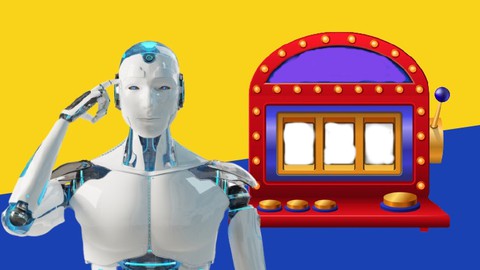
Practical Multi-Armed Bandit Algorithms in Python
Practical Multi-Armed Bandit Algorithms in Python, available at $69.99, has an average rating of 4.7, with 23 lectures, 3 quizzes, based on 140 reviews, and has 811 subscribers.
You will learn about Understanding and being able to identify Multi-Armed Bandit problems. Modelling real business problems as MAB and implementing digital AI agents to automate them. Understanding the challenge of RL regarding the exploration-exploitation dilema. Practical implementation of the various algorithmic strategies for balancing between exploration and exploitation. Python implementation of the Epsilon-greedy strategy. Python implementation of the Softmax Exploration strategy. Python implementation of the Optimistic Initialization strategy. Python implementation of the Upper Confidence Bounds (UCB) strategy. Understand the challenges of RL in terms of the design of reward functions and sample efficiency. Estimation of action values through incremental sampling. This course is ideal for individuals who are Anyone with a basic Python skills desiring to the started in Reinforcement Learning. or Experienced AI Engineers, ML Engineers, Data Scientist, and Software Engineers wanting to apply Reinforcement Learning to real business problems. or Business professionals willing to learn how Reinforcement Learning can help with automating adaptive decision making processes. It is particularly useful for Anyone with a basic Python skills desiring to the started in Reinforcement Learning. or Experienced AI Engineers, ML Engineers, Data Scientist, and Software Engineers wanting to apply Reinforcement Learning to real business problems. or Business professionals willing to learn how Reinforcement Learning can help with automating adaptive decision making processes.
Enroll now: Practical Multi-Armed Bandit Algorithms in Python
Summary
Title: Practical Multi-Armed Bandit Algorithms in Python
Price: $69.99
Average Rating: 4.7
Number of Lectures: 23
Number of Quizzes: 3
Number of Published Lectures: 23
Number of Published Quizzes: 3
Number of Curriculum Items: 26
Number of Published Curriculum Objects: 26
Original Price: $19.99
Quality Status: approved
Status: Live
What You Will Learn
- Understanding and being able to identify Multi-Armed Bandit problems.
- Modelling real business problems as MAB and implementing digital AI agents to automate them.
- Understanding the challenge of RL regarding the exploration-exploitation dilema.
- Practical implementation of the various algorithmic strategies for balancing between exploration and exploitation.
- Python implementation of the Epsilon-greedy strategy.
- Python implementation of the Softmax Exploration strategy.
- Python implementation of the Optimistic Initialization strategy.
- Python implementation of the Upper Confidence Bounds (UCB) strategy.
- Understand the challenges of RL in terms of the design of reward functions and sample efficiency.
- Estimation of action values through incremental sampling.
Who Should Attend
- Anyone with a basic Python skills desiring to the started in Reinforcement Learning.
- Experienced AI Engineers, ML Engineers, Data Scientist, and Software Engineers wanting to apply Reinforcement Learning to real business problems.
- Business professionals willing to learn how Reinforcement Learning can help with automating adaptive decision making processes.
Target Audiences
- Anyone with a basic Python skills desiring to the started in Reinforcement Learning.
- Experienced AI Engineers, ML Engineers, Data Scientist, and Software Engineers wanting to apply Reinforcement Learning to real business problems.
- Business professionals willing to learn how Reinforcement Learning can help with automating adaptive decision making processes.
This course is your perfect entry point into the exciting field of Reinforcement Learning where digital Artificial Intelligence agents are built to automatically learn how to make sequential decisions through trial-and-error. Specifically, this course focuses on the Multi-Armed Bandit problems and the practical hands-on implementation of various algorithmic strategies for balancing between exploration and exploitation. Whenever you desire to consistently make the best choice out of a limited number of options over time, you are dealing with a Multi-Armed Bandit problem and this course teaches you every detail you need to know to be able to build realistic business agents to handle such situations.
