve it.
You will understand the advantages and disadvantages of different models and when to use which one. Furthermore, you will know how to take your knowledge into the real world.
You will get access to an interactive learning platformthat will help you to understand the concepts much better.
In this course code will never come out of thin air via copy/paste. We will developevery important line of code togetherand I will tell you why and how we implement it.
Take a look at some sample lectures. Or visit some of my interactive learning boards. Furthermore, there is a 30 day money back warranty, so there is no risk for you taking the course right now. Don’t wait. See you in the course.
Course Curriculum
Chapter 1: Course Introduction
Lecture 1: Course Overview
Lecture 2: R and RStudio (Overview and Installation)
Lecture 3: How to get the code
Lecture 4: How to get the code (alternative)
Lecture 5: RStudio Introduction / Project Setup
Lecture 6: File Formats
Lecture 7: Rmarkdown Lab
Lecture 8: Package Handling
Chapter 2: Data Types and -structures
Lecture 1: Basic Data Types 101
Lecture 2: Basic Data Types Lab
Lecture 3: Matrices and Arrays Lab
Lecture 4: Lists
Lecture 5: Factors
Lecture 6: Dataframes
Lecture 7: Strings Lab
Lecture 8: Datetime
Chapter 3: R Programming
Lecture 1: Operators
Lecture 2: Loops 101
Lecture 3: Loops Lab
Lecture 4: Functions 101
Lecture 5: Functions Lab (Intro)
Lecture 6: Functions Lab (Coding)
Chapter 4: Data Im- and Export
Lecture 1: Data Import Lab
Lecture 2: Data Export Lab
Lecture 3: Web Scraping Intro
Lecture 4: Web Scraping Lab
Chapter 5: Basic Data Manipulation
Lecture 1: Piping 101
Lecture 2: Filtering 101
Lecture 3: Filtering Lab
Lecture 4: Filtering Exercise
Lecture 5: Filtering Solution
Lecture 6: Data Aggregation 101
Lecture 7: Data Aggregation Lab
Lecture 8: Data Aggregation Exercise
Lecture 9: Data Aggregation Solution
Lecture 10: Data Reshaping 101
Lecture 11: Data Reshaping Lab
Lecture 12: Data Reshaping Exercise
Lecture 13: Data Reshaping Solution
Lecture 14: Set Operations 101
Lecture 15: Set Operations Lab
Lecture 16: Joining Datasets 101
Lecture 17: Joining Datasets Lab
Chapter 6: Data Visualisation
Lecture 1: Visualisation Overview
Lecture 2: ggplot 101
Lecture 3: ggplot Lab
Lecture 4: plotly Lab (Intro)
Lecture 5: plotly Lab
Lecture 6: leaflet Lab (Intro)
Lecture 7: leaflet Lab
Lecture 8: dygraphs Lab (Intro)
Lecture 9: dygraphs Lab
Chapter 7: Advanced Data Manipulation
Lecture 1: Outlier Detection 101
Lecture 2: Outlier Detection Lab (Intro)
Lecture 3: Outlier Detection Lab
Lecture 4: Outlier Detection Exercise
Lecture 5: Outlier Detection Solution
Lecture 6: Missing Data Handling 101
Lecture 7: Missing Data Handling Lab (Intro)
Lecture 8: Missing Data Handling Lab (1/1)
Lecture 9: Regular Expressions 101
Lecture 10: Regular Expressions Lab
Chapter 8: Machine Learning: Introduction
Lecture 1: AI 101
Lecture 2: Machine Learning 101
Lecture 3: Models
Chapter 9: Machine Learning: Regression
Lecture 1: Regression Types 101
Lecture 2: Univariate Regression 101
Lecture 3: Univariate Regression Interactive
Lecture 4: Univariate Regression Lab
Lecture 5: Univariate Regression Exercise
Lecture 6: Univariate Regression Solution
Lecture 7: Polynomial Regression 101
Lecture 8: Polynomial Regression Lab
Lecture 9: Multivariate Regression 101
Lecture 10: Multivariate Regression Lab
Lecture 11: Multivariate Regression Exercise
Lecture 12: Multivariate Regression Solution
Chapter 10: Machine Learning: Model Preparation and Evaluation
Lecture 1: Underfitting / Overfitting 101
Lecture 2: Train / Validation / Test Split 101
Lecture 3: Train / Validation / Test Split Interactive
Lecture 4: Train / Validation / Test Split Lab
Lecture 5: Resampling Techniques 101
Lecture 6: Resampling Techniques Lab
Chapter 11: Machine Learning: Regularization
Lecture 1: Regularization 101
Lecture 2: Regularization Lab
Chapter 12: Machine Learning: Classification Basics
Lecture 1: Confusion Matrix 101
Lecture 2: ROC Curve 101
Lecture 3: ROC Curve Interactive
Instructors
-
Bert Gollnick
Data Scientist
Rating Distribution
- 1 stars: 0 votes
- 2 stars: 4 votes
- 3 stars: 8 votes
- 4 stars: 55 votes
- 5 stars: 126 votes
Frequently Asked Questions
How long do I have access to the course materials?
You can view and review the lecture materials indefinitely, like an on-demand channel.
Can I take my courses with me wherever I go?
Definitely! If you have an internet connection, courses on Udemy are available on any device at any time. If you don’t have an internet connection, some instructors also let their students download course lectures. That’s up to the instructor though, so make sure you get on their good side!
You may also like
- Best Video Editing Courses to Learn in March 2025
- Best Music Production Courses to Learn in March 2025
- Best Animation Courses to Learn in March 2025
- Best Digital Illustration Courses to Learn in March 2025
- Best Renewable Energy Courses to Learn in March 2025
- Best Sustainable Living Courses to Learn in March 2025
- Best Ethical AI Courses to Learn in March 2025
- Best Cybersecurity Fundamentals Courses to Learn in March 2025
- Best Smart Home Technology Courses to Learn in March 2025
- Best Holistic Health Courses to Learn in March 2025
- Best Nutrition And Diet Planning Courses to Learn in March 2025
- Best Yoga Instruction Courses to Learn in March 2025
- Best Stress Management Courses to Learn in March 2025
- Best Mindfulness Meditation Courses to Learn in March 2025
- Best Life Coaching Courses to Learn in March 2025
- Best Career Development Courses to Learn in March 2025
- Best Relationship Building Courses to Learn in March 2025
- Best Parenting Skills Courses to Learn in March 2025
- Best Home Improvement Courses to Learn in March 2025
- Best Gardening Courses to Learn in March 2025