With very concise explanations, this course teaches you how to confidently translate seemingly scary mathematical formulas into Python code painlessly. We understand that not many of us are technically adept in the subject of mathematics so this course intentionally stays away from maths unless it is necessary. And even when it becomes necessary to talk about mathematics, the approach taken in this course is such that anyone with basic algebra skills can understand and most importantly easily translate the maths into code and build useful intuitions in the process.
Some of the algorithmic strategies taught in this course are Epsilon Greedy, Softmax Exploration, Optimistic Initialization, Upper Confidence Bounds, and Thompson Sampling. With these tools under your belt, you are adequately equipped to readily build and deploy AI agents that can handle critical business operations under uncertainties.
To bridge the gap between theory and application, I’ve updated this course to include a section where I show how to apply the MAB algorithms in Robotics using the EV3 Mindstorm. I’ll soon upload a section that will show how to apply the algorithms taught in this course to optimize advertisements.
Course Curriculum
Chapter 1: Course Lectures
Lecture 1: Introduction to Reinforcement Learning & Multi-Armed Bandit Problems
Lecture 2: Implementing Simulated MAB Environments in Python
Lecture 3: Estimating Action Values Through Sampling
Lecture 4: Implementing Incremental Average In Code
Lecture 5: Implementing Incremental Average For Non-Stationary Bandits
Lecture 6: Building A Baseline Agent That Behaves Randomly
Lecture 7: Why Are The Results Not Repeatable?
Lecture 8: Implementing And Analysing A Greedy Agent
Lecture 9: Balancing Exploration & Exploitation With Epsilon Greedy Agents
Lecture 10: Controlling Exploration With A Decay
Lecture 11: Exploring Intelligently With Softmax Exploration
Lecture 12: Being Optimistic Under Uncertainties
Lecture 13: Realistic Optimism Under Uncertainties
Chapter 2: Applying MAB In Robotics
Lecture 1: Introduction
Lecture 2: What is Reinforcement Learning
Lecture 3: The Reinforcement Learning Workflow
Lecture 4: Reinforcement Learning In A Robotics Context
Lecture 5: The Multi-Armed Bandit Algorithm
Lecture 6: More About The Multi-Armed Bandit Algorithm
Lecture 7: Balancing Exploration & Exploitation
Lecture 8: Using The Multi-Armed Bandit Algorithm In Robotics
Lecture 9: Implementing The Multi-Armed Bandit Algorithm on EV3 Mindstorm
Lecture 10: Demo & Explanation Of The Behaviour Of The Robot
Instructors
-
Edward Pie
Snr. AI Engineer
Rating Distribution
- 1 stars: 0 votes
- 2 stars: 5 votes
- 3 stars: 9 votes
- 4 stars: 51 votes
- 5 stars: 75 votes
Frequently Asked Questions
How long do I have access to the course materials?
You can view and review the lecture materials indefinitely, like an on-demand channel.
Can I take my courses with me wherever I go?
Definitely! If you have an internet connection, courses on Udemy are available on any device at any time. If you don’t have an internet connection, some instructors also let their students download course lectures. That’s up to the instructor though, so make sure you get on their good side!
You may also like
- Best Augmented Reality Courses to Learn in April 2025
- Best Blockchain Development Courses to Learn in April 2025
- Best Unity Game Development Courses to Learn in April 2025
- Best Artificial Intelligence Courses to Learn in April 2025
- Best Flutter Development Courses to Learn in April 2025
- Best Docker Kubernetes Courses to Learn in April 2025
- Best Business Analytics Courses to Learn in April 2025
- Best Excel Vba Courses to Learn in April 2025
- Best Devops Courses to Learn in April 2025
- Best Angular Courses to Learn in April 2025
- Best Node Js Development Courses to Learn in April 2025
- Best React Js Courses to Learn in April 2025
- Best Cyber Security Courses to Learn in April 2025
- Best Machine Learning Courses to Learn in April 2025
- Best Ethical Hacking Courses to Learn in April 2025
- Best Ui Ux Design Courses to Learn in April 2025
- Best Aws Certification Courses to Learn in April 2025
- Best Data Science Courses to Learn in April 2025
- Best Digital Marketing Courses to Learn in April 2025
- Best Web Development Courses to Learn in April 2